Prediction of equivalent sand-grain size and identification of drag-relevant scales of roughness - a data-driven approach
JOURNAL OF FLUID MECHANICS(2023)
摘要
Despite decades of research, a universal method for prediction of roughness-induced skin friction in a turbulent flow over an arbitrary rough surface is still elusive. The purpose of the present work is to examine two possibilities; first, predicting equivalent sand-grain roughness size k(s) based on the roughness height probability density function and power spectrum (PS) leveraging machine learning as a regression tool; and second, extracting information about relevance of different roughness scales to skin-friction drag by interpreting the output of the trained data-driven model. The model is an ensemble neural network (ENN) consisting of 50 deep neural networks. The data for the training of the model are obtained from direct numerical simulations (DNS) of turbulent flow in plane channels over 85 irregular multi-scale roughness samples at friction Reynolds number Re-tau =800. The 85 roughness samples are selected from a repository of 4200 samples, covering a wide parameter space, through an active learning (AL) framework. The selection is made in several iterations, based on the informativeness of samples in the repository, quantified by the variance of ENN predictions. This AL framework aims to maximize the generalizability of the predictions with a certain amount of data. This is examined using three different testing data sets with different types of roughness, including 21 surfaces from the literature. The model yields overall mean error 5 %-10 % on different testing data sets. Subsequently, a data interpretation technique, known as layer-wise relevance propagation, is applied to measure the contributions of different roughness wavelengths to the predicted k(s). High-pass filtering is then applied to the roughness PS to exclude the wavenumbers identified as drag-irrelevant. The filtered rough surfaces are investigated using DNS, and it is demonstrated that despite significant impact of filtering on the roughness topographical appearance and statistics, the skin-friction coefficient of the original roughness is preserved successfully.
更多查看译文
关键词
turbulent flows
AI 理解论文
溯源树
样例
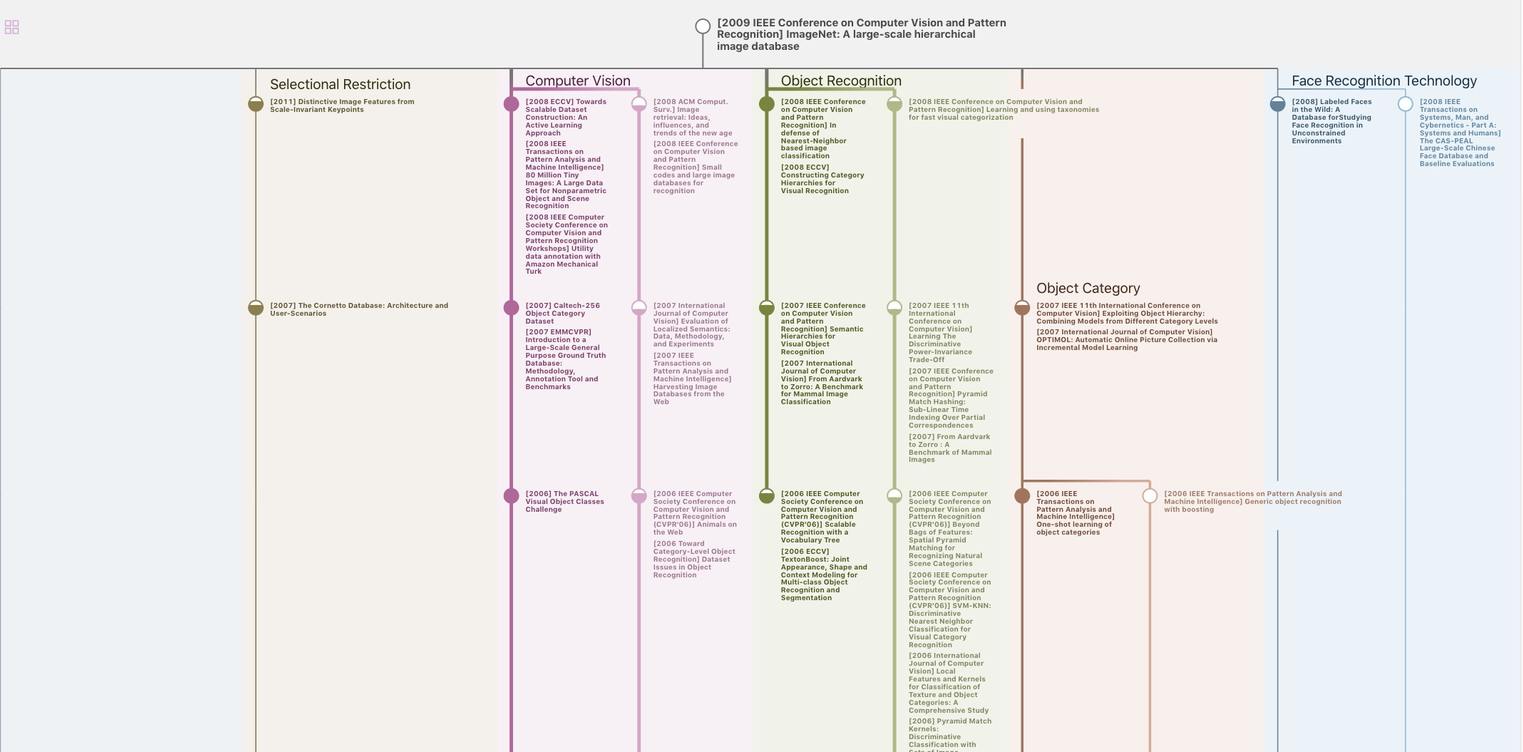
生成溯源树,研究论文发展脉络
Chat Paper
正在生成论文摘要