MS-HNN: Multi-scale Hierarchical Neural Network with Squeeze and Excitation Block for Neonatal Sleep Staging Using a Single-Channel EEG.
IEEE transactions on neural systems and rehabilitation engineering : a publication of the IEEE Engineering in Medicine and Biology Society(2023)
摘要
Most existing neonatal sleep staging approaches applied multiple EEG channels to obtain good performance. However, it potentially increased the computational complexity and led to an increased risk of skin disruption to neonates during data acquisition. In this paper, a multi-scale hierarchical neural network (MS-HNN) with a squeeze and excitation (SE) block for neonatal sleep staging is presented in this study on the basis of a single EEG channel. MS-HNN composes of multi-scale convolutional neural network (MSCNN), temporal information learning (TIL) module, and squeeze and excitation (SE) block. MSCNN can extract features from different scales and frequencies, and TIL module can acquire the transition information among adjacent stages. In addition, for these extracted features, SE block can selectively concentrate on informative features and weaken redundant features for achieving better performance. The proposed approach was validated on a clinical dataset involving 64 neonates from the Children's Hospital of Fudan University (CHFU). The proposed network achieves an accuracy of 75.4% and 76.5% for three-class automatic neonatal sleep staging with the single-EEG channel and the eight-EEG channels, respectively. The experimental results show that the proposed method can maintain good performance by making full use of the information in the single channel while reducing the channels to control the computational overhead.
更多查看译文
关键词
neonatal sleep staging,eeg,ms-hnn,multi-scale,single-channel
AI 理解论文
溯源树
样例
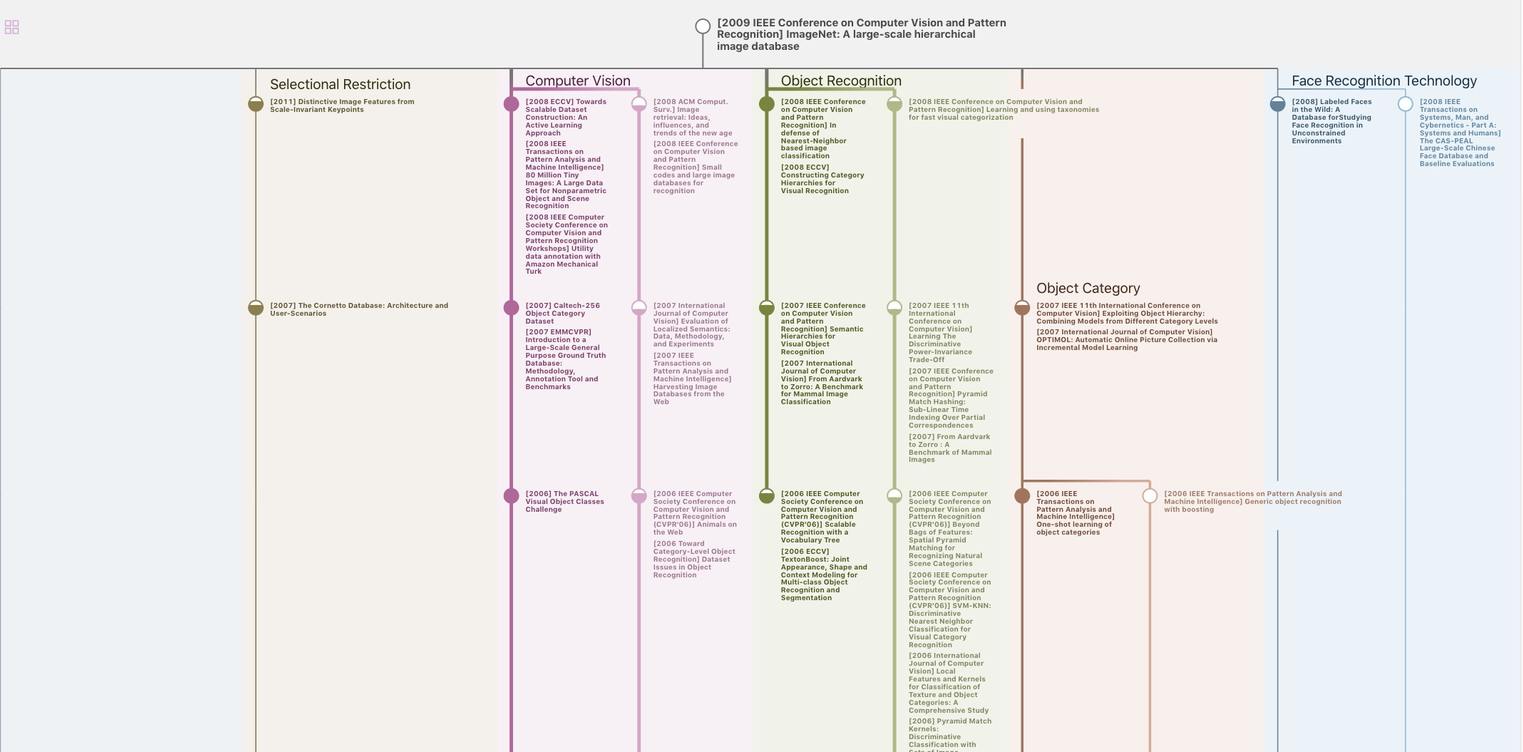
生成溯源树,研究论文发展脉络
Chat Paper
正在生成论文摘要