An Integrated vMF Embedding and VOS Framework for Robust Pedestrian Re-Identification and Out-of-Distribution Detection
crossref(2023)
摘要
In recent years, pedestrian re-identification has gained a lot of interest due to its importance for many purposes such as security and safety. Many types of solutions have been proposed to solve this problem, where the majority are based on a features extraction such Convolution Neural Networks (CNNs). These approaches assume that only the identities that are in the training data can be recognized. The pedestrians in the training data are called In distribution (ID). However, in real world scenarios, new pedestrians and objects can appear in the scene and the model should detect them as Out Of Distribution (OOD). In our previous study , we proposed a pedestrian re-identification based on von-Mises Fisher (vMF) distribution. Each identity is embedded in the unit sphere as a compact vMF distribution far from other identities distributions. The embedding is done through a base CNN. Recently, proposed a framework called Virtual Outlier Synthetic (VOS), that detects OOD based on synthesising virtual outlier in the embedding space in an online manner. Their approach assumes that the samples from the same object maps to a compact space. This assumption aligns with the vMF based approach. Therefore, in this paper, we revisited the vMF approach and merged with VOS in order to detect OOD data points. We present our approach to merge both frameworks. We conducted several experiments to evaluate our proposed framework. Results showed that our framework was able to detect new pedestrian that do not exist in the training data in the inference phase. It also slightly helped to improve the re-identification performance.
更多查看译文
AI 理解论文
溯源树
样例
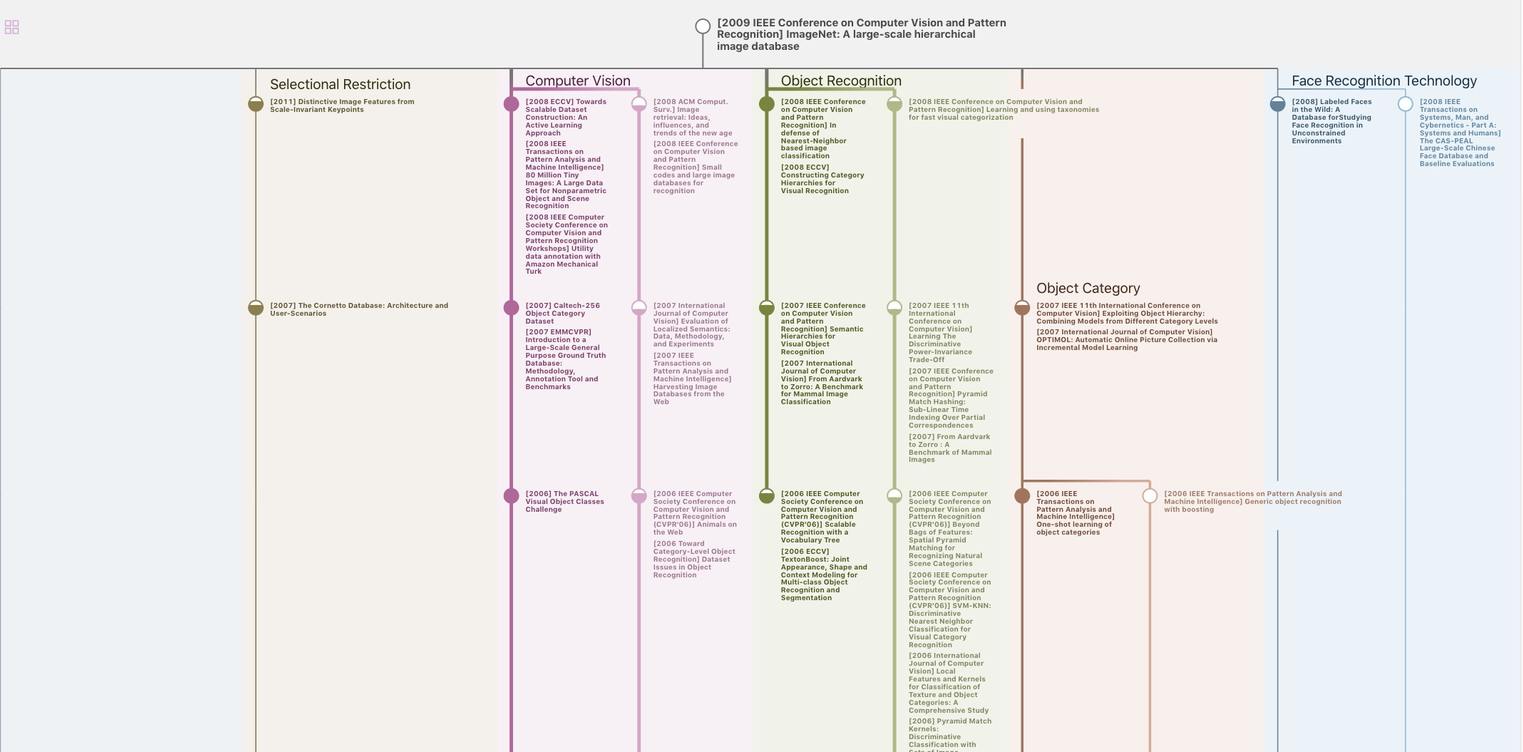
生成溯源树,研究论文发展脉络
Chat Paper
正在生成论文摘要