Avatars Grow Legs: Generating Smooth Human Motion From Sparse Tracking Inputs With Diffusion Model
CVPR 2023(2023)
摘要
With the recent surge in popularity of AR/VR applications, realistic and accurate control of 3D full-body avatars has become a highly demanded feature. A particular challenge is that only a sparse tracking signal is available from standalone HMDs (Head Mounted Devices), often limited to tracking the user's head and wrists. While this signal is resourceful for reconstructing the upper body motion, the lower body is not tracked and must be synthesized from the limited information provided by the upper body joints. In this paper, we present AGRoL, a novel conditional diffusion model specifically designed to track full bodies given sparse upper-body tracking signals. Our model is based on a simple multi-layer perceptron (MLP) architecture and a novel conditioning scheme for motion data. It can predict accurate and smooth full-body motion, particularly the challenging lower body movement. Unlike common diffusion architectures, our compact architecture can run in real-time, making it suitable for online body-tracking applications. We train and evaluate our model on AMASS motion capture dataset, and demonstrate that our approach outperforms state-of-the-art methods in generated motion accuracy and smoothness. We further justify our design choices through extensive experiments and ablation studies.
更多查看译文
关键词
Humans: Face,body,pose,gesture,movement
AI 理解论文
溯源树
样例
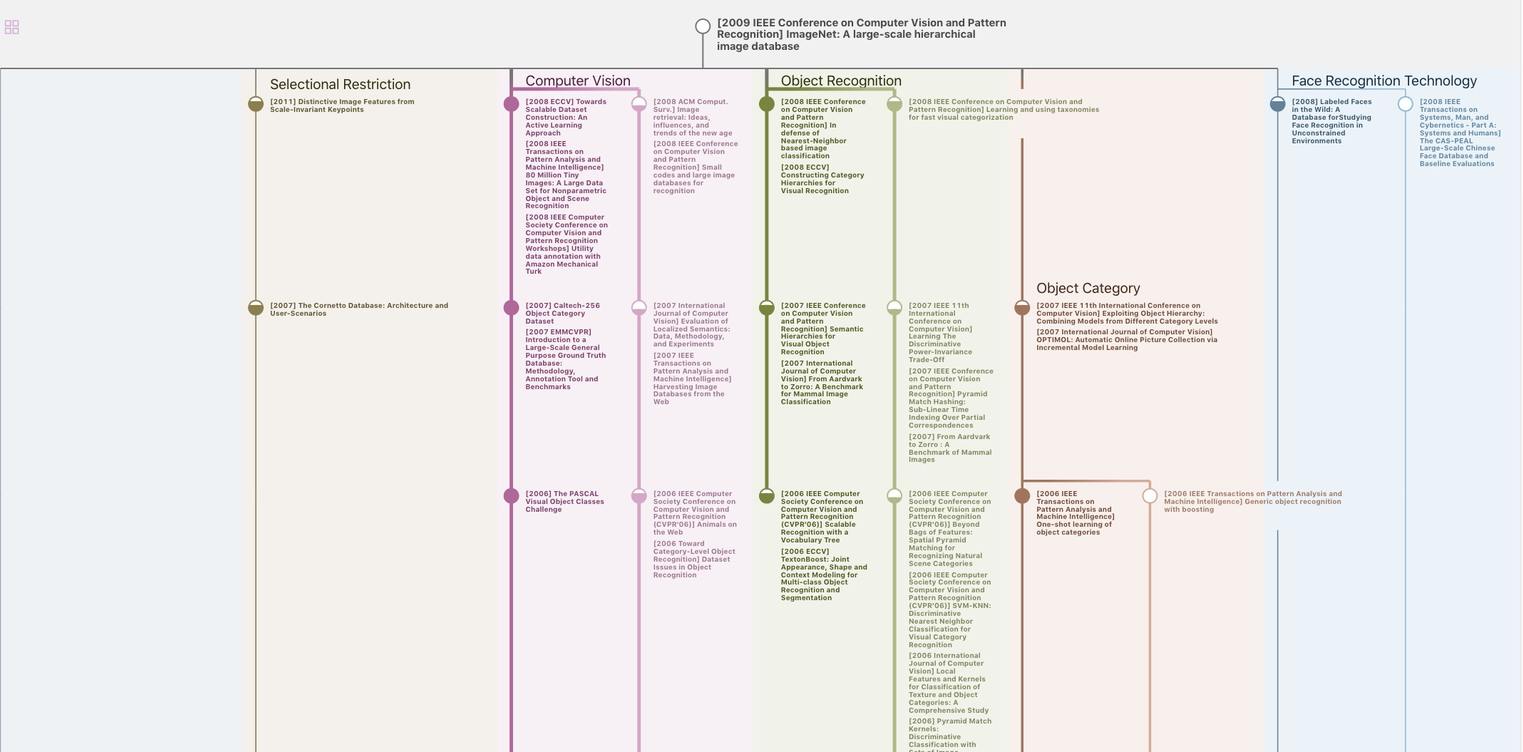
生成溯源树,研究论文发展脉络
Chat Paper
正在生成论文摘要