SpatialCTD: a large-scale TME spatial transcriptomic dataset to evaluate cell type deconvolution for immuno-oncology
bioRxiv (Cold Spring Harbor Laboratory)(2023)
摘要
Recent technological advancements have enabled spatially resolved transcriptomic profiling but at multi-cellular resolution. The task of cell type deconvolution has been introduced to disentangle discrete cell types from such multi-cellular spots. However, existing datasets for cell type deconvolution are limited in scale, predominantly encompassing data on mice, and are not designed for human immuno-oncology. In order to overcome these limitations and promote comprehensive investigation of cell type deconvolution for human immuno-oncology, we introduce a large-scale spatial transcriptomic dataset named SpatialCTD, encompassing 1.8 million cells from the human tumor microenvironment across the lung, kidney, and liver. Distinct from existing approaches that primarily depend on single-cell RNA sequencing data as a reference without incorporating spatial information, we introduce Graph Neural Network-based method (i.e., GNNDeconvolver) that effectively utilize the spatial information from reference samples, and extensive experiments show that GNNDeconvolver often outperforms existing state-of-the-art methods by a substantial margin, without requiring single-cell RNA-seq data. To enable comprehensive evaluations on spatial transcriptomics data from flexible protocols, we provide an online tool capable of converting spatial transcriptomic data from other platforms (e.g., 10x Visium, MERFISH and sci-Space) into pseudo spots, featuring adjustable spot size. The SpatialCTD dataset and GNNDeconvolver implementation are available at , and the online converter tool can be accessed at .
### Competing Interest Statement
The authors have declared no competing interest.
更多查看译文
关键词
spatialctd transcriptomic dataset,cell type deconvolution,large-scale,immuno-oncology
AI 理解论文
溯源树
样例
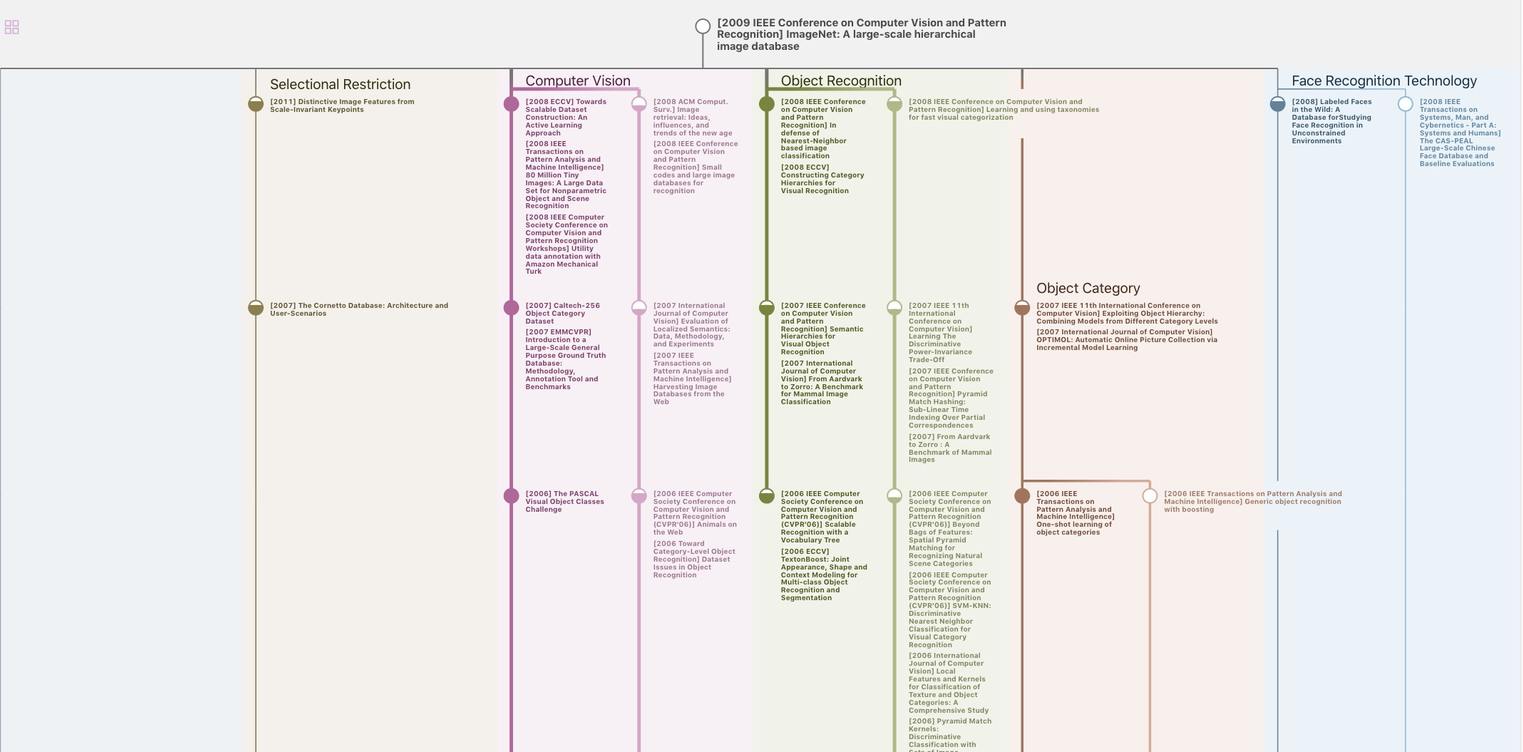
生成溯源树,研究论文发展脉络
Chat Paper
正在生成论文摘要