Region-Enhanced Feature Learning for Scene Semantic Segmentation
CoRR(2023)
摘要
Semantic segmentation in complex scenes not only relies on local object appearance but also on object locations and the surrounding environment. Nonetheless, it is difficult to model long-range context in the format of pairwise point correlations due to its huge computational cost for large-scale point clouds. In this paper, we propose to use regions as the intermediate representation of point clouds instead of fine-grained points or voxels to reduce the computational burden. We introduce a novel Region-Enhanced Feature Learning network (REFL-Net) that leverages region correlations to enhance the features of ambiguous points. We design a Region-based Feature Enhancement module (RFE) which consists of a Semantic-Spatial Region Extraction (SSRE) stage and a Region Dependency Modeling (RDM) stage. In the SSRE stage, we group the input points into a set of regions according to the point distances in both semantic and spatial space. In the RDM part, we explore region-wise semantic and spatial relationships via a self-attention block on region features and fuse point features with the region features to obtain more discriminative representations. Our proposed RFE module is a plug-and-play module that can be integrated with common semantic segmentation backbones. We conduct extensive experiments on ScanNetv2 and S3DIS datasets, and evaluate our RFE module with different segmentation backbones. Our REFL-Net achieves 1.8% mIoU gain on ScanNetv2 and 1.0% mIoU gain on S3DIS respectively with negligible computational cost compared to the backbone networks. Both quantitative and qualitative results show the powerful long-range context modeling ability and strong generalization ability of our REFL-Net.
更多查看译文
关键词
semantic segmentation,region extraction,region dependency modeling
AI 理解论文
溯源树
样例
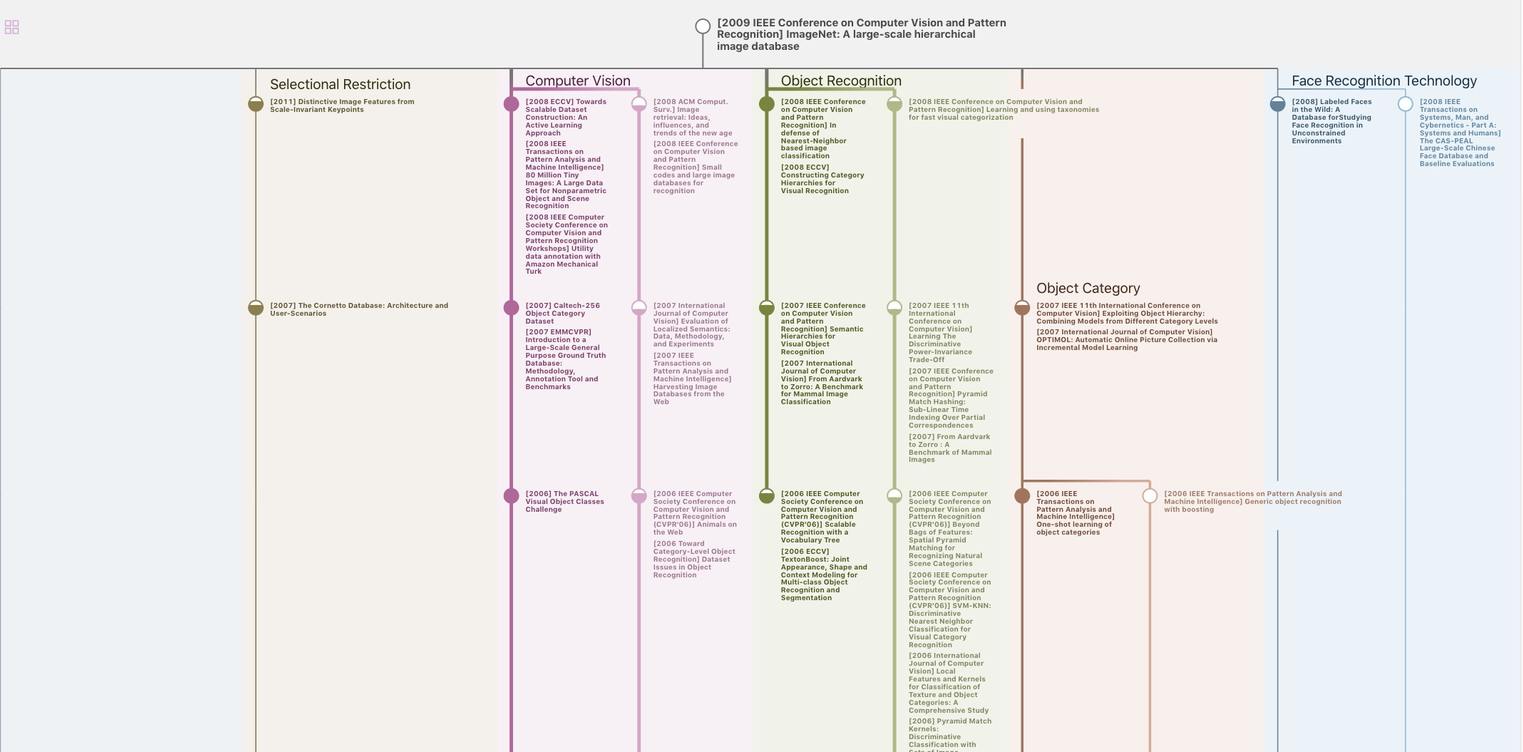
生成溯源树,研究论文发展脉络
Chat Paper
正在生成论文摘要