Dynamic exploration-exploitation trade-off in active learning regression with Bayesian hierarchical modeling
IISE TRANSACTIONS(2024)
摘要
Active learning provides a framework to adaptively query the most informative experiments towards learning an unknown black-box function. Various approaches of active learning have been proposed in the literature, however, they either focus on exploration or exploitation in the design space. Methods that do consider exploration-exploitation simultaneously employ fixed or ad-hoc measures to control the trade-off that may not be optimal. In this article, we develop a Bayesian hierarchical approach, referred to as BHEEM, to dynamically balance the exploration-exploitation trade-off as more data points are queried. To sample from the posterior distribution of the trade-off parameter, we subsequently formulate an approximate Bayesian computation approach based on the linear dependence of queried data in the feature space. Simulated and real-world examples show the proposed approach achieves at least 21% and 11% average improvement when compared to pure exploration and exploitation strategies, respectively. More importantly, we note that by optimally balancing the trade-off between exploration and exploitation, BHEEM performs better or at least as well as either pure exploration or pure exploitation.
更多查看译文
关键词
Active learning regression,exploration-exploitation trade-off,Bayesian hierarchical model,approximate Bayesian computation
AI 理解论文
溯源树
样例
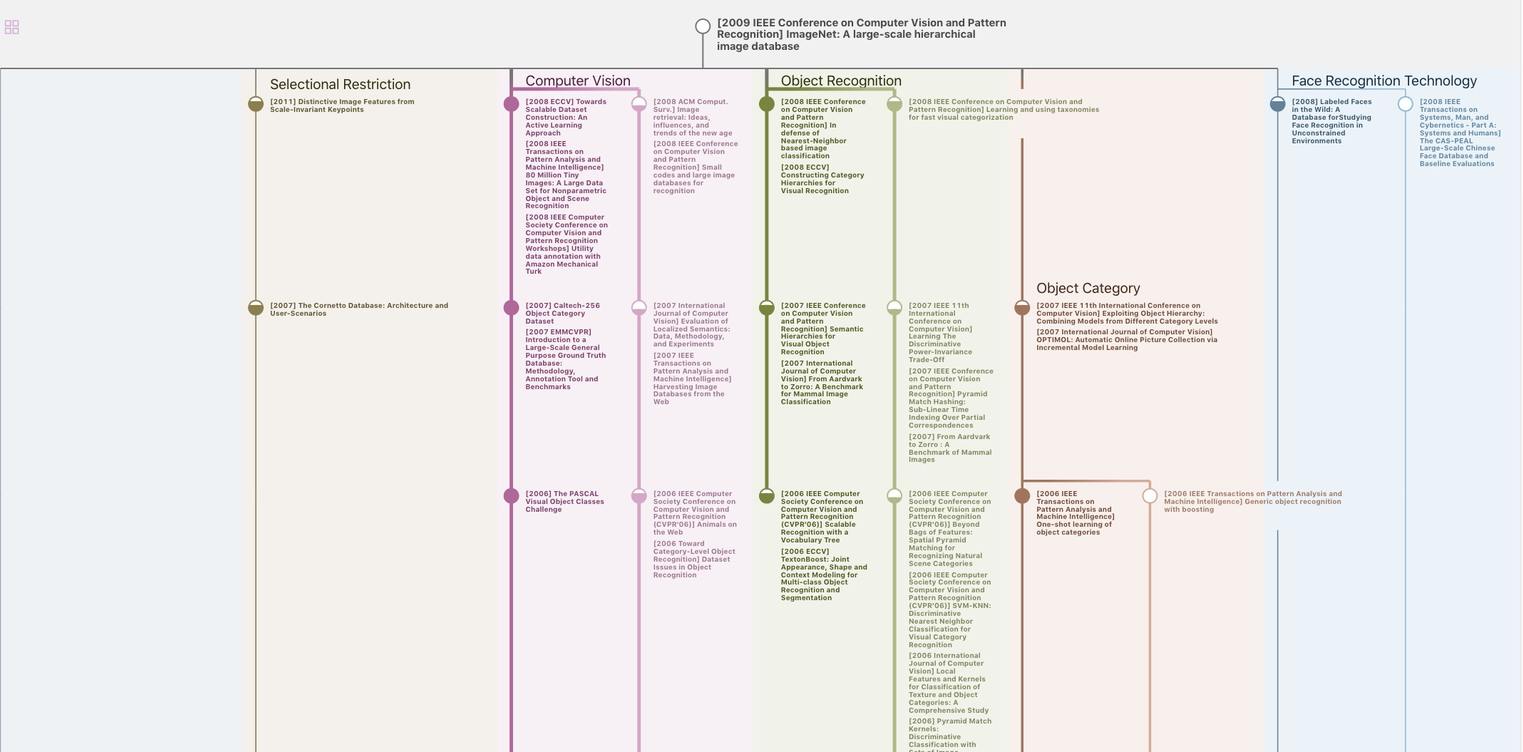
生成溯源树,研究论文发展脉络
Chat Paper
正在生成论文摘要