LOCC: a novel visualization and scoring of cutoffs for continuous variables.
bioRxiv : the preprint server for biology(2023)
摘要
Objective:There is a need for new methods to select and analyze cutoffs employed to define genes that are most prognostic significant and impactful. We designed LOCC (Luo's Optimization Categorization Curve), a novel tool to visualize and score continuous variables for a dichotomous outcome.
Methods:To demonstrate LOCC with real world data, we analyzed TCGA hepatocellular carcinoma gene expression and patient data using LOCC. We compared LOCC visualization to receiver operating characteristic (ROC) curve for prognostic modeling to showcase its utility in understanding predictors in various TCGA datasets.
Results:Analysis of E2F1 expression in hepatocellular carcinoma using LOCC demonstrated appropriate cutoff selection and validation. In addition, we compared LOCC visualization and scoring to ROC curves and c-statistics, demonstrating that LOCC better described predictors. Analysis of a previously published gene signature showed large differences in LOCC scoring, and removing the lowest scoring genes did not affect prognostic modeling of the gene signature demonstrating LOCC scoring could distinguish which predictors were most critical.
Conclusion:Overall, LOCC is a novel visualization tool for understanding and selecting cutoffs, particularly for gene expression analysis in cancer. The LOCC score can be used to rank genes for prognostic potential and is more suitable than ROC curves for prognostic modeling.
更多查看译文
关键词
cutoffs,novel visualization,continuous
AI 理解论文
溯源树
样例
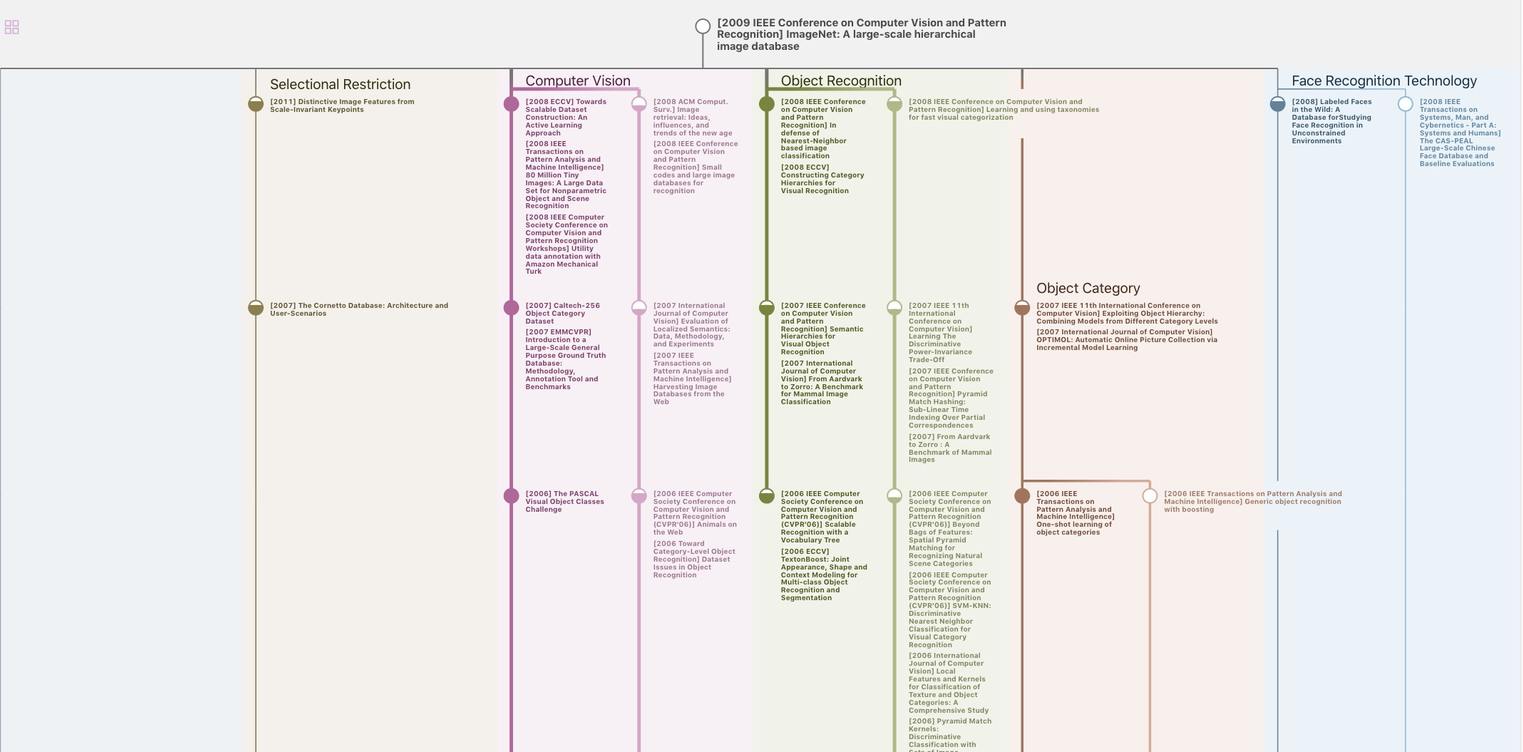
生成溯源树,研究论文发展脉络
Chat Paper
正在生成论文摘要