Re-building a machine learning model for IFI risk prediction
Journal of Critical Care(2023)
摘要
Combat-related invasive fungal infections (IFIs) are associated with high morbidity and mortality. Using data collected from combat-wounded military service members, two Bayesian believe network (BBN) models were previously developed to predict IFI risks. We believed that these models could potentially be improved with an alternate methodology; we then reanalyzed the data and redeveloped a random forest model with 14 features (F14-RF model) selected by machine learning. Evidence from both internal and external model assessments, plus direct comparisons of the model with the two BBNs, indicated that the F14-RF model is superior to the two BBNs, in terms of discrimination, calibration, and decision curve analysis. Furthermore, we propose a machine learning pipeline for the surgical critical care setting, which includes robust methods for feature selection, model training, model assessment, and calibration.
更多查看译文
关键词
prediction,machine learning model,risk,machine learning,learning model,re-building
AI 理解论文
溯源树
样例
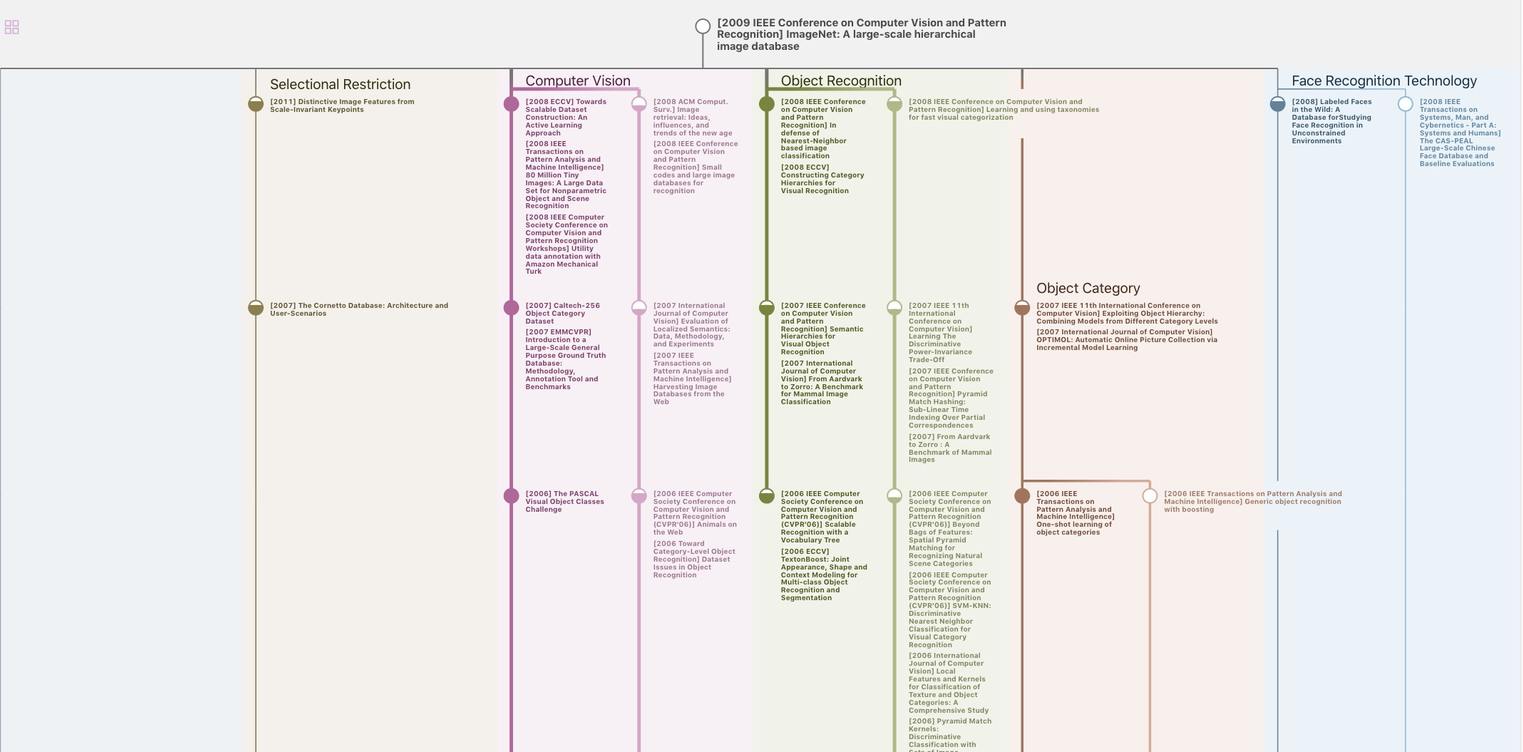
生成溯源树,研究论文发展脉络
Chat Paper
正在生成论文摘要