E2CNN: An Efficient Concatenated CNN for Classification of Surface EMG Extracted From Upper Limb
IEEE Sensors Journal(2023)
摘要
Surface electromyography is a bioelectrical indicator that emerges during muscle contraction and has been widely used in a variety of clinical applications. Several prosthetic control applications can benefit from the analysis based on the classification of surface electromyography (sEMG) signals. However, for the real-time application of upper limb prosthesis, the EMG-based systems need robust performance and rapid response behavior. In this study, we propose an efficient concatenated convolutional neural network (E2CNN) for classification of sEMG extracted from the upper limb. We have tested and validated the performance of the proposed E2CNN on two datasets: a longitudinal dataset comprising ten nondisabled (healthy) subjects and six transradial amputee subjects and spanning the data collected for a period of seven days; and the publicly available NinaPro DB1 dataset. The raw sEMG signals are converted into Log-Mel spectrograms (LMSs). This model combines the input layers with the output of each convolutional block using concatenation layers. The proposed E2CNN when applied to LMS-based images provides a good response time with high-performance accuracy of 98.31% ± 0.5% and 97.97% ± 1.41% for both nondisabled and amputee subjects. When applied to NinaPro DB1, the proposed E2CNN has attained a mean accuracy of 91.27%, an increase by 24.67% with respect to the baseline CNN model. The results show that the achieved results are comparable to those obtained using stacked sparse autoencoders (SSAEs) and other CNN models; however, E2CNN is associated with reduced training and prediction times, making it a potential candidate for real-time classification of sEMG based on LM spectrogram images.
更多查看译文
关键词
Concatenation,convolutional neural network (CNN),Log-Mel spectrogram (LMS),NinaPro DB1,surface electromyography (sEMG)
AI 理解论文
溯源树
样例
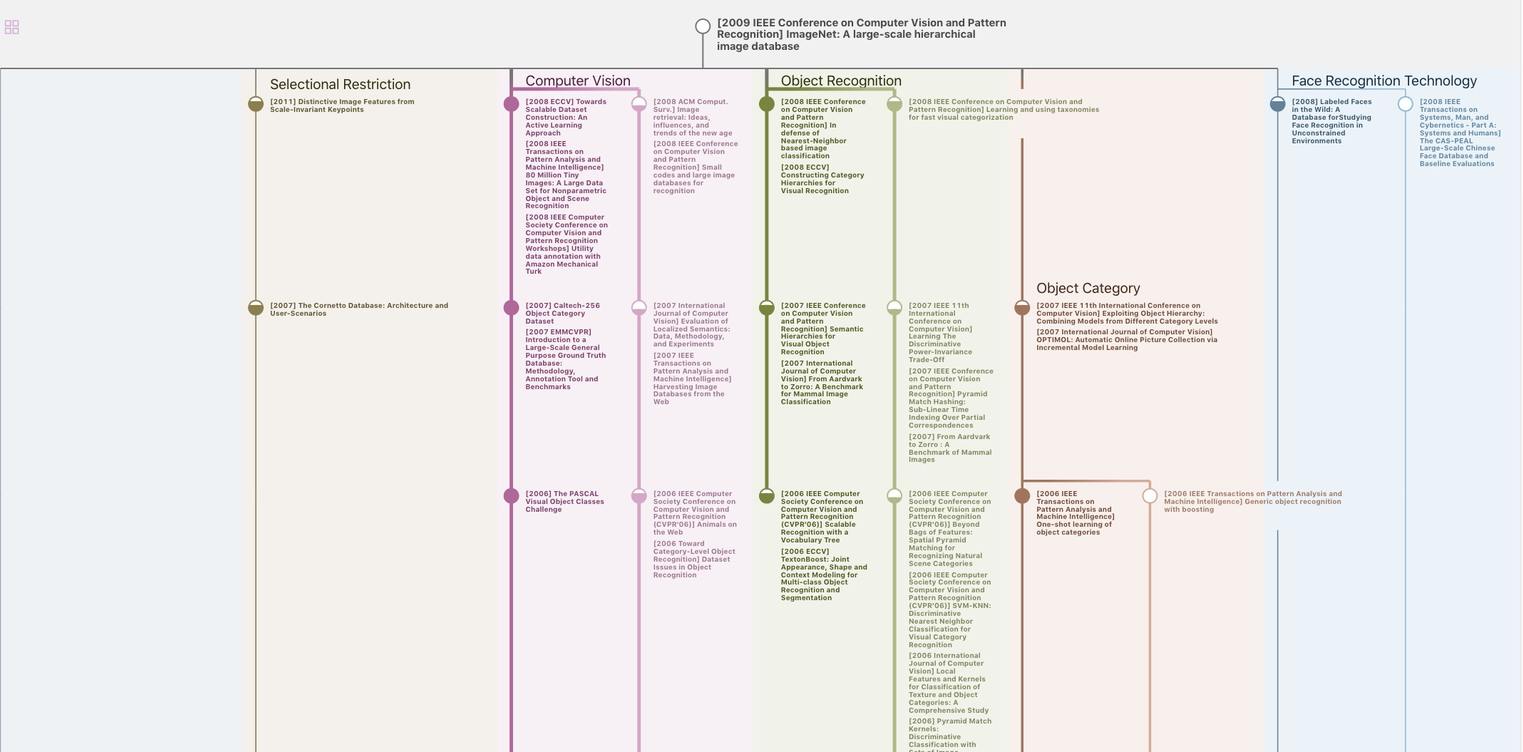
生成溯源树,研究论文发展脉络
Chat Paper
正在生成论文摘要