Multi-agent and Reinforcement Learning Schemes for Demand Response Estimation in Distributed Microgrids
2023 6th International Conference on Energy Conservation and Efficiency (ICECE)(2023)
摘要
Demand response (DR) is an approach that encourages consumers to shape consumption patterns in peak demands for the reliability of the power system and cost minimization. The optimal DR scheme has not only leverages the distribution system operators (DSOs) but also the consumers in the energy network. This paper introduced a multi-agent coordination control and reinforcement learning approach for optimal DR management. Each microgrid is considered an agent for the state and action estimation in the smart grid and programmed rewards and incentive plans. In this regard, the Multi-agent Markov game (MAMG) is utilized for the state and action. At the same time, the reward is articulated through reinforcement learning deep Q-network (DQN) and deep deterministic policy gradient (DDPG) schemes. The proposed DR model also encourages consumer participation for long-term incentivized benefits through integrating battery energy storage systems (BESS) in the SG network. The reliability of DQN and DDPG schemes is demonstrated and observed that the dynamically changing electricity cost is reduced by 19.86%. Moreover, the controllability of complex microgrids is achieved with limited control information to ensure the integrity and reliability of the network. The proposed schemes were simulated and evaluated in MATLAB and Python (PyCharm IDE) environments.
更多查看译文
关键词
Demand response,Multi-agent control,Deep Q-network,Reinforcement learning,Deep deterministic policy gradient,Networked microgrids,Distributed Smart grid
AI 理解论文
溯源树
样例
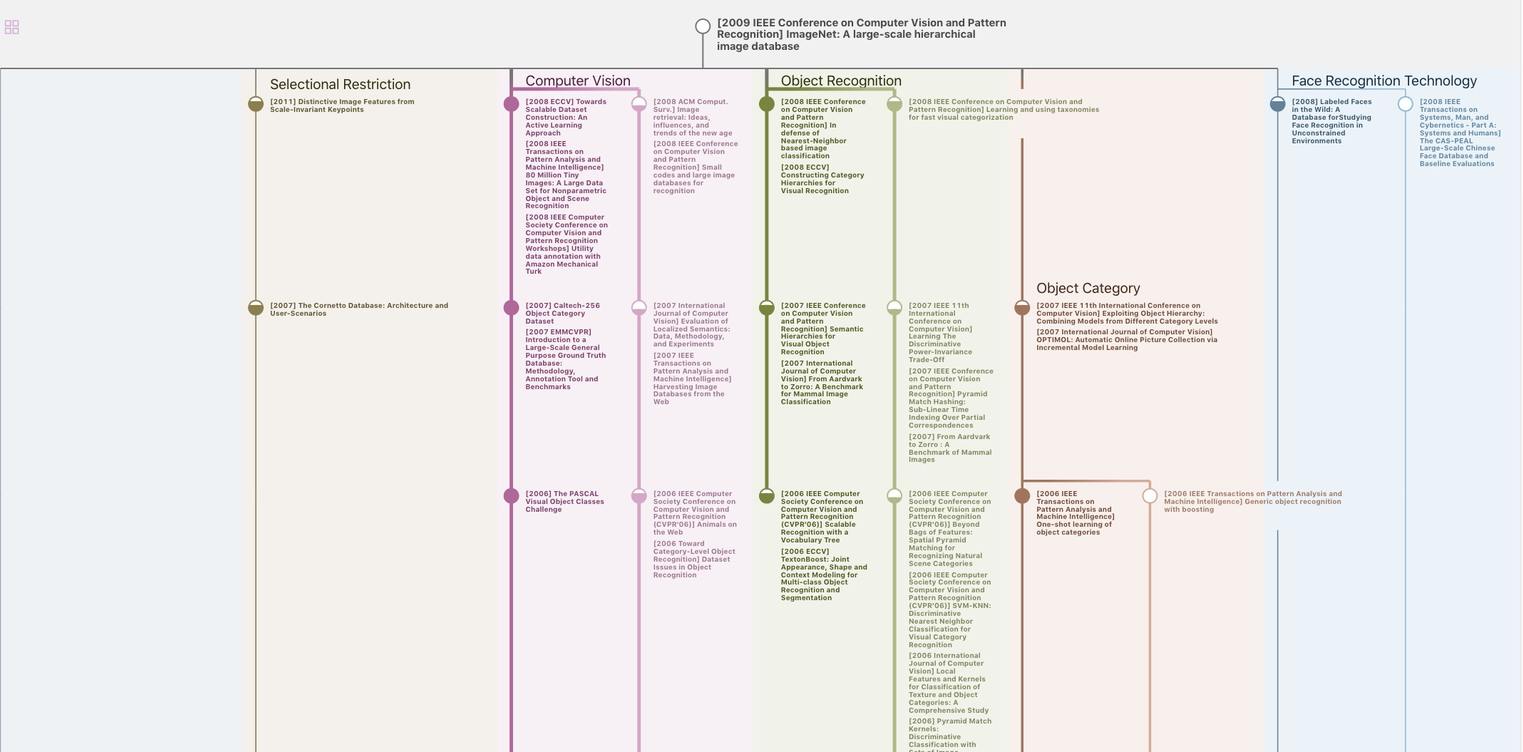
生成溯源树,研究论文发展脉络
Chat Paper
正在生成论文摘要