Knowledge Graph Completion by Multi-Channel Translating Embeddings
2022 IEEE 34TH INTERNATIONAL CONFERENCE ON TOOLS WITH ARTIFICIAL INTELLIGENCE, ICTAI(2022)
摘要
Knowledge graph completion (KGC) aims to perform link prediction to fill lost relations between entities by knowledge graph embedding (KGE). Translating embedding, as an efficient embedding method in KGE, is widely applied in numerous recent KGC models. However, these translating models may lack the ability to express various relation patterns and mapping properties for knowledge graphs (KGs). In this paper, a simple and well-performed translating model named TransC is proposed to express different relations. A multi-channel mechanism is defined firstly to constrain translating embeddings. Then a relation-aware transfer function is designed to break the expressive restriction and map triplets involving the same relation into a corresponding plane. We also mathematically prove that TransC is capable of expressing four popular relation patterns and all mapping properties. Finally, experimental results illustrate that TransC can efficiently represent the different relation patterns and properties and achieve better performance than state-of-the-art translating models.
更多查看译文
关键词
Knowledge Graph Completion,Link Prediction,Translating Embedding
AI 理解论文
溯源树
样例
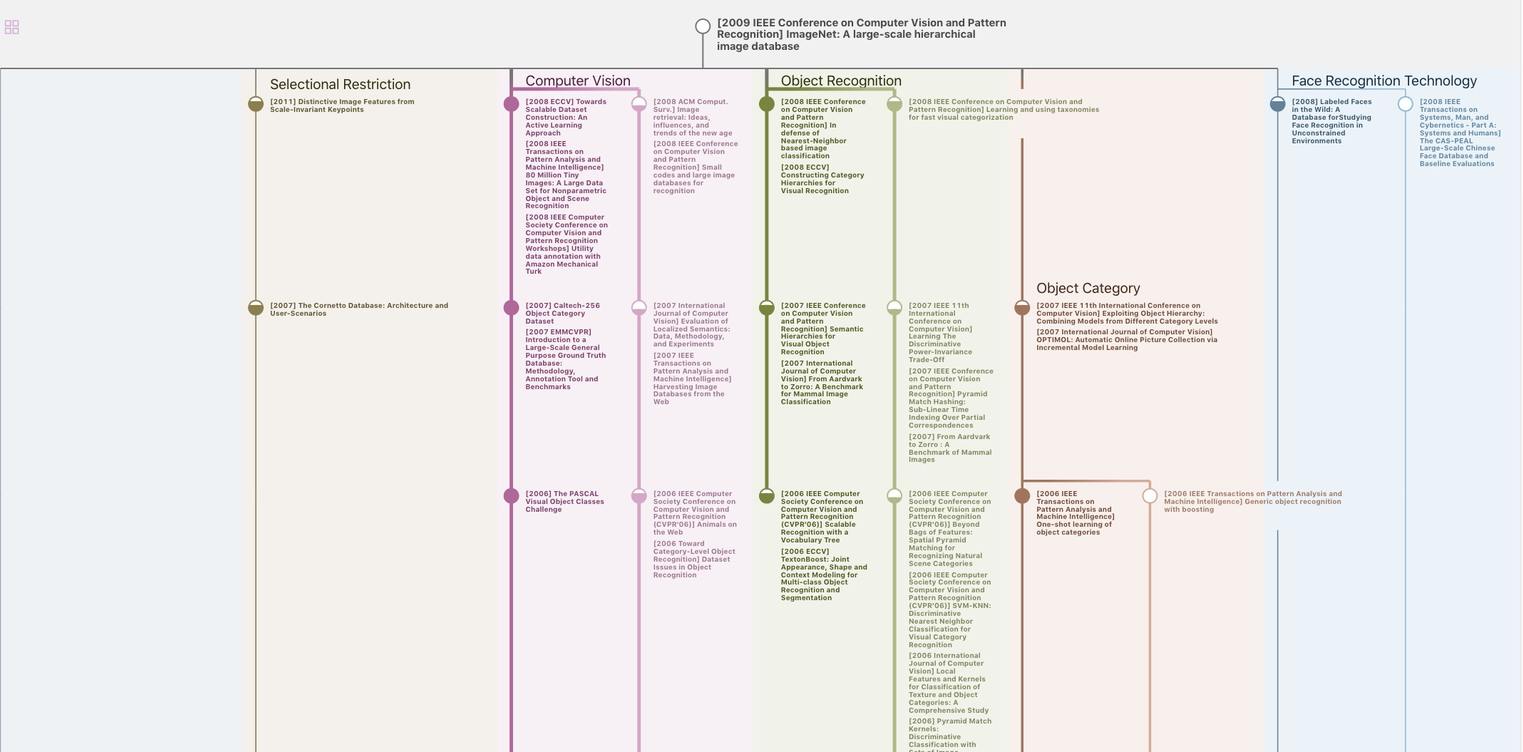
生成溯源树,研究论文发展脉络
Chat Paper
正在生成论文摘要