The advantage of quantum control in many-body Hamiltonian learning
arXiv (Cornell University)(2023)
摘要
We study the problem of learning the Hamiltonian of a many-body quantum system from experimental data. We show that the rate of learning depends on the amount of control available during the experiment. We consider three control models: a 'discrete quantum control' model where the experimentalist can interleave time evolution under the unknown Hamiltonian with instantaneous quantum operations, a 'continuous quantum control' model where the experimentalist can augment the Hamiltonian with bounded control terms, and a model where the experimentalist has no control over the system's time evolution (but can choose initial states and final measurements). With continuous quantum control, we provide an adaptive algorithm for learning a many-body Hamiltonian at the Heisenberg limit, $T = \mathcal{O}(\epsilon^{-1})$, which requires only preparation of product states, time-evolution, and measurement in a product basis. In the absence of quantum control, we prove that learning is standard quantum limited, $T = \Omega(\epsilon^{-2})$, for large classes of many-body Hamiltonians, including any Hamiltonian that thermalizes via the eigenstate thermalization hypothesis. Our no-go results apply even to learning algorithms that utilize quantum memories or involve a limited number of discrete control operations of arbitrary quantum complexity. These results establish a quadratic advantage in experimental runtime for learning with quantum control.
更多查看译文
关键词
hamiltonian learning,quantum control,many-body
AI 理解论文
溯源树
样例
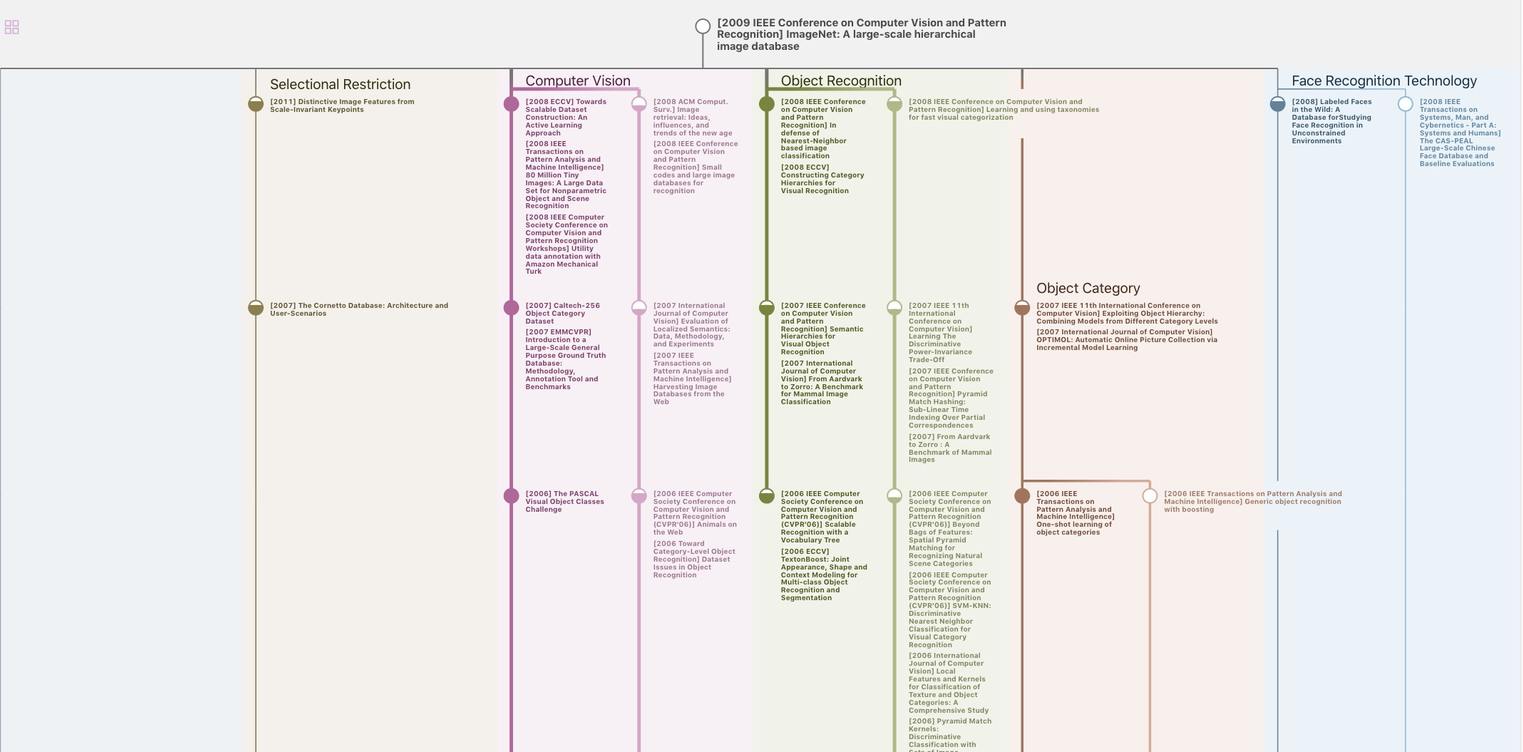
生成溯源树,研究论文发展脉络
Chat Paper
正在生成论文摘要