AdapterGNN: Efficient Delta Tuning Improves Generalization Ability in Graph Neural Networks
CoRR(2023)
摘要
Fine-tuning pre-trained models has recently yielded remarkable performance gains in graph neural networks (GNNs). In addition to pre-training techniques, inspired by the latest work in the natural language fields, more recent work has shifted towards applying effective fine-tuning approaches, such as parameter-efficient tuning (delta tuning). However, given the substantial differences between GNNs and transformer-based models, applying such approaches directly to GNNs proved to be less effective. In this paper, we present a comprehensive comparison of delta tuning techniques for GNNs and propose a novel delta tuning method specifically designed for GNNs, called AdapterGNN. AdapterGNN preserves the knowledge of the large pre-trained model and leverages highly expressive adapters for GNNs, which can adapt to downstream tasks effectively with only a few parameters, while also improving the model's generalization ability on the downstream tasks. Extensive experiments show that AdapterGNN achieves higher evaluation performance (outperforming full fine-tuning by 1.4% and 5.5% in the chemistry and biology domains respectively, with only 5% of its parameters tuned) and lower generalization gaps compared to full fine-tuning. Moreover, we empirically show that a larger GNN model can have a worse generalization ability, which differs from the trend observed in large language models. We have also provided a theoretical justification for delta tuning can improve the generalization ability of GNNs by applying generalization bounds.
更多查看译文
关键词
graph neural networks,efficient delta tuning,neural networks
AI 理解论文
溯源树
样例
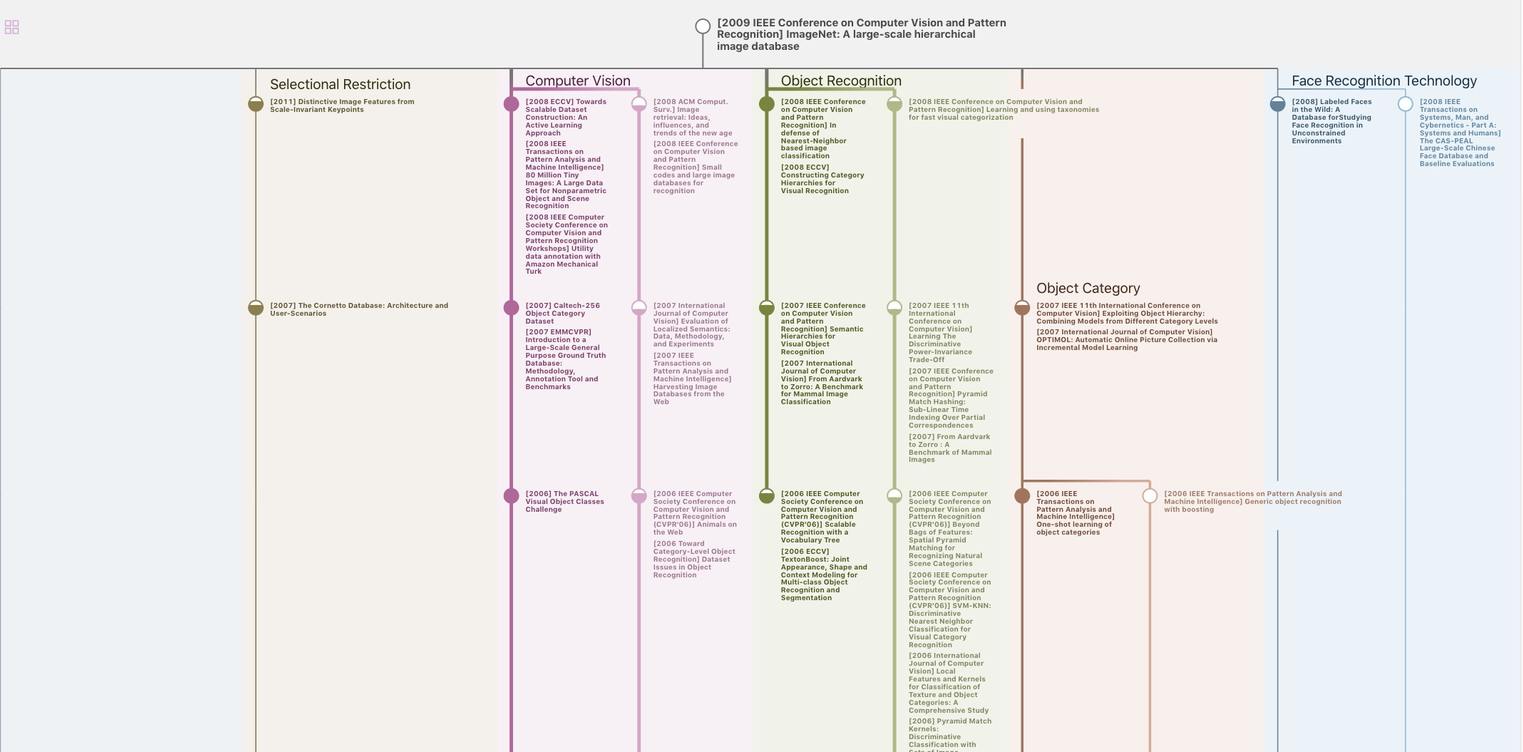
生成溯源树,研究论文发展脉络
Chat Paper
正在生成论文摘要