Causal Disentangled Variational Auto-Encoder for Preference Understanding in Recommendation
PROCEEDINGS OF THE 46TH INTERNATIONAL ACM SIGIR CONFERENCE ON RESEARCH AND DEVELOPMENT IN INFORMATION RETRIEVAL, SIGIR 2023(2023)
摘要
Recommendation models are typically trained on observational user interaction data, but the interactions between latent factors in users' decision-making processes lead to complex and entangled data. Disentangling these latent factors to uncover their underlying representation can improve the robustness, interpretability, and controllability of recommendation models. This paper introduces the Causal Disentangled Variational Auto-Encoder (CaD-VAE), a novel approach for learning causal disentangled representations from interaction data in recommender systems. The CaD-VAE method considers the causal relationships between semantically related factors in real-world recommendation scenarios, rather than enforcing independence as in existing disentanglement methods. The approach utilizes structural causal models to generate causal representations that describe the causal relationship between latent factors. The results demonstrate that CaD-VAE outperforms existing methods, offering a promising solution for disentangling complex user behavior data in recommendation systems.
更多查看译文
关键词
Recommender Systems,Causal Disentangled Representation,Variational Autoencoder
AI 理解论文
溯源树
样例
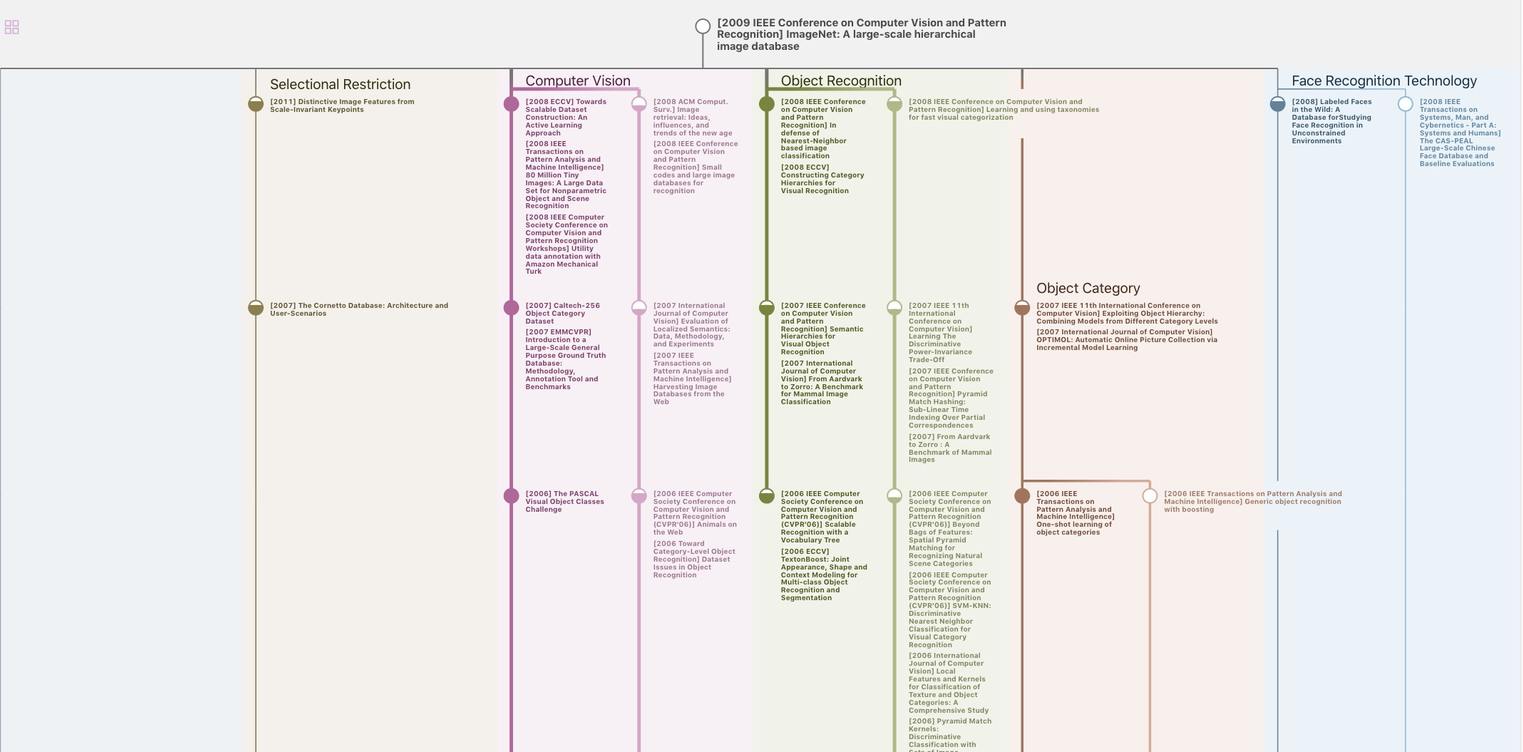
生成溯源树,研究论文发展脉络
Chat Paper
正在生成论文摘要