Laboratory and field evaluation of a low-cost methane sensor and key environmental factors for sensor calibration.
Environmental science: atmospheres(2023)
摘要
Low-cost sensors enable finer-scale spatiotemporal measurements within the existing methane (CH) monitoring infrastructure and could help cities mitigate CH emissions to meet their climate goals. While initial studies of low-cost CH sensors have shown potential for effective CH measurement at ambient concentrations, sensor deployment remains limited due to questions about interferences and calibration across environments and seasons. This study evaluates sensor performance across seasons with specific attention paid to the sensor's understudied carbon monoxide (CO) interferences and environmental dependencies through long-term ambient co-location in an urban environment. The sensor was first evaluated in a laboratory using chamber calibration and co-location experiments, and then in the field through two 8 week co-locations with a reference CH instrument. In the laboratory, the sensor was sensitive to CH concentrations below ambient background concentrations. Different sensor units responded similarly to changing CH, CO, temperature, and humidity conditions but required individual calibrations to account for differences in sensor response factors. When deployed in-field, co-located with a reference instrument near Baltimore, MD, the sensor captured diurnal trends in hourly CH concentration after corrections for temperature, absolute humidity, CO concentration, and hour of day. Variable performance was observed across seasons with the sensor performing well ( = 0.65; percent bias 3.12%; RMSE 0.10 ppm) in the winter validation period and less accurately ( = 0.12; percent bias 3.01%; RMSE 0.08 ppm) in the summer validation period where there was less dynamic range in CH concentrations. The results highlight the utility of sensor deployment in more variable ambient CH conditions and demonstrate the importance of accounting for temperature and humidity dependencies as well as co-located CO concentrations with low-cost CH measurements. We show this can be addressed Multiple Linear Regression (MLR) models accounting for key covariates to enable urban measurements in areas with CH enhancement. Together with individualized calibration prior to deployment, the sensor shows promise for use in low-cost sensor networks and represents a valuable supplement to existing monitoring strategies to identify CH hotspots.
更多查看译文
AI 理解论文
溯源树
样例
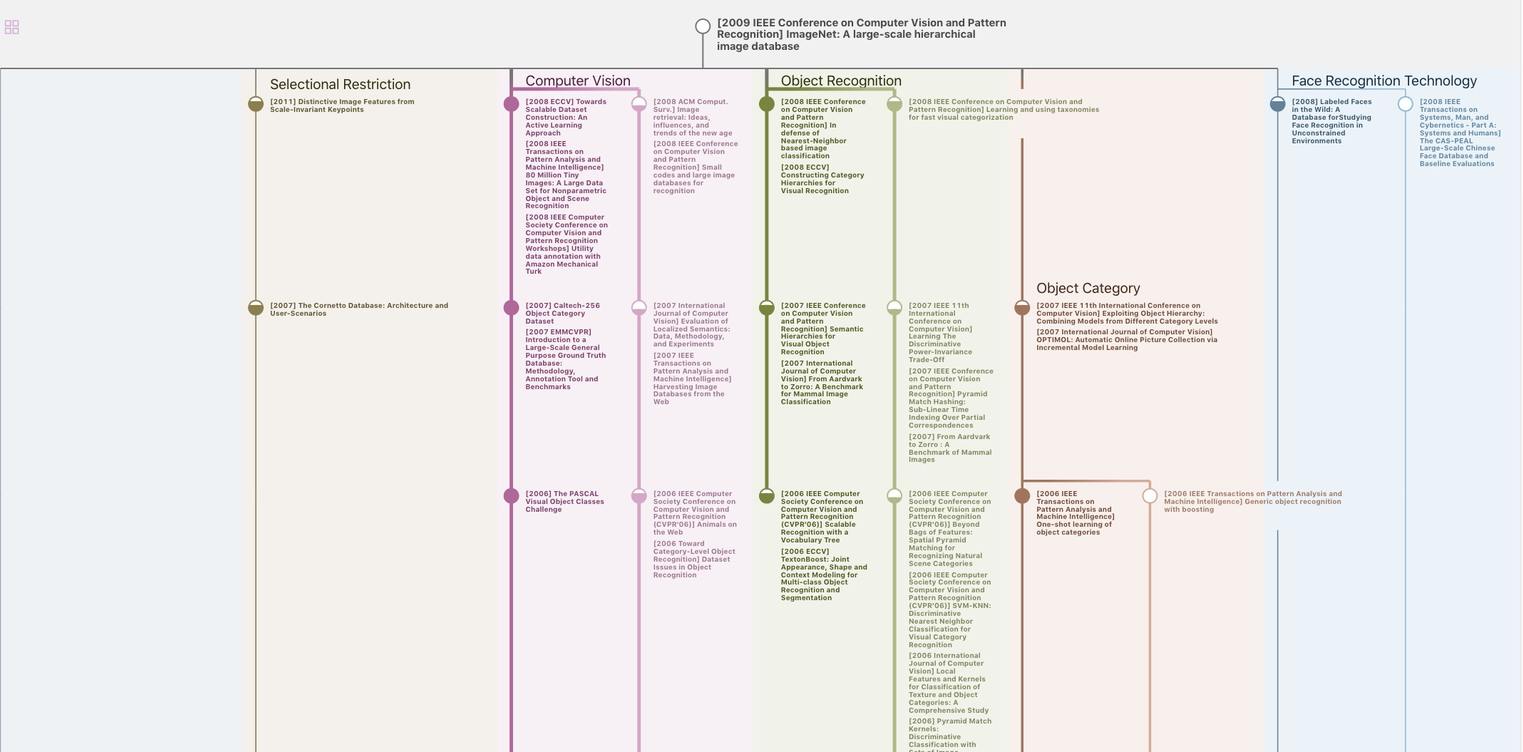
生成溯源树,研究论文发展脉络
Chat Paper
正在生成论文摘要