Diversifying the High-level Features for better Adversarial Transferability
CoRR(2023)
摘要
Given the great threat of adversarial attacks against Deep Neural Networks (DNNs), numerous works have been proposed to boost transferability to attack real-world applications. However, existing attacks often utilize advanced gradient calculation or input transformation but ignore the white-box model. Inspired by the fact that DNNs are over-parameterized for superior performance, we propose diversifying the high-level features (DHF) for more transferable adversarial examples. In particular, DHF perturbs the high-level features by randomly transforming the high-level features and mixing them with the feature of benign samples when calculating the gradient at each iteration. Due to the redundancy of parameters, such transformation does not affect the classification performance but helps identify the invariant features across different models, leading to much better transferability. Empirical evaluations on ImageNet dataset show that DHF could effectively improve the transferability of existing momentum-based attacks. Incorporated into the input transformation-based attacks, DHF generates more transferable adversarial examples and outperforms the baselines with a clear margin when attacking several defense models, showing its generalization to various attacks and high effectiveness for boosting transferability.
更多查看译文
关键词
better adversarial
AI 理解论文
溯源树
样例
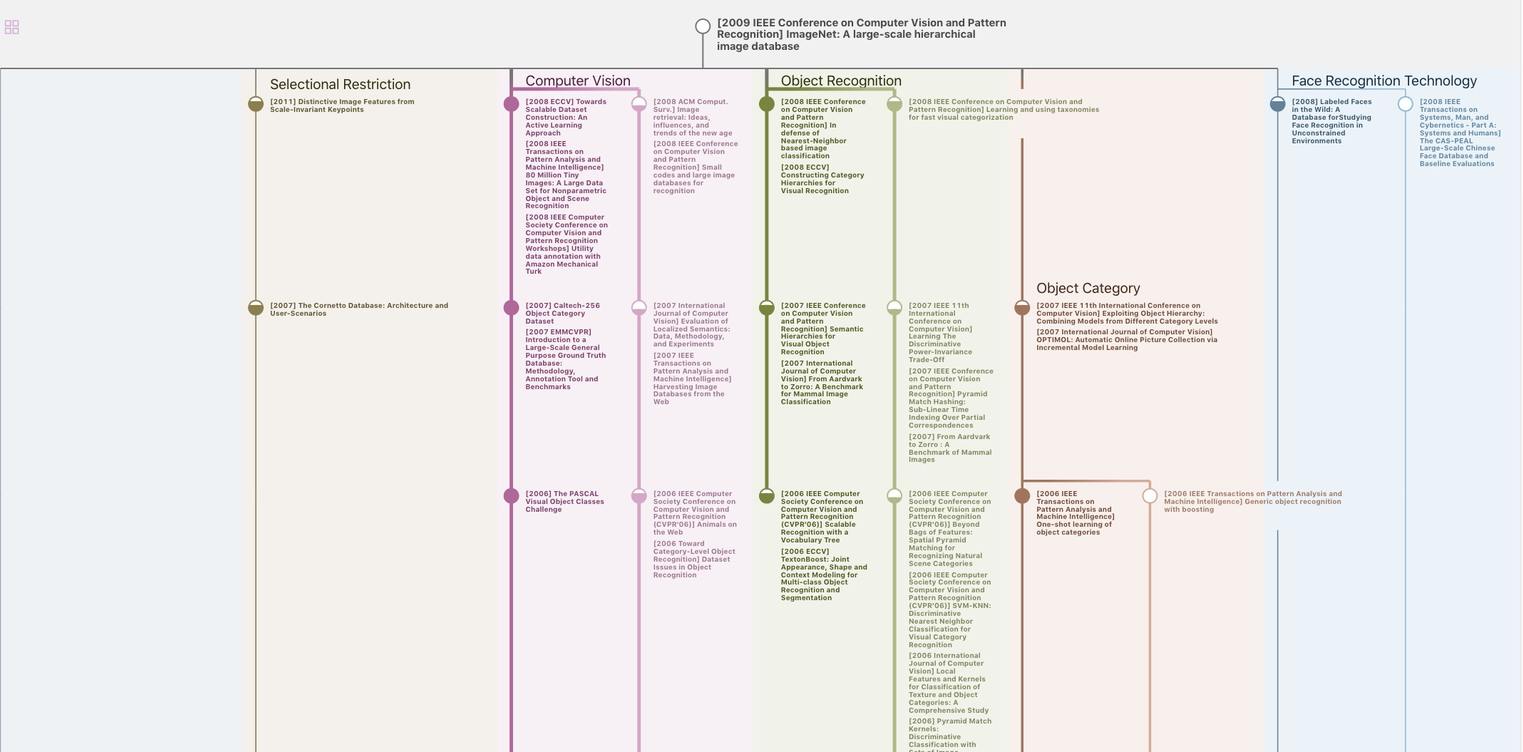
生成溯源树,研究论文发展脉络
Chat Paper
正在生成论文摘要