The Discriminants of Long and Short Duration Failures in Fulfillment Sortation Equipment: A Machine Learning Approach
Journal of Engineering(2023)
摘要
Due to the difficulties inherent in diagnostics and prognostics, maintaining machine health remains a substantial issue in industrial production. Current approaches rely substantially on human engagement, making them costly and unsustainable, especially in high-volume industrial complexes like fulfillment centers. The length of time that fulfillment center equipment failures last is particularly important because it affects operational costs dramatically. A machine learning approach for identifying long and short equipment failures is presented using historical equipment failure and fault data. Under a variety of hyperparameter configurations, we test and compare the outcomes of eight different machine learning classification algorithms, seven individual classifiers, and a stacked ensemble. The gradient boosting classifier (GBC) produces state-of-the-art results in this setting, with precision of 0.76, recall of 0.82, and false positive rate (FPR) of 0.002. This model has since been applied successfully to automate the detection of long- and short-term defects, which has improved equipment maintenance schedules and personnel allocation towards fulfillment operations. Since its launch, this system has contributed to saving over $500 million in fulfillment expenses. It has also resulted in a better understanding of the flaws that cause long-term failures, which is now being used to build more sophisticated failure prediction and risk-mitigation systems for fulfillment equipment.
更多查看译文
关键词
fulfillment sortation equipment,machine learning approach,short duration failures,machine learning
AI 理解论文
溯源树
样例
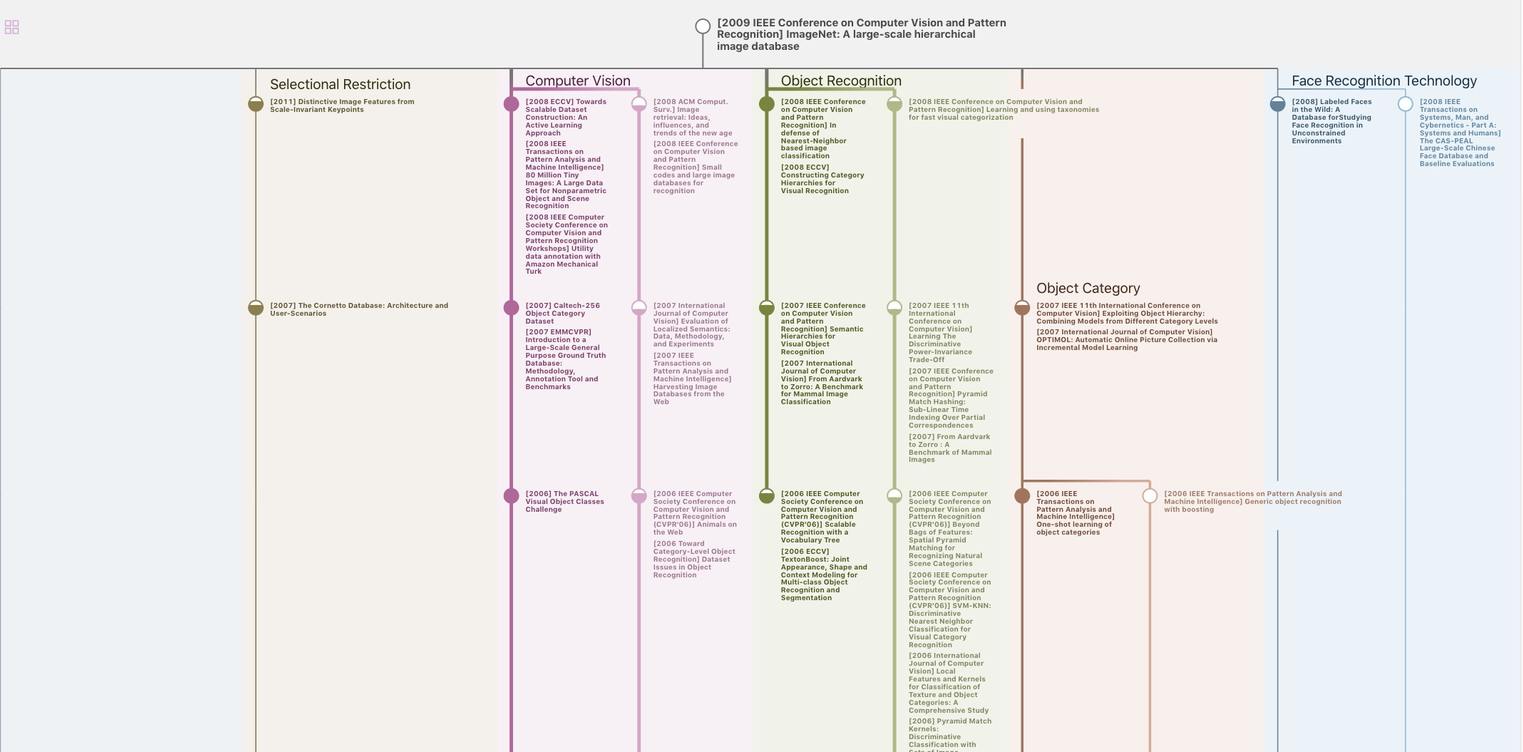
生成溯源树,研究论文发展脉络
Chat Paper
正在生成论文摘要