Fault-Tolerant Predictive Control With Deep-Reinforcement-Learning-Based Torque Distribution for Four In-Wheel Motor Drive Electric Vehicles
IEEE/ASME Transactions on Mechatronics(2023)
摘要
This article proposes a fault-tolerant control (FTC) method for four in-wheel motor drive electric vehicles considering both vehicle stability and motor power consumption. First, a seven-degree-of-freedom vehicle nonlinear model integrating motor faults is built to design a hierarchical FTC control scheme. The control structure is composed of two levels: an upper level nonlinear model-predictive controller and a lower level fault-tolerant coordinated controller. The upper level controller provides an appropriate reference in terms of additional yaw moment and vehicle longitudinal force, required for vehicle stability control, to the lower level controller. This latter aims at distributing the four-wheel torques taking into account both vehicle stability and power consumption. Specifically, the weighting factor involved in the optimization-based design of the lower level controller is determined online by the randomized ensembled double
$Q$
-learning reinforcement learning algorithm to achieve an optimal control strategy for the whole vehicle operating range. Moreover, the tradeoff between vehicle stability and power consumption is analyzed, and the necessity of using reinforcement learning is discussed. Numerical experiments are performed under various driving scenarios with a high-fidelity CarSim vehicle model to demonstrate the effectiveness of the proposed control method. Via a comparative study, we highlight the advantages of the new FTC control method over many related existing control results in terms of improving the vehicle stability and driver comfort as well as reducing the power consumption.
更多查看译文
关键词
Electric ground vehicles,fault-tolerant control (FTC),in-wheel/hub motor,reinforcement learning (RL),torque vectoring,vehicle motion dynamics
AI 理解论文
溯源树
样例
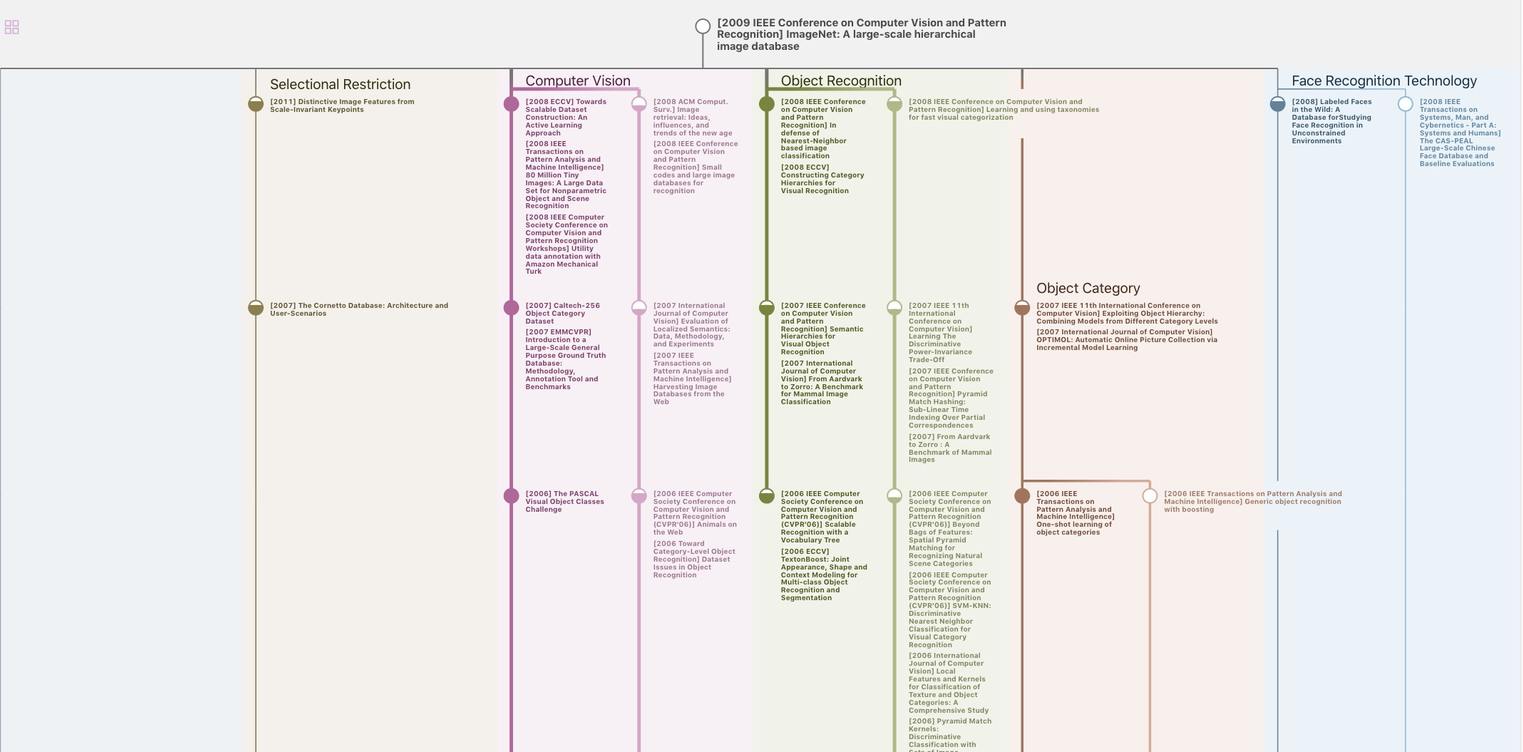
生成溯源树,研究论文发展脉络
Chat Paper
正在生成论文摘要