Neural Speech Enhancement with Very Low Algorithmic Latency and Complexity via Integrated Full- and Sub-Band Modeling
CoRR(2023)
摘要
We propose FSB-LSTM, a novel long short-term memory (LSTM) based architecture that integrates full- and sub-band (FSB) modeling, for single- and multi-channel speech enhancement in the short-time Fourier transform (STFT) domain. The model maintains an information highway to flow an over-complete input representation through multiple FSB-LSTM modules. Each FSB-LSTM module consists of a full-band block to model spectro-temporal patterns at all frequencies and a sub-band block to model patterns within each sub-band, where each of the two blocks takes a down-sampled representation as input and returns an up-sampled discriminative representation to be added to the block input via a residual connection. The model is designed to have a low algorithmic complexity, a small run-time buffer and a very low algorithmic latency, at the same time producing a strong enhancement performance on a noisy-reverberant speech enhancement task even if the hop size is as low as $2$ ms.
更多查看译文
关键词
Low-complexity speech enhancement,frame-online speech enhancement,deep learning,hearing aids design
AI 理解论文
溯源树
样例
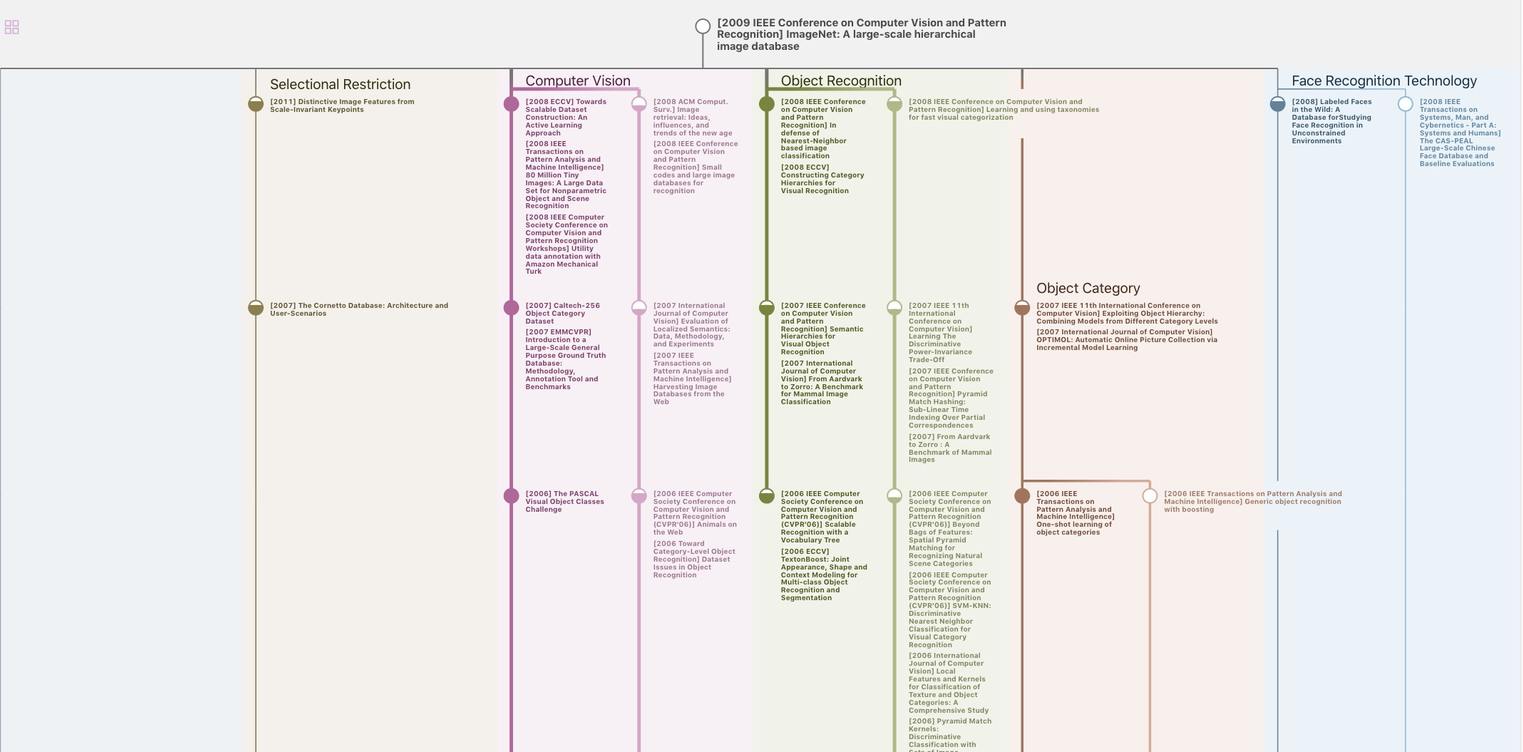
生成溯源树,研究论文发展脉络
Chat Paper
正在生成论文摘要