A grouping-based AdaBoost method for factor investing
2022 IEEE 34th International Conference on Tools with Artificial Intelligence (ICTAI)(2022)
摘要
Constructing a quantitative factor investment strategy based on hundreds of candidate factors is a critical challenge. Existing linear models do not account for nonlinearities and variable interactions, while complex machine learning models are easily overfitting. In this paper, motivated by the portfolio sorts methods in empirical asset pricing, we propose an alternative approach called grouping-based AdaBoost by adapting the existing AdaBoost. It introduces the experience of the financial field into the algorithm design to improve the performance and generalization of machine learning-based factor investing strategies. The proposed method restricts the factor to only predict the common part of the returns of the same groups and allows the potential nonlinear relationship between a factor and the return. Moreover, to enhance the model's ability to use factors with high correlation, we extend the single-grouping AdaBoost in a multi-grouping way. Experiments on the Chinese A-share market demonstrate the effectiveness of our approach in both stock performance classification and portfolio selection and provide intuitive evidence for the generalization of the proposed method.
更多查看译文
关键词
Factor Investing,Machine Learning,AdaBoost,Fintech
AI 理解论文
溯源树
样例
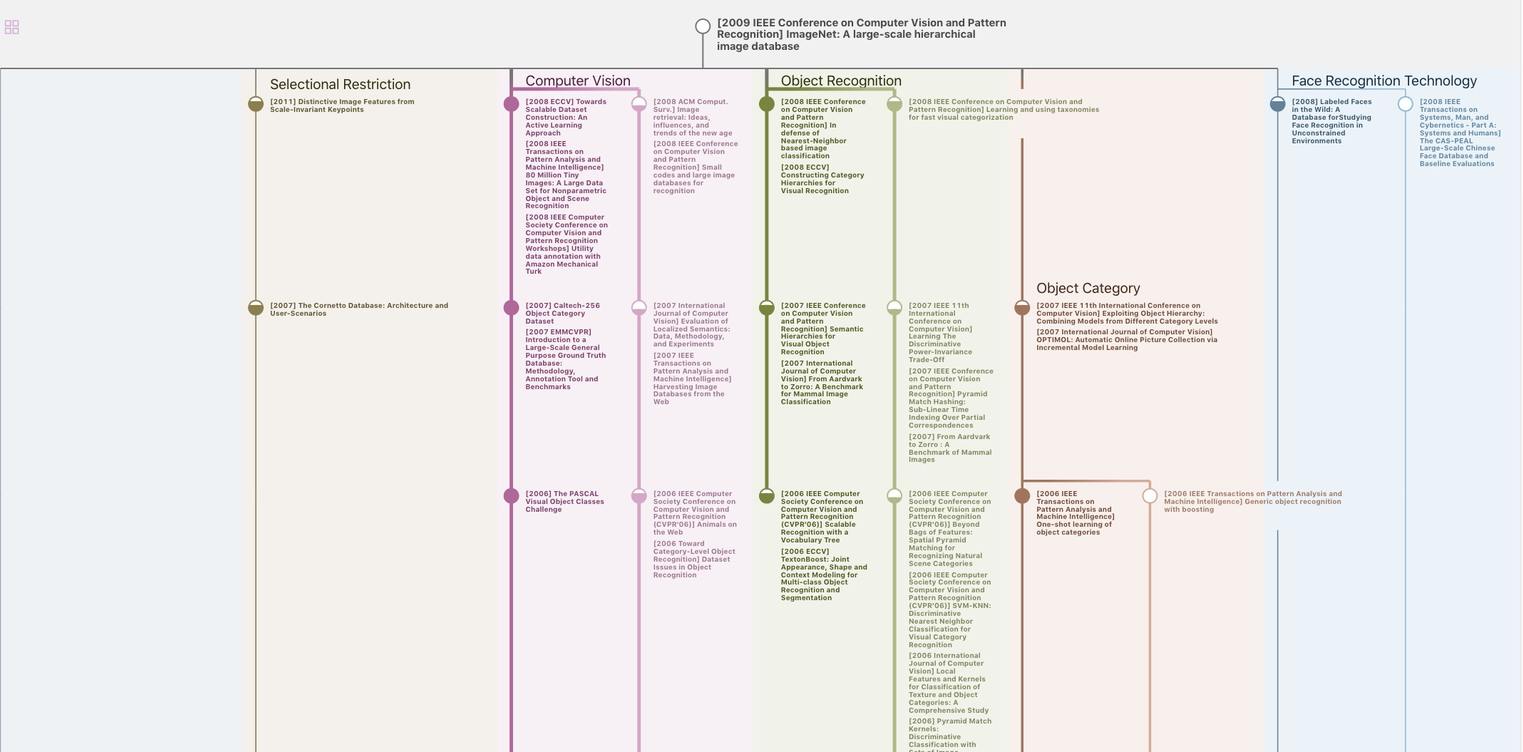
生成溯源树,研究论文发展脉络
Chat Paper
正在生成论文摘要