Multi-Server Secure Aggregation with Unreliable Communication Links
IEEE CONFERENCE ON GLOBAL COMMUNICATIONS, GLOBECOM(2023)
摘要
Federated learning (FL) is a novel training paradigm that allows clients to jointly train models locally without sharing their data. However, it encounters various challenges, such as limited client communication resources, unreliable client-server connections, and the privacy leakage of client information. In this paper, we consider multi-server secure FL with unreliable communication links. We first define a threat model using Shannon's information-theoretic security framework, and propose a novel scheme called Lagrange Coding with Mask (LCM), which introduces client-side masking to reduce the disclosure of client information and injects appropriate coding redundancy to counter the effects of unreliable links while ensuring security. Furthermore, we derive the lower bounds of the uplink and downlink communication loads, respectively, and prove that LCM achieves the optimal uplink communication load, which is unrelated to the number of collusion clients.
更多查看译文
关键词
Coding computing,federated learning,straggling links,secure aggregation
AI 理解论文
溯源树
样例
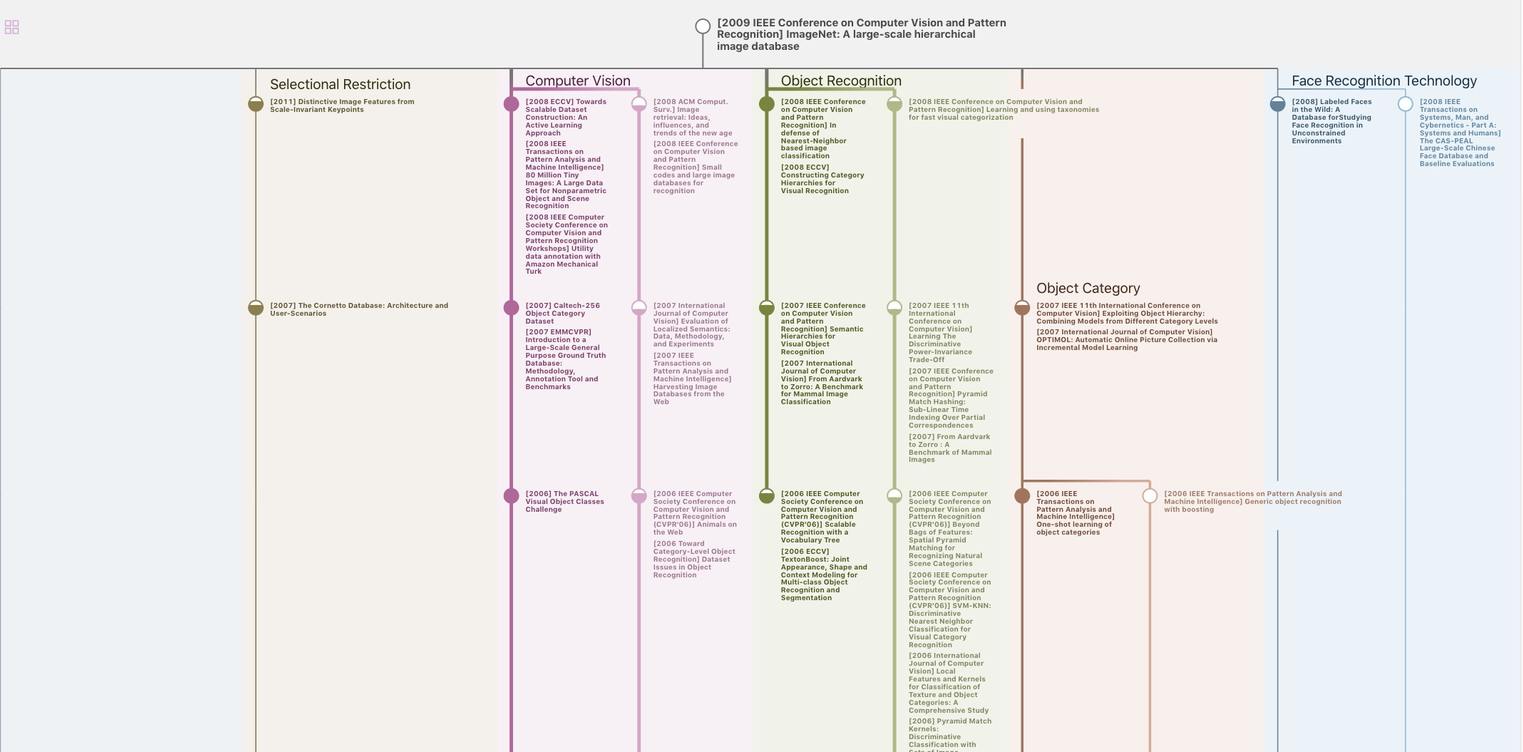
生成溯源树,研究论文发展脉络
Chat Paper
正在生成论文摘要