Integration of Reinforcement Learning Based Behavior Planning With Sampling Based Motion Planning for Automated Driving
CoRR(2023)
摘要
Reinforcement learning has received high research interest for developing planning approaches in automated driving. Most prior works consider the end-to-end planning task that yields direct control commands and rarely deploy their algorithm to real vehicles. In this work, we propose a method to employ a trained deep reinforcement learning policy for dedicated high-level behavior planning. By populating an abstract objective interface, established motion planning algorithms can be leveraged, which derive smooth and drivable trajectories. Given the current environment model, we propose to use a built-in simulator to predict the traffic scene for a given horizon into the future. The behavior of automated vehicles in mixed traffic is determined by querying the learned policy. To the best of our knowledge, this work is the first to apply deep reinforcement learning in this manner, and as such lacks a state-of-the-art benchmark. Thus, we validate the proposed approach by comparing an idealistic single-shot plan with cyclic replanning through the learned policy. Experiments with a real testing vehicle on proving grounds demonstrate the potential of our approach to shrink the simulation to real world gap of deep reinforcement learning based planning approaches. Additional simulative analyses reveal that more complex multi-agent maneuvers can be managed by employing the cycling replanning approach.
更多查看译文
关键词
abstract objective interface,automated driving,automated vehicles,current environment model,dedicated high-level behavior planning,end-to-end planning,established motion planning algorithms,high research interest,idealistic single-shot plan,learned policy,planning approaches,sampling based motion planning,testing vehicle,trained deep reinforcement learning policy,yields direct control commands
AI 理解论文
溯源树
样例
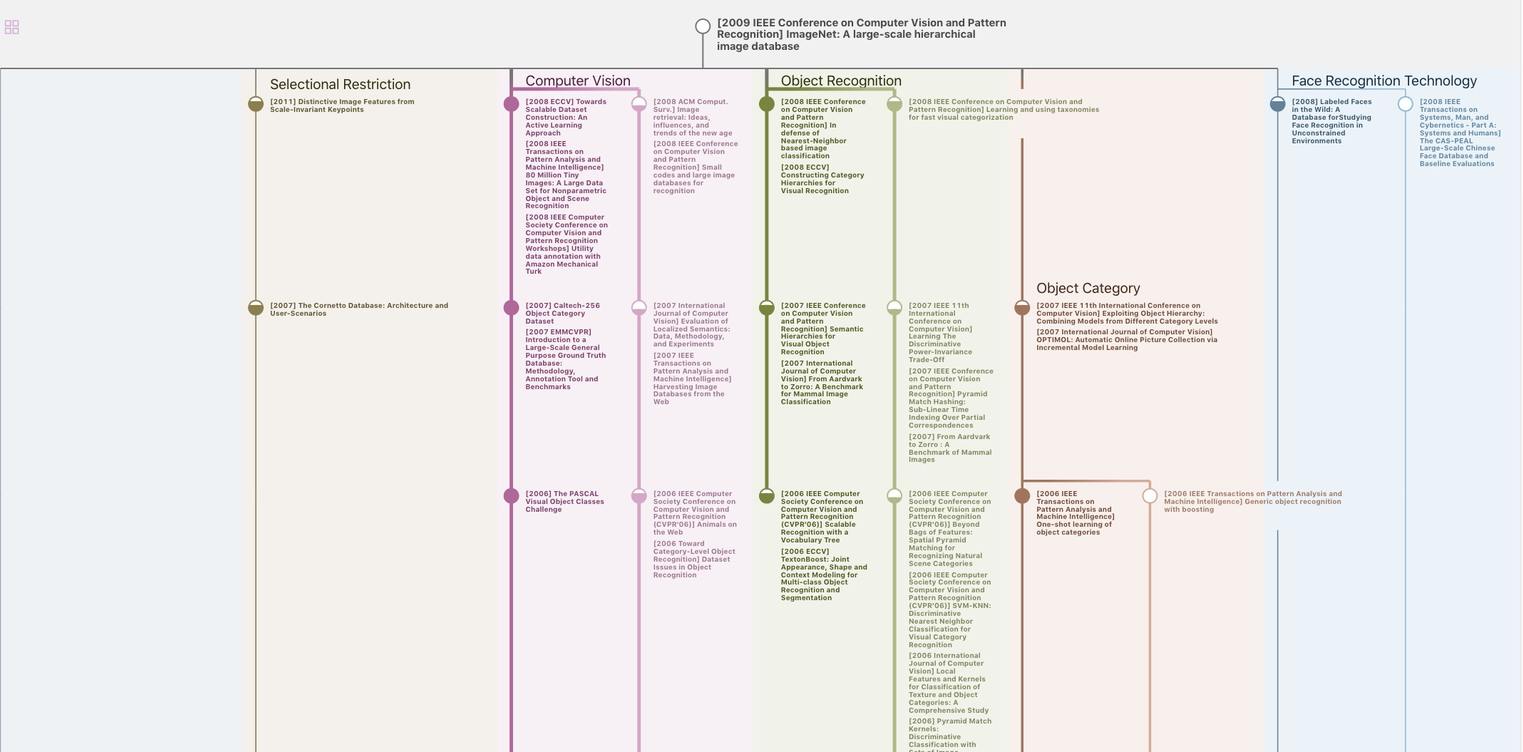
生成溯源树,研究论文发展脉络
Chat Paper
正在生成论文摘要