Prediction of Minimum Horizontal Stress in Oil Wells Using Recurrent Neural Networks
Geoenergy science and engineering(2023)
摘要
The calculation of the minimum horizontal stress (Shmin) is critical for well planning and hydraulic fracture design. The in-situ Shmin can be estimated via borehole injection tests or theoretical approaches. However, these approaches are complex, costly, need unavailable tectonic stress data, and can only be performed at a specified depth. To that end, this paper intends to apply the most recent varieties of recurrent neural networks (RNNs), such as conventional RNN, long-short-term memory (LSTM), and gated recurrent unit (GRU), to Shmin time-series prediction for the first time. In the models, 13,956 datasets including six input parameters effective on the Shmin from an oil well in Iran were used. 80 percent of the data (from depth 1936 m to depth 3637 m) was used for training, while 20 percent (from depth 3637 m to depth 4068 m) was used for testing. All of its hyper-parameters were extensively adjusted to maximize the accuracy of the RNN models. The performance of the RNN models was compared to that of six other machine learning approaches using various statistical criteria. All of the models demonstrated potential capacity to forecast Shmin. However, for Shmin prediction in oil wells, the GRU model with 700 epochs, 32 hidden neurons, 8 batch sizes, 3 hidden layers, ReLU activation function, 6 time series length, Nadam optimization algorithm, and 0.5 dropout rate was recommended. It is possible to reduce significantly the time and costs associated with measuring the Shmin in this manner.
更多查看译文
关键词
Minimum horizontal stress,Machine learning,Recurrent neural networks
AI 理解论文
溯源树
样例
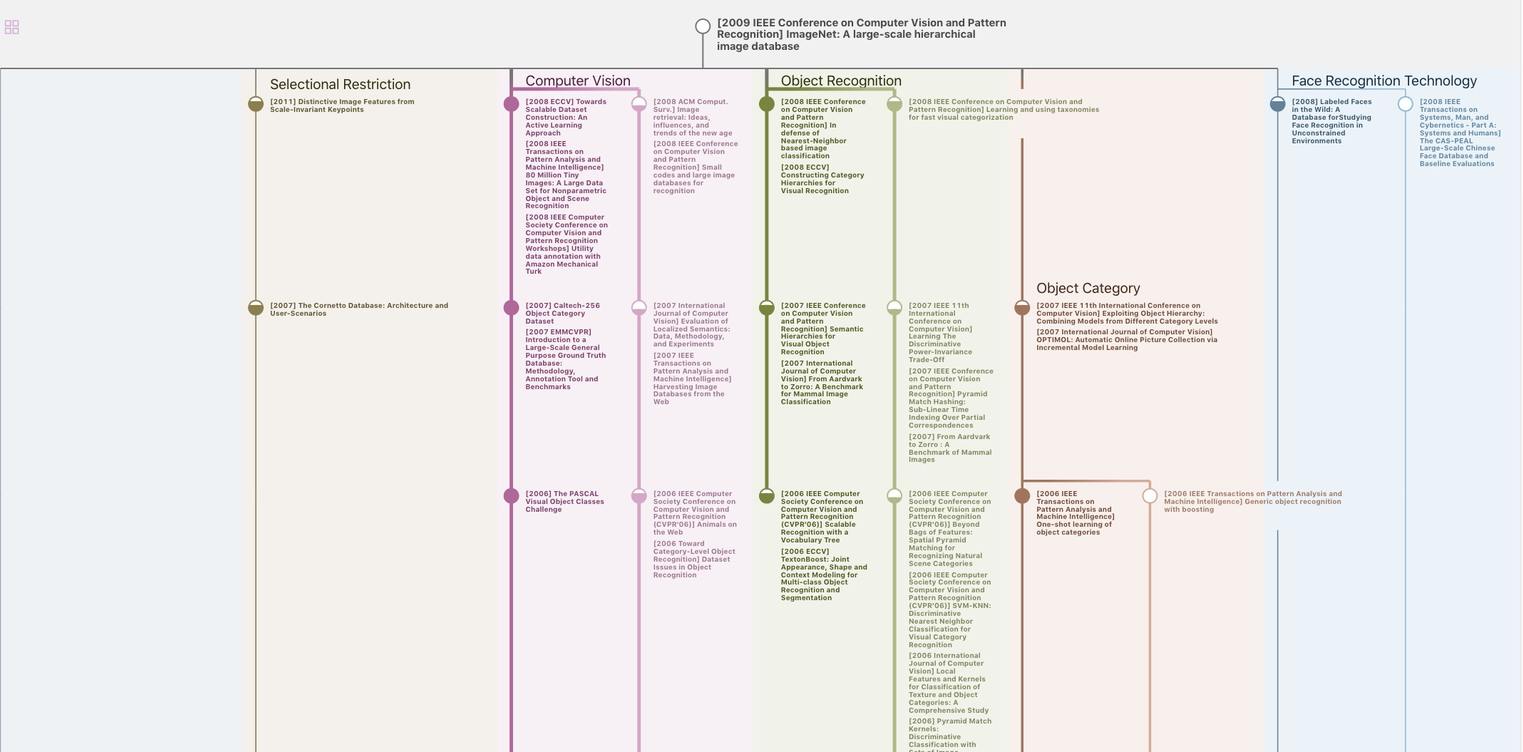
生成溯源树,研究论文发展脉络
Chat Paper
正在生成论文摘要