Medical image segmentation based on self-supervised hybrid fusion network
Frontiers in Oncology(2023)
摘要
Automatic segmentation of medical images has been a hot research topic in the field of deep learning in recent years, and achieving accurate segmentation of medical images is conducive to breakthroughs in disease diagnosis, monitoring, and treatment. In medicine, MRI imaging technology is often used to image brain tumors, and further judgment of the tumor area needs to be combined with expert analysis. If the diagnosis can be carried out by computer-aided methods, the efficiency and accuracy will be effectively improved. Therefore, this paper completes the task of brain tumor segmentation by building a self-supervised deep learning network. Specifically, it designs a multi-modal encoder-decoder network based on the extension of the residual network. Aiming at the problem of multi-modal feature extraction, the network introduces a multi-modal hybrid fusion module to fully extract the unique features of each modality and reduce the complexity of the whole framework. In addition, to better learn multi-modal complementary features and improve the robustness of the model, a pretext task to complete the masked area is set, to realize the self-supervised learning of the network. Thus, it can effectively improve the encoder’s ability to extract multi-modal features and enhance the noise immunity. Experimental results present that our method is superior to the compared methods on the tested datasets.
更多查看译文
关键词
self-supervised learning,multi-modal,hybrid fusion,medical image segmentation,medical image segmentation based on self-supervised hybrid fusion network
AI 理解论文
溯源树
样例
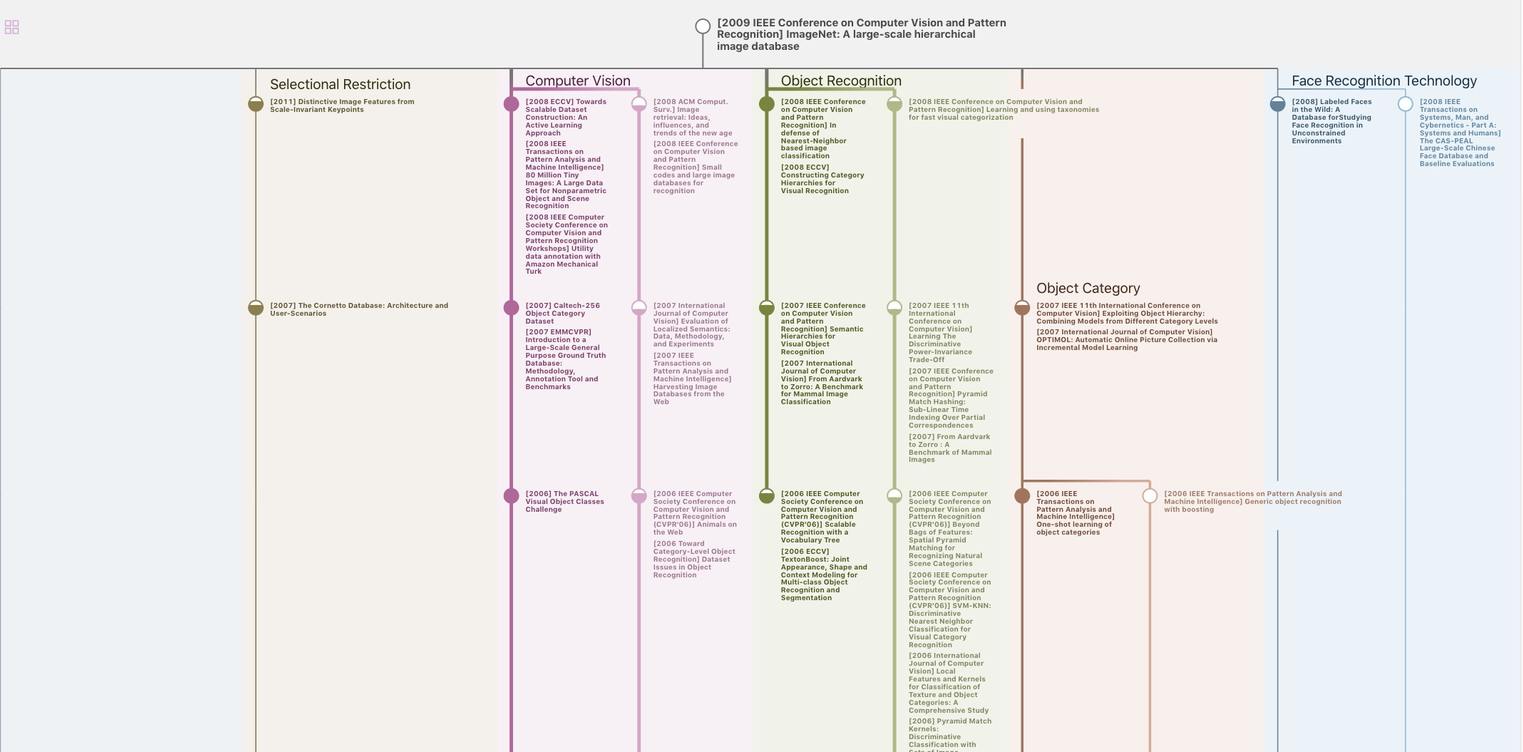
生成溯源树,研究论文发展脉络
Chat Paper
正在生成论文摘要