Synthetically Generating Human-like Data for Sequential Decision Making Tasks via Reward-Shaped Imitation Learning
CoRR(2023)
摘要
We consider the problem of synthetically generating data that can closely resemble human decisions made in the context of an interactive human-AI system like a computer game. We propose a novel algorithm that can generate synthetic, human-like, decision making data while starting from a very small set of decision making data collected from humans. Our proposed algorithm integrates the concept of reward shaping with an imitation learning algorithm to generate the synthetic data. We have validated our synthetic data generation technique by using the synthetically generated data as a surrogate for human interaction data to solve three sequential decision making tasks of increasing complexity within a small computer game-like setup. Different empirical and statistical analyses of our results show that the synthetically generated data can substitute the human data and perform the game-playing tasks almost indistinguishably, with very low divergence, from a human performing the same tasks.
更多查看译文
关键词
sequential decision making,data,human-like,reward-shaped
AI 理解论文
溯源树
样例
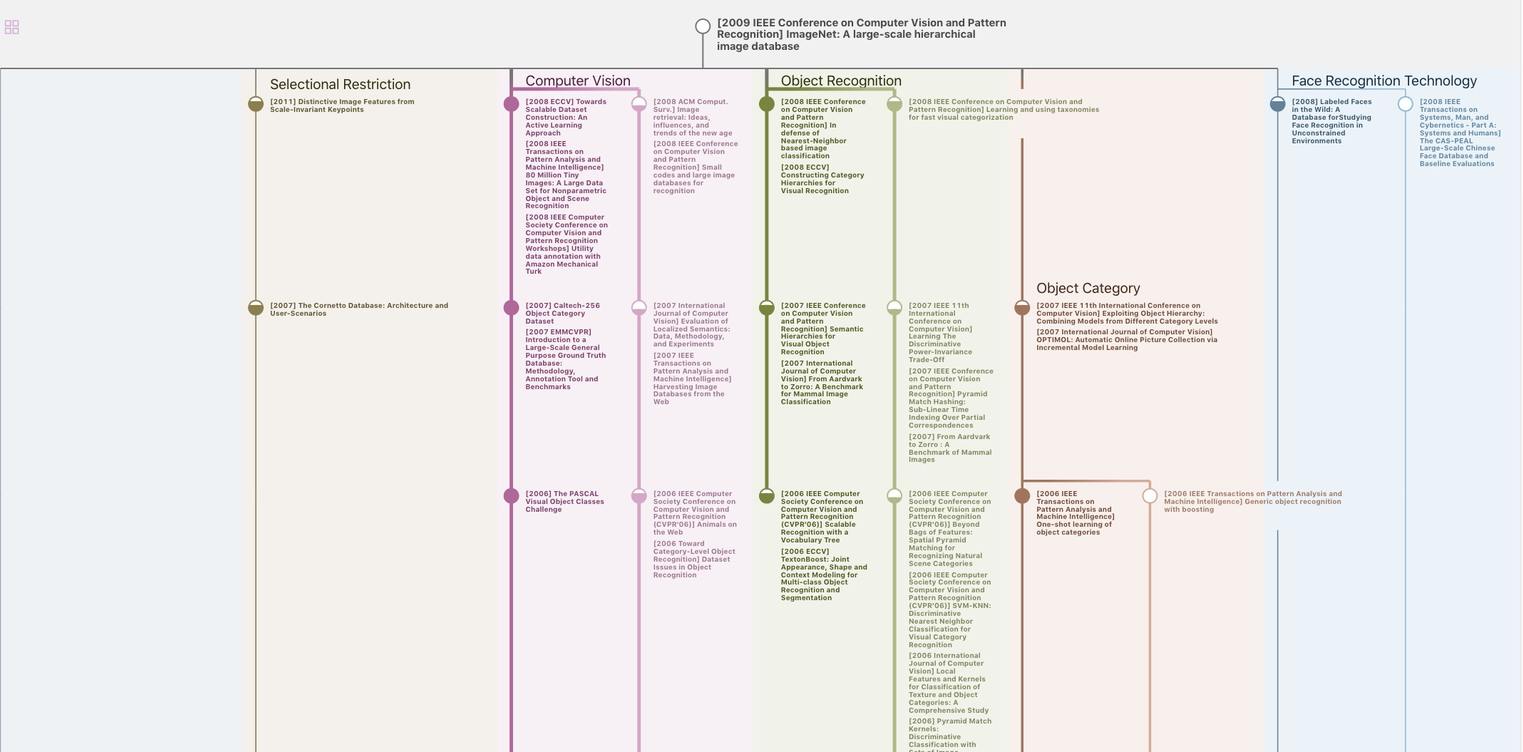
生成溯源树,研究论文发展脉络
Chat Paper
正在生成论文摘要