Advancing Adversarial Training by Injecting Booster Signal.
IEEE transactions on neural networks and learning systems(2023)
摘要
Recent works have demonstrated that deep neural networks (DNNs) are highly vulnerable to adversarial attacks. To defend against adversarial attacks, many defense strategies have been proposed, among which adversarial training (AT) has been demonstrated to be the most effective strategy. However, it has been known that AT sometimes hurts natural accuracy. Then, many works focus on optimizing model parameters to handle the problem. Different from the previous approaches, in this article, we propose a new approach to improve the adversarial robustness using an external signal rather than model parameters. In the proposed method, a well-optimized universal external signal called a booster signal is injected into the outside of the image which does not overlap with the original content. Then, it boosts both adversarial robustness and natural accuracy. The booster signal is optimized in parallel to model parameters step by step collaboratively. Experimental results show that the booster signal can improve both the natural and robust accuracies over the recent state-of-the-art AT methods. Also, optimizing the booster signal is general and flexible enough to be adopted on any existing AT methods.
更多查看译文
关键词
Adversarial defense,adversarial robustness,adversarial training (AT),booster signal
AI 理解论文
溯源树
样例
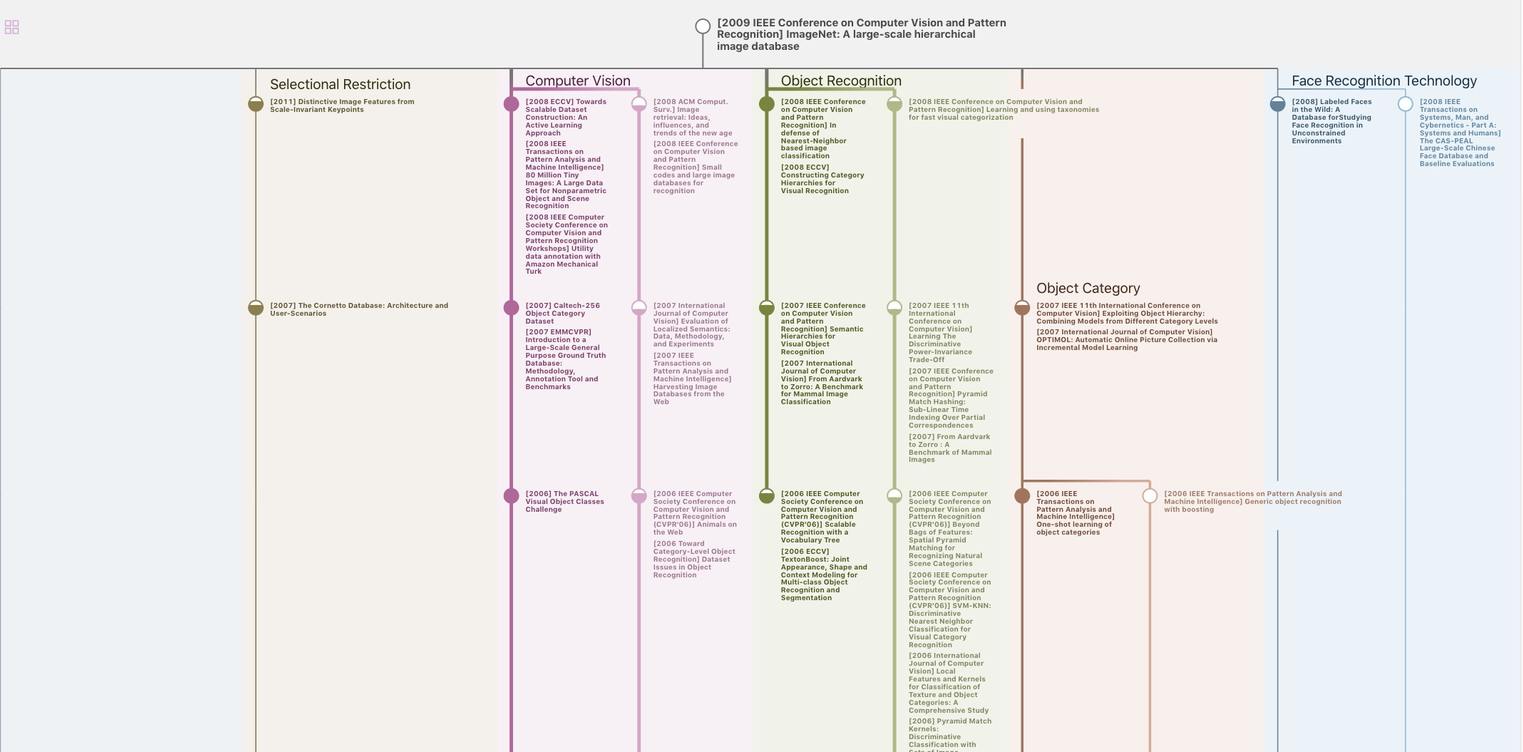
生成溯源树,研究论文发展脉络
Chat Paper
正在生成论文摘要