Data-driven heat pump operation strategy using rainbow deep reinforcement learning for significant reduction of electricity cost
Energy(2023)
摘要
The need for reducing carbon emissions and achieving “Net Zero” energy has made improving heat pumps’ (HPs) operational efficiency a crucial goal. However, current rule or model-based control strategies have limitations of inability to consider the entire heat production-storage-utilization cycle and inherent difficulties in achieving both high performance and generality. Here, we propose a model-free deep reinforcement learning (DRL)-based HP operation strategy that utilizes the Rainbow deep Q network algorithm to minimize electricity costs by considering thermal load demand, renewable generation, coefficient of performance (COP) of HPs, and state of charge (SOC) of thermal storage. We employ artificial neural networks to train for the regression of future load demands and COP, creating a data-driven and connectable environment with DRL. The Rainbow agent learns a creative strategy of limiting the maximum number of HP operations by increasing the SOC in advance to match future load demands. The performance of the Rainbow agent is evaluated against rule-based control in cases of future states, future uncertainty, and five-year long-term deployment. The proposed method reduces the year-round demand charge by 23.1% and the energy charge by 21.7%, resulting in a 22.2% reduction in the electricity cost.
更多查看译文
关键词
Deep reinforcement learning,Heat pump,Electricity cost,Rainbow deep Q network,Load demand,Renewable energy
AI 理解论文
溯源树
样例
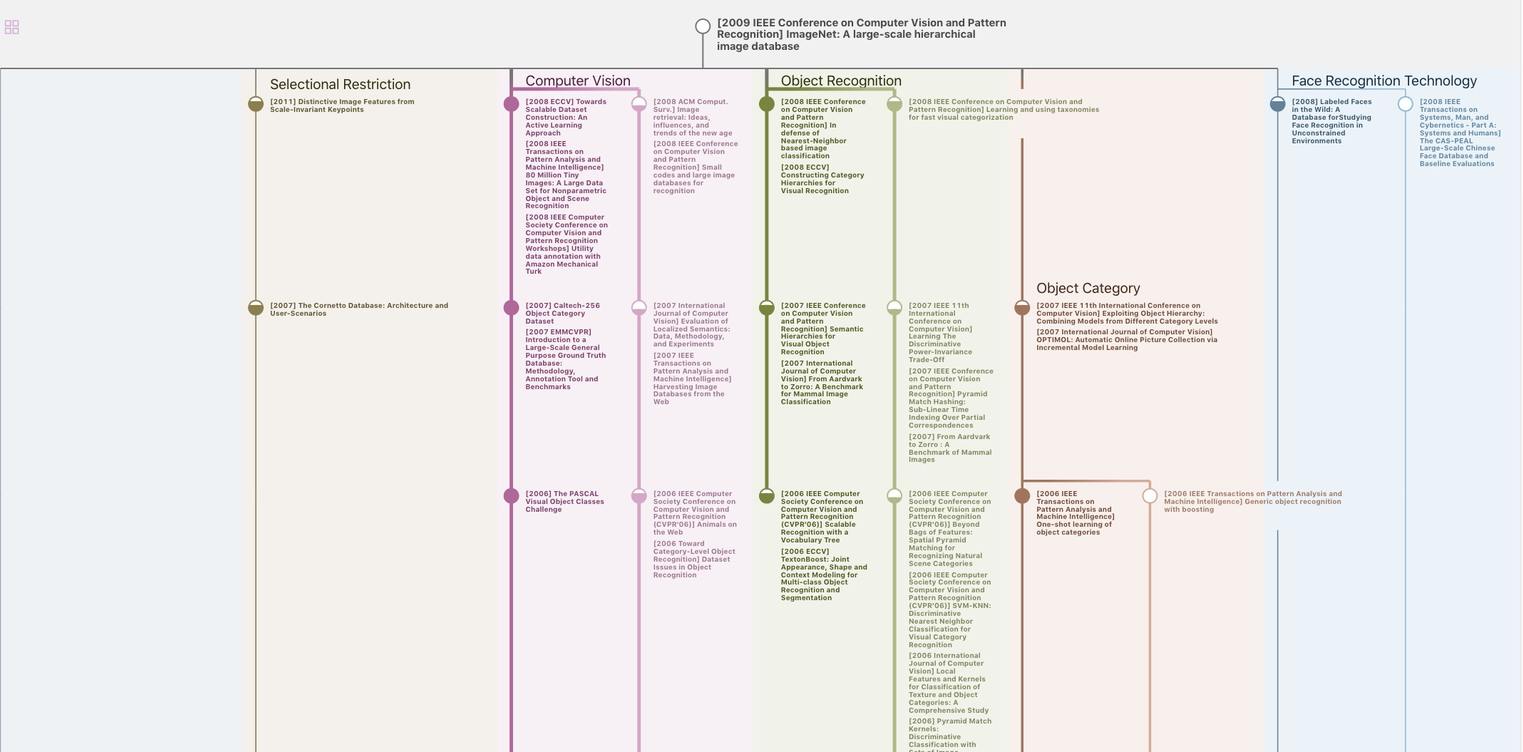
生成溯源树,研究论文发展脉络
Chat Paper
正在生成论文摘要