Prediction of thermo-mechanical properties of PCB conductive layers using convolutional neural networks
2023 24th International Conference on Thermal, Mechanical and Multi-Physics Simulation and Experiments in Microelectronics and Microsystems (EuroSimE)(2023)
摘要
Deriving the effective material properties of printed circuit board (PCB) layers is a particular challenge due to the intricate patterns of the conductive artwork. In this paper, we develop a convolutional neural network model to predict the effective orthotropic thermo-mechanical properties of the copper conductive layers of PCBs. To ensure the necessary level of detail for the material modeling, the images of the copper-resin distributions are extracted from the real electronic design files as patches of preselected pixel sizes. For each image, the corresponding homogenized material properties are computed using finite element analyses. After the training process on the formed dataset is completed, the developed model is validated using data unseen during training. The obtained results indicate that the considered approach is suitable for the prediction of the effective orthotropic properties of copper-resin patterns, and moreover, is promising for facilitating present-day time-to-market objectives in the industry.
更多查看译文
AI 理解论文
溯源树
样例
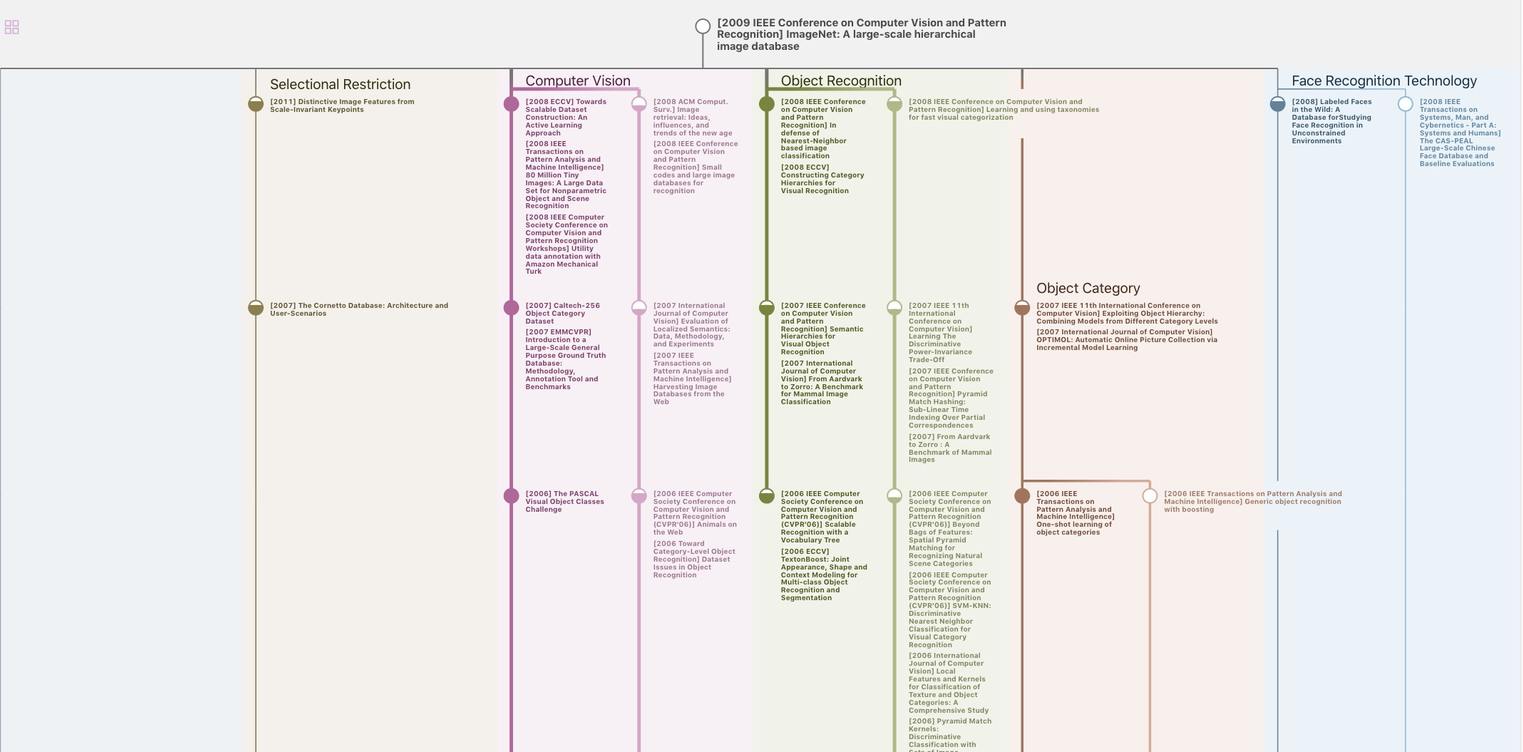
生成溯源树,研究论文发展脉络
Chat Paper
正在生成论文摘要