A Semi-supervised Learning Method for Electricity Theft Detection Based on CT-GAN
2022 IEEE International Conference on Power Systems and Electrical Technology (PSET)(2022)
摘要
Nowadays, energy theft has been a major problem worldwide. Most existing machine learning algorithms require large amounts of labeled data to train and build detection models, but the cost of acquiring labeled data is very high. In this paper, a semi-supervised learning method for electricity theft detection based on cooperative training generative adversarial network (CT-GAN) is proposed to avoid the poor effect due to little labeled data. The method has two advantages. The first is semisupervised learning which can solve the defect that the deep convolutional network model is easy to overfit and leads to poor classification performance in the case of insufficient label samples, and the second is two discriminators for co-training, which eliminates the problem of large distribution error of single discriminator and improves the training of semi-supervised generative adversarial networks stability, the ability of GAN to generate labeled sample data is also improved. A large number of experiments validate the effectiveness of our method.
更多查看译文
关键词
Electricity theft,machine learning,Generative Adversarial Networks (GAN),semi-supervised learning,discriminator
AI 理解论文
溯源树
样例
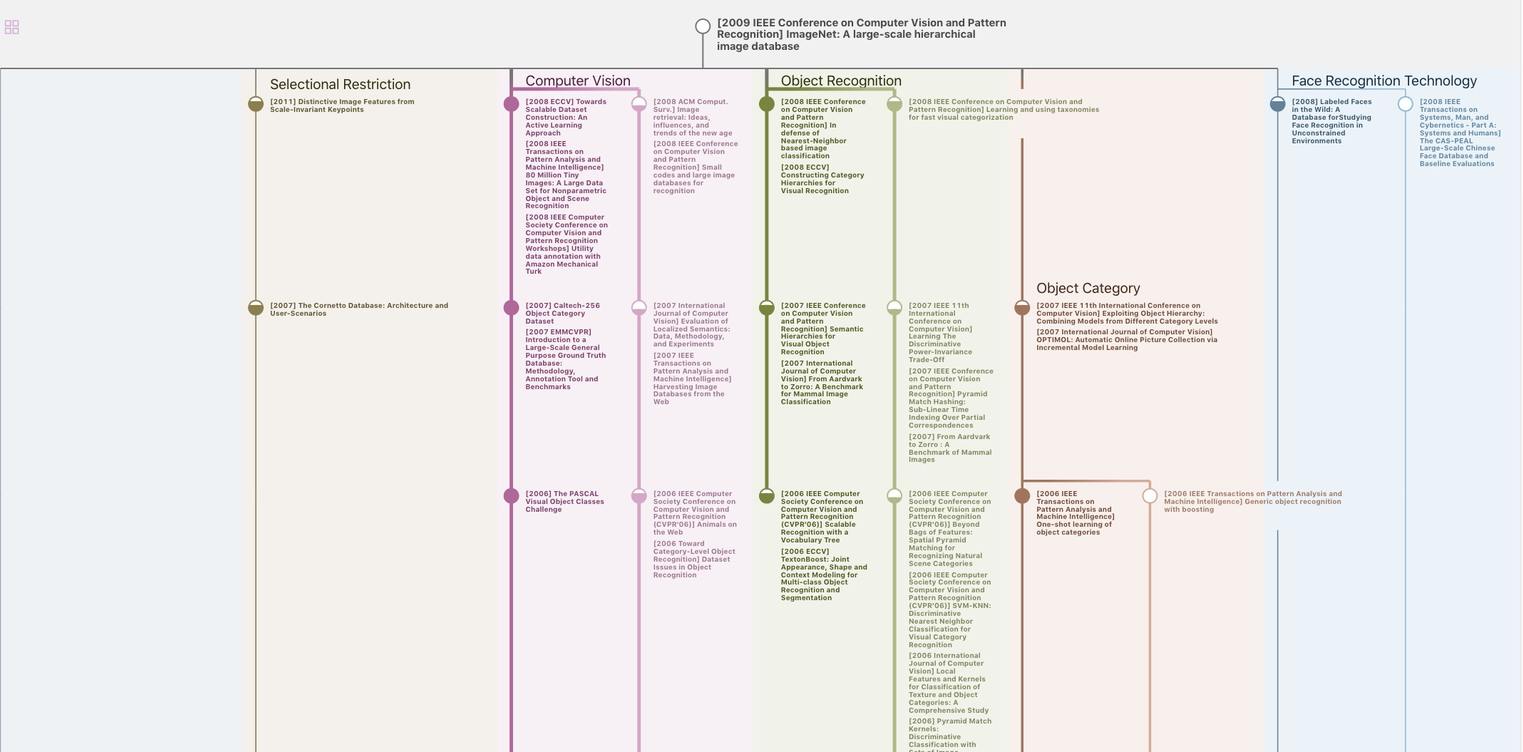
生成溯源树,研究论文发展脉络
Chat Paper
正在生成论文摘要