Structured Pruning for Multi-Task Deep Neural Networks
CoRR(2023)
摘要
Although multi-task deep neural network (DNN) models have computation and storage benefits over individual single-task DNN models, they can be further optimized via model compression. Numerous structured pruning methods are already developed that can readily achieve speedups in single-task models, but the pruning of multi-task networks has not yet been extensively studied. In this work, we investigate the effectiveness of structured pruning on multi-task models. We use an existing single-task filter pruning criterion and also introduce an MTL-based filter pruning criterion for estimating the filter importance scores. We prune the model using an iterative pruning strategy with both pruning methods. We show that, with careful hyper-parameter tuning, architectures obtained from different pruning methods do not have significant differences in their performances across tasks when the number of parameters is similar. We also show that iterative structure pruning may not be the best way to achieve a well-performing pruned model because, at extreme pruning levels, there is a high drop in performance across all tasks. But when the same models are randomly initialized and re-trained, they show better results.
更多查看译文
关键词
structured pruning,neural networks,deep,multi-task
AI 理解论文
溯源树
样例
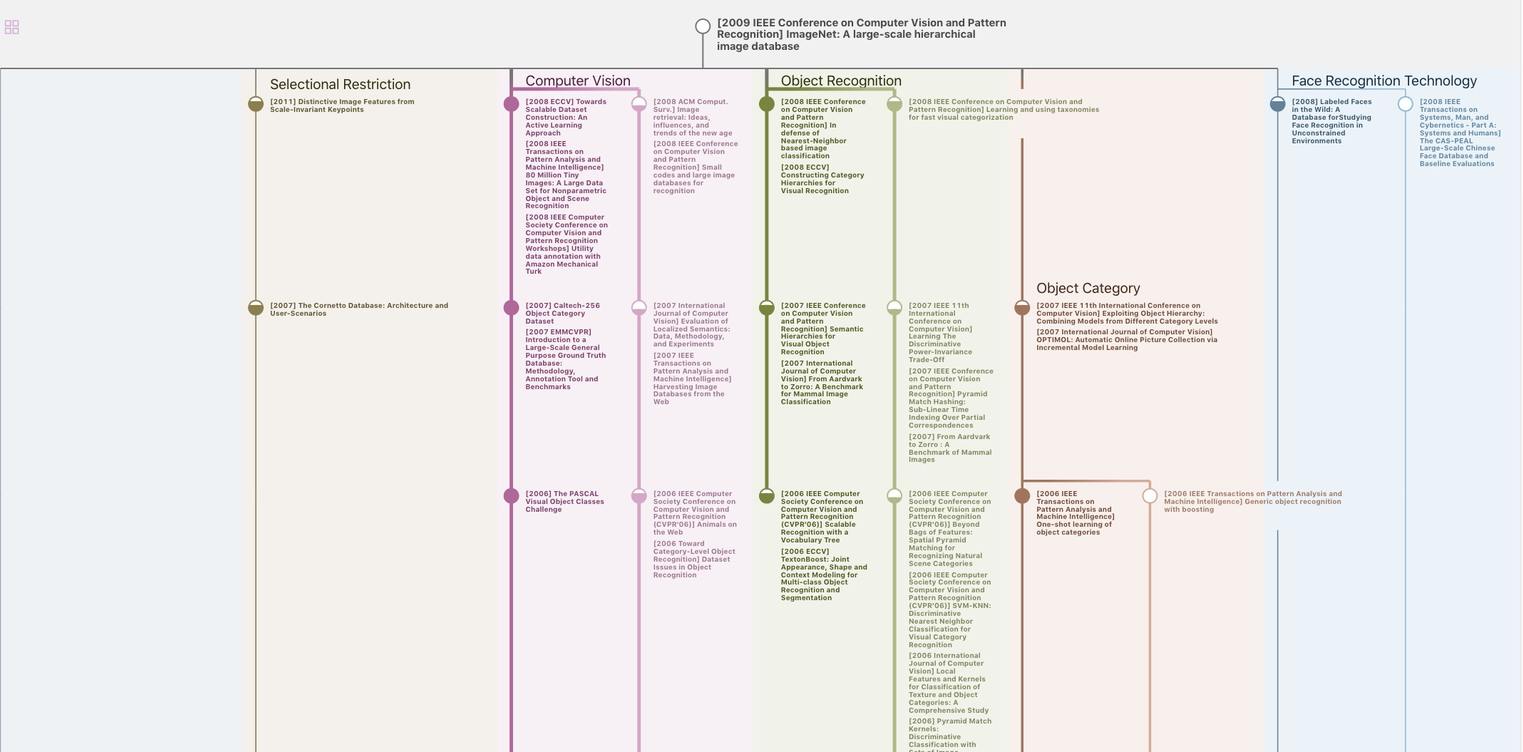
生成溯源树,研究论文发展脉络
Chat Paper
正在生成论文摘要