Rapid Inverse Calibration of a Multiscale Model for the Viscoplastic and Creep Behavior of Short Fiber-Reinforced Thermoplastics Based on Deep Material Networks
International journal of plasticity(2023)
摘要
In this work, we propose to use deep material networks (DMNs) as a surrogate model for full-field computational homogenization to inversely identify material parameters of constitutive inelastic models for short fiber-reinforced thermoplastics (SFRTs).Micromechanics offers an elegant way to obtain constitutive models of materials with complex microstructure, as these emerge naturally once an accurate geometrical representation of the microstructure and expressive material models of the constituents forming the material are known. Unfortunately, obtaining the latter is non-trivial, essentially for two reasons. For a start, experiments on pure samples may not accurately represent the conditions present in the composite. Moreover, the manufacturing process may alter the material behavior, and a subsequent modification is necessary. To avoid modeling the physics of the manufacturing process, one may identify the material models of the individual phases of the composite based on experiments on the composite. Unfortunately, this procedure requires conducting time-consuming simulations.In the work at hand, we use Deep Material Networks to replace full-field simulations, and to carry out an inverse parameter optimization of the matrix model in a SFRT. We are specifically concerned with the long-term creep response of SFRTs, which is particularly challenging to both experimental and simulation-based approaches due to the strong anisotropy and the long time scales involved. We propose a dedicated fully coupled plasticity and creep model for the polymer matrix and provide details on the experimental procedures.
更多查看译文
关键词
Viscoplasticity,Creep,Multiscale model,Deep material networks,Inverse parameter identification,Short fiber reinforced thermoplastics
AI 理解论文
溯源树
样例
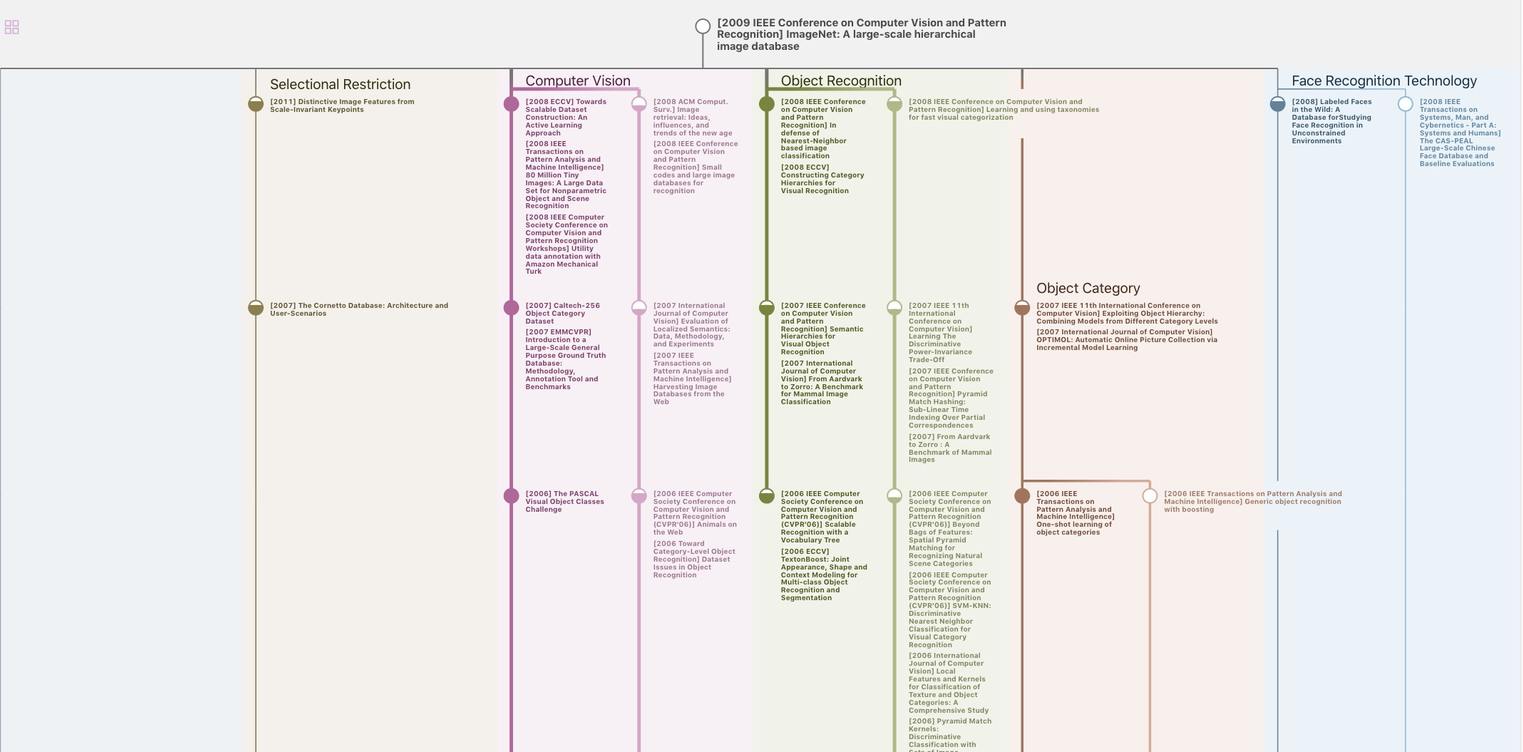
生成溯源树,研究论文发展脉络
Chat Paper
正在生成论文摘要