An Evaluation of Federated Learning Techniques for Secure and Privacy-Preserving Machine Learning on Medical Datasets
2022 IEEE Applied Imagery Pattern Recognition Workshop (AIPR)(2022)
摘要
Federated learning is a machine learning technique that trains a machine learning model across multiple decentralized edge devices or servers holding local data samples without revealing them. It is a technique that ensures the privacy of data stored (locally on a server/edge device) while training a machine learning model. This technique is particularly valuable for hospitals to collaboratively learn machine learning models without moving all the training data (e.g., patient records) to a centralized location due to federal laws. In this experimental study, we investigate how federated learning performs on different real-world medical datasets using a cluster of machines. We tested different deep learning models and federated optimization strategies on CloudLab, which is an experimental testbed for networking and distributed computing research. Our experiments showed that Inception-v3 and EfficientNetB0 were usually the top two performing models based on accuracy computed over the test set. For federated optimizations, FedAvg was the best strategy followed by FedAvgM.
更多查看译文
关键词
Federated learning,medical image datasets,secure,privacy-preserving,machine learning
AI 理解论文
溯源树
样例
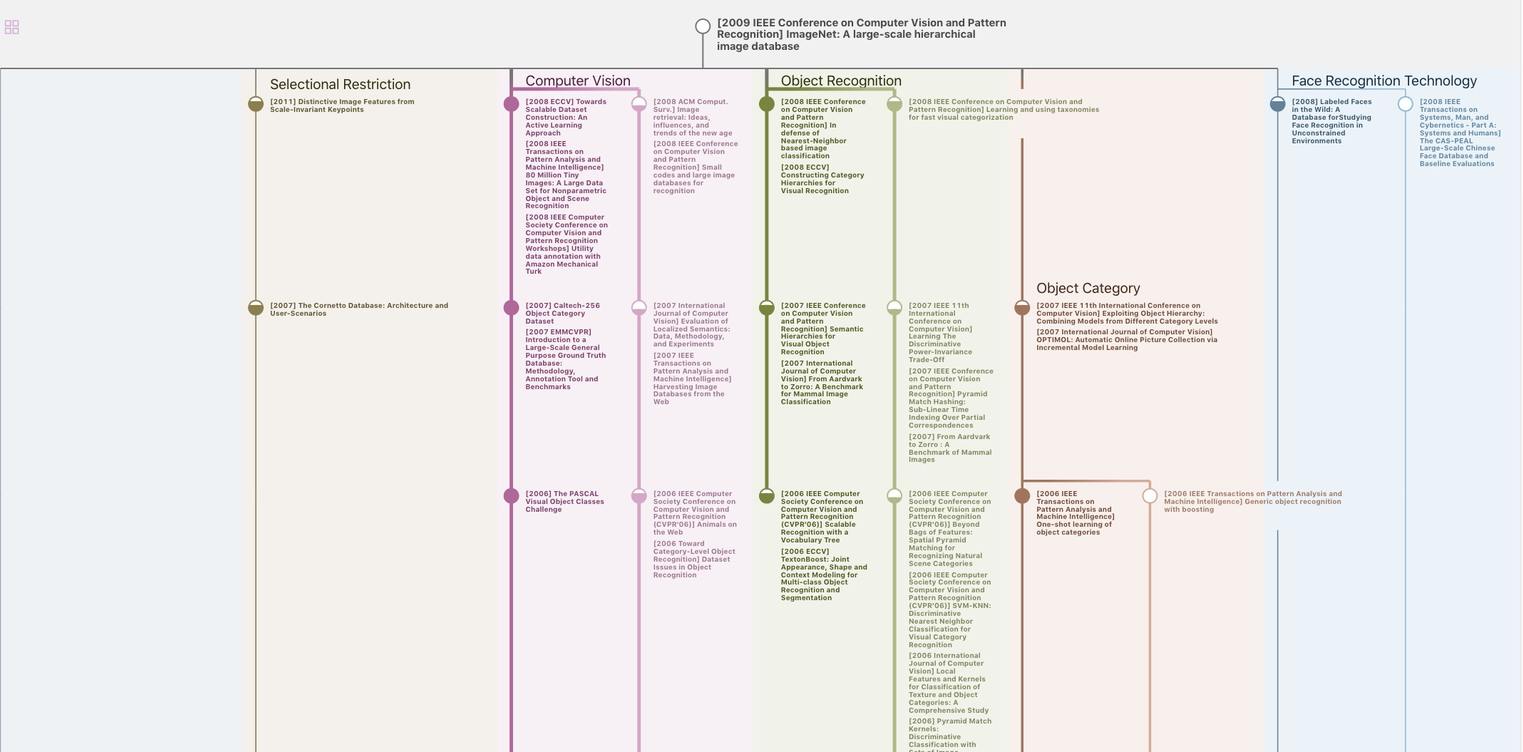
生成溯源树,研究论文发展脉络
Chat Paper
正在生成论文摘要