Modelling the Long-Term Effects of Covid-19 Cancer Services Disruption on Patient Outcome in Scotland
medRxiv (Cold Spring Harbor Laboratory)(2021)
摘要
Background Modelling the long-term effects of disruption of cancer services and minimising any excess cancer mortality due to the Covid-19 pandemic is of great importance. Here we adapted a stage-shift model to inform service planning decisions within NHS Scotland for the ‘‘Detect Cancer Early’ tumours, breast, colorectal and lung cancer which represent 46% of all cancers diagnosed in Scotland.
Methods & Data Lung, colorectal and breast cancer incidence data for years 2017-18 were obtained from Public Health Scotland Cancer Quality Performance Indicators (QPI), to define a baseline scenario. The most current stage-specific 5-year survival data came from 2009-2014 national cancer registry and South East Scotland Cancer Network (SCAN) QPI audit datasets. The Degeling et al., inverse stage-shift model was adapted to estimate changes in stage at diagnosis, excess mortality and life-years lost from delays to diagnosis and treatment due to Covid-19-related health services disruption. Three and 6-month periods of disruption were simulated to demonstrate the model predictions.
Results Approximately, 1-9% reductions in stage I/II presentations leading up to 2-10% increases in stage III/IV presentations are estimated across the three cancer types. A 6-month period of service disruption is predicted to lead to excess deaths at 5 years of 32.5 (31.1, 33.9) per 1000 cases for lung cancer, 16.5 (7.9, 24.3) for colorectal cancer and 31.6 (28.5, 34.4) for breast cancer.
Conclusions Disruption of cancer diagnostic services can lead to significant excess deaths in following years. Increasing diagnostic and capacity for cancer services to deal with the backlog of care are needed. Real time monitoring of incidence and referral patterns over the disruption and post-disruption period to reduce excess deaths including more rapid incidence data by stage and other key tumour/clinical characteristics at presentation for key cancer cases (on a quarterly basis). Real time monitoring in cancer care and referral patterns should help inform what type of interventions are needed to reduce excess mortality and whether different population subgroups require public health messaging campaigns. Specific mitigation measures can be the subject of additional modelling analysis to assess the benefits and inform service planning decision making.
### Competing Interest Statement
The authors have declared no competing interest.
### Funding Statement
This study was funded by the University of Edinburgh Data-Driven Innovation funds 19 projects to fight COVID-19
### Author Declarations
I confirm all relevant ethical guidelines have been followed, and any necessary IRB and/or ethics committee approvals have been obtained.
Yes
The details of the IRB/oversight body that provided approval or exemption for the research described are given below:
These data are from a modeling study using publicly/published available data.
All necessary patient/participant consent has been obtained and the appropriate institutional forms have been archived.
Yes
I understand that all clinical trials and any other prospective interventional studies must be registered with an ICMJE-approved registry, such as ClinicalTrials.gov. I confirm that any such study reported in the manuscript has been registered and the trial registration ID is provided (note: if posting a prospective study registered retrospectively, please provide a statement in the trial ID field explaining why the study was not registered in advance).
Yes
I have followed all appropriate research reporting guidelines and uploaded the relevant EQUATOR Network research reporting checklist(s) and other pertinent material as supplementary files, if applicable.
Yes
Model and input paramaters are available on github
[https://github.com/EwanGray/cancer\_covid\_scot][1]
[1]: https://github.com/EwanGray/cancer_covid_scot
更多查看译文
关键词
patient outcome,cancer,disruption,long-term
AI 理解论文
溯源树
样例
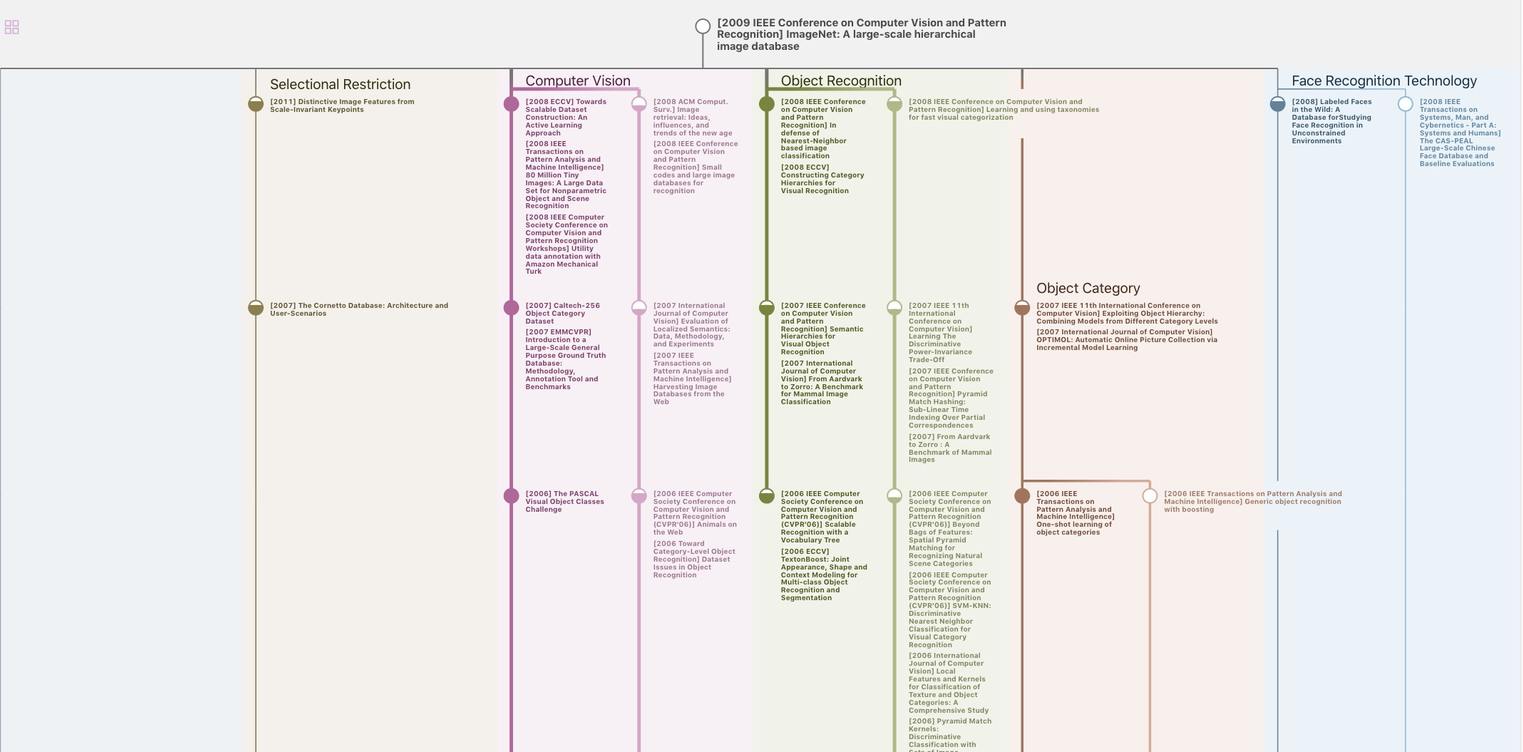
生成溯源树,研究论文发展脉络
Chat Paper
正在生成论文摘要