Short-Term Load Forecasting of Electricity Demand for the Residential Sector Based on Modelling Techniques: A Systematic Review
crossref(2023)
摘要
In this paper, a systematic literature review is presented, through a survey in the main digital databases, about modelling methods for Short-Term Load Forecasting (STLF) for hourly electricity demand for residential electricity and to realize the performance evolution and impact of the Artificial Intelligent (AI) in STLF. With these specific objectives, it was developed a conceptual framework on the subject, a systematic review of the literature based on scientific publications with high impact and a bibliometric study directed to the scientific production of AI and STLF. The review of research articles over a 10-year period, which takes place between 2012-2022, uses the Preferred Reporting Items for Systematic and Meta-Analyses (PRISMA) method. This research resulted in more than 300 articles, available in 4 (four) databases: Web of Science, IEEE Xplore, Scopus and Science Direct. The research was organized around 3 (three) central themes, which were defined through keywords: STLF, Electricity, Residential and their corresponding synonyms. 334 research articles were analyzed and identified the year of publication, journal, author, geography by continent and country and the area of application. Of the 334 documents found in the initial research and after applying the inclusion/exclusion criteria, which allowed delimiting the subject addressed in the topics of interest for analysis, 37 (thirty-seven) documents are in English (25 are journal articles and 12 are conference papers). The results point to a diversity of modelling techniques and associated algorithms. The corresponding performance is measured with different metrics and, therefore, cannot be compared directly. Hence, it should be desirable to have a unified data set together with a set of benchmarks with well-defined metrics for a clear comparison of all the modelling techniques and the corresponding algorithms.
更多查看译文
AI 理解论文
溯源树
样例
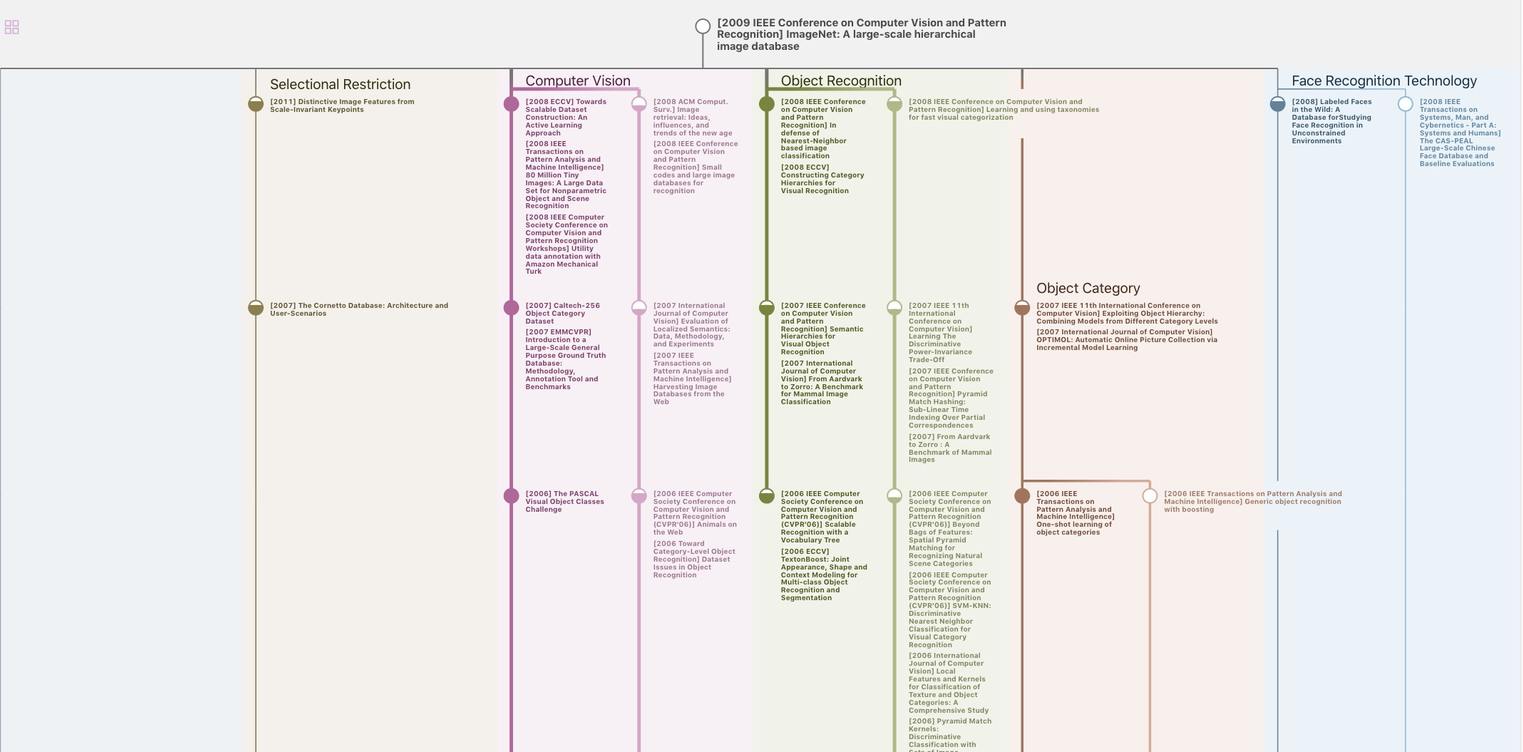
生成溯源树,研究论文发展脉络
Chat Paper
正在生成论文摘要