Maritime Object Detection with Event-Based Cameras
2022 IEEE Applied Imagery Pattern Recognition Workshop (AIPR)(2022)
摘要
Event cameras differ from conventional frame-based cameras in that each individual pixel responds independently and asynchronously to brightness changes. Instead of absolute brightness measurements reported as entire frames at regular time intervals, data from event cameras come as a stream of spatially and temporally sparse brightness change events. Event cameras have several characteristics that are favorable to maritime computer vision tasks, including high dynamic range and high temporal resolution. In this work, we apply Asynet, a sparse convolutional neural network based object detection model, to maritime event data sets we collected in the field. To address the limited size of our data set, we propose fine-tuning from pretrained weights learned from the neuromorphic Caltech101 (N-Caltech101) data set and then using a combination of augmentation techniques drawn from traditional image-based computer vision as well as event-specific augmentations. Empirical findings show that using simple image-based augmentation strategies are enough to significantly boost the performance of the Asynet model.
更多查看译文
AI 理解论文
溯源树
样例
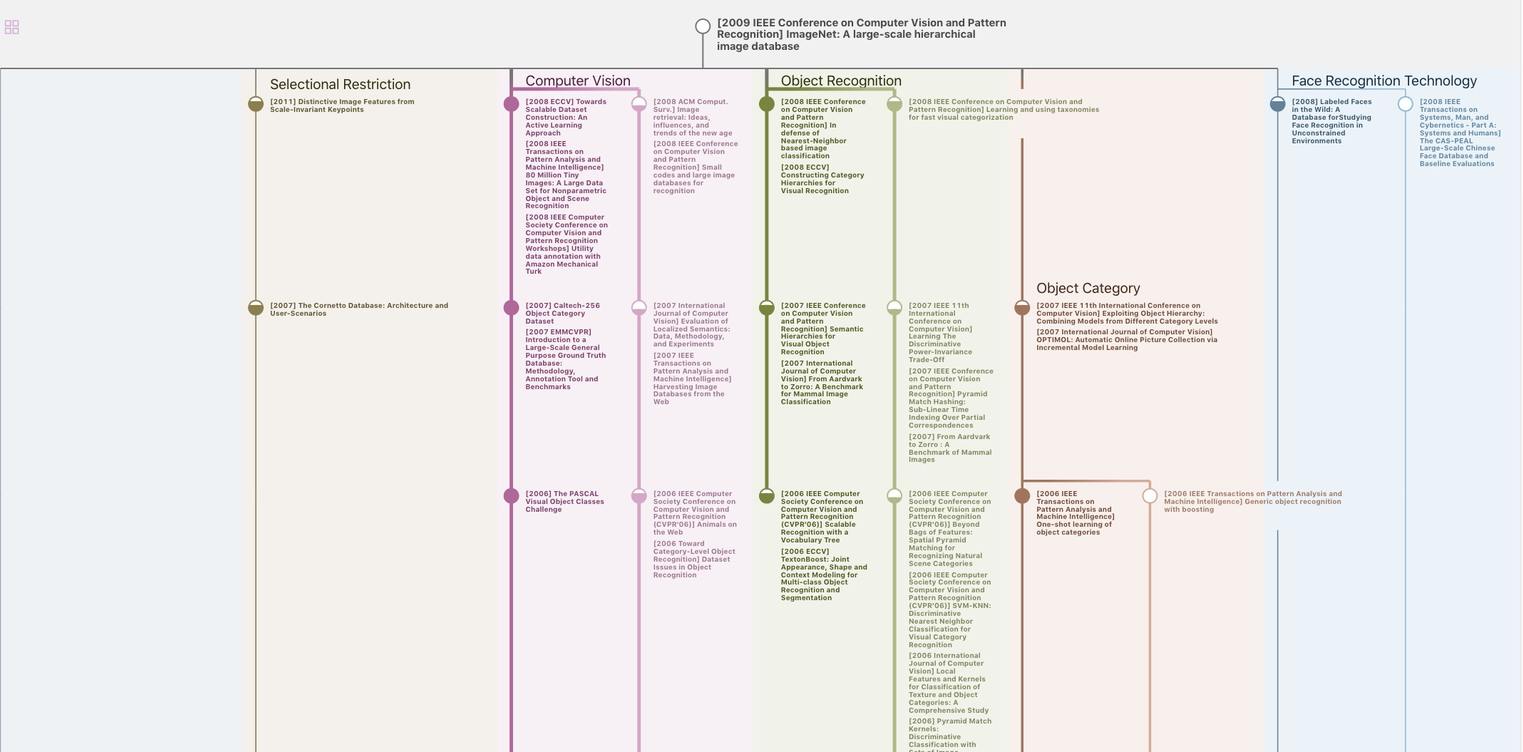
生成溯源树,研究论文发展脉络
Chat Paper
正在生成论文摘要