Deep-Learning Hopping Capture Model for Automatic Modulation Classification of Wireless Communication Signals
IEEE Transactions on Aerospace and Electronic Systems(2023)
摘要
Recent years have witnessed a surge of developments in deep learning (DL) motivated by a variety of contemporary applications. The conventional DL-based automatic modulation classification (AMC) methods are always relying on a great quantity of data. In this article, we propose a DL-based AMC model with short data for the spectrum sensing of wireless communication signals. First, a hopping transform unit is proposed to represent the transient variation occurred either by frequency, amplitude, or phase modulations. Second, a bidirectional long short-term memory-based hopping feature perception model, namely deep-learning hopping capture model (DHCM), is built for the AMC. A comprehensive comparison of the DHCM with other existing methods is then provided under various signal-to-noise ratios. The experimental results demonstrate the superiority of the proposed method under short data.
更多查看译文
关键词
Time-frequency analysis,Feature extraction,Modulation,Phase shift keying,Frequency shift keying,Constellation diagram,Long short term memory,Automatic modulation classification (AMC),deep learning (DL),short data,spectrum sensing
AI 理解论文
溯源树
样例
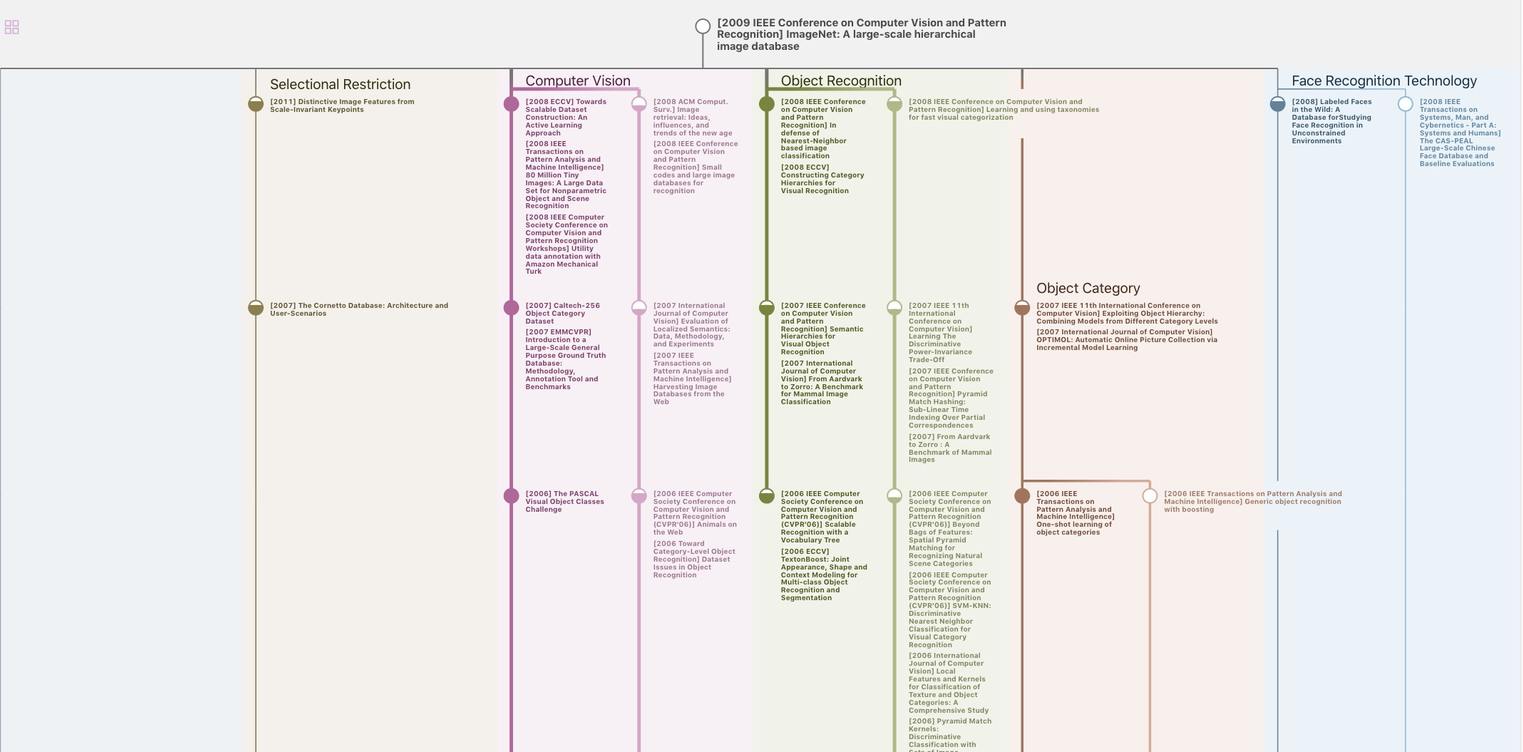
生成溯源树,研究论文发展脉络
Chat Paper
正在生成论文摘要