A cascaded deep learning framework for photovoltaic power forecasting with multi-fidelity inputs
Energy(2023)
摘要
Accurate forecasts of photovoltaic power (PVP) are essential to the production, transmission, and distribution of electricity in power systems. However, PVP output is strongly weather-dependent, and the forecasting of PVP is highly dependent on the quality of numerical weather prediction (NWP) data. In recent years, a huge volume of numerical weather observation (NWO) data which are strongly associated with PVP output have been collected on-site by widely-installed smart meters and sensors. Appropriately utilizing high-fidelity NWO, in addition to low-fidelity NWP, has great potential in promoting the forecasting capability of deep learning (DL) models. Therefore, this paper proposes a cascaded multi-fidelity deep learning (CMF-DL) framework, which is coordinately driven by the data of both NWO and NWP, to deal with the day-ahead PVP forecasting problem. The proposed CMF-DL framework possesses great compatibility, and thus it can be incorporated with various DL models, such as the long short-term memory (LSTM) model and the gated recurrent unit (GRU) model. Subsequently, incorporated with CMF-DL, two newly-developed forecasting models, i.e., CMF-LSTM and CMF-GRU, are proposed, and datasets from a real-life PV plant are utilized, to evaluate the feasibility and effectiveness of the proposed approaches. From the results, the proposed CMF-LSTM and CMF-GRU show greater forecasting capability and anti-noise ability than the basic LSTM and GRU. Both CMF-LSTM and CMF-GRU can accept noisy NWP data with up to 35% errors. Additionally, compared to the persistence model, the forecasting skills of CMF-LSTM and CMF-GRU can be significantly promoted by 39.87% and 44.02%, respectively. The proposed CMF-LSTM and CMF-GRU also achieve better day-ahead PVP forecasting performance than the widely-used reference models in previous works.
更多查看译文
关键词
Deep learning,Photovoltaic power forecasting,Multi-fidelity inputs,Numerical weather observation,Numerical weather prediction
AI 理解论文
溯源树
样例
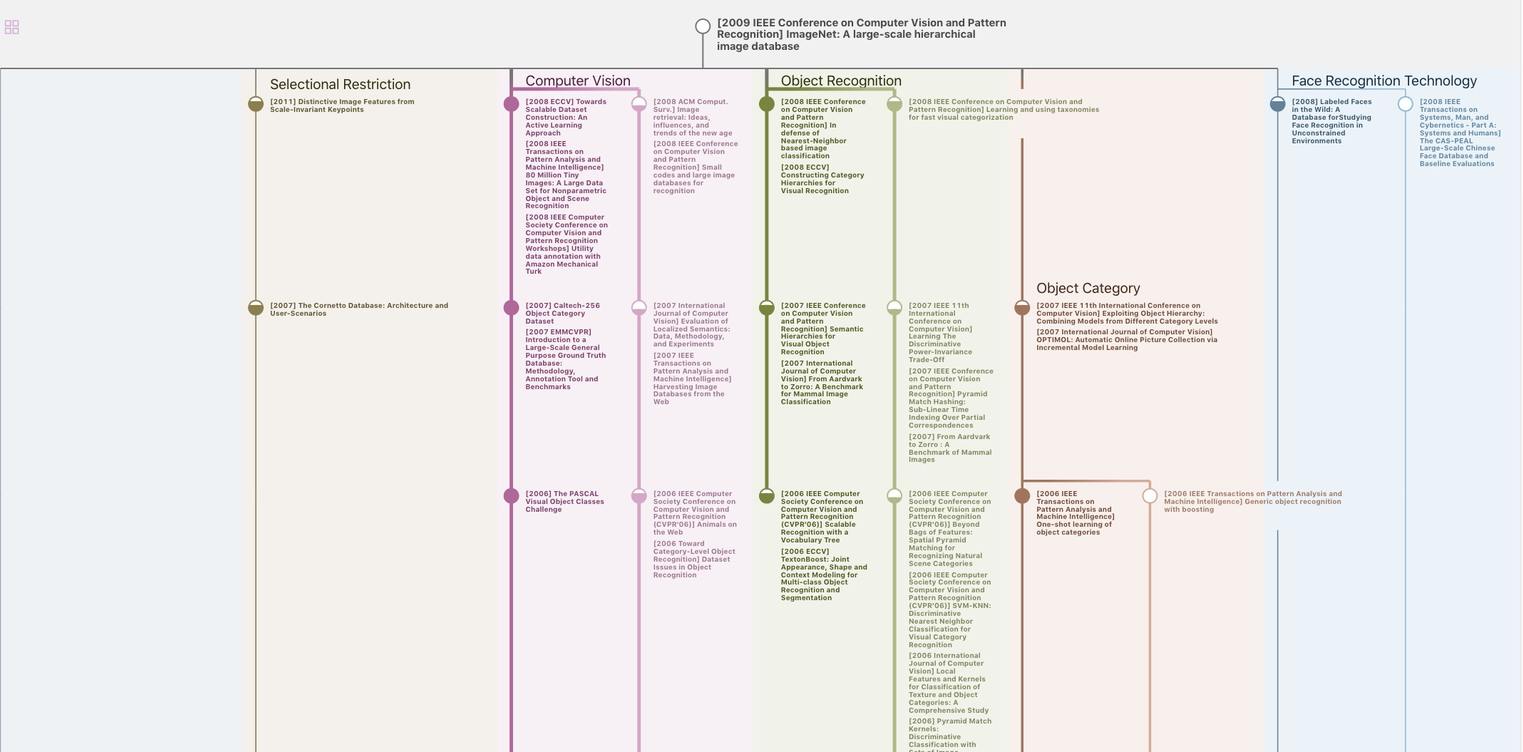
生成溯源树,研究论文发展脉络
Chat Paper
正在生成论文摘要