Filter-Aware Model-Predictive Control
CoRR(2023)
摘要
Partially-observable problems pose a trade-off between reducing costs and gathering information. They can be solved optimally by planning in belief space, but that is often prohibitively expensive. Model-predictive control (MPC) takes the alternative approach of using a state estimator to form a belief over the state, and then plan in state space. This ignores potential future observations during planning and, as a result, cannot actively increase or preserve the certainty of its own state estimate. We find a middle-ground between planning in belief space and completely ignoring its dynamics by only reasoning about its future accuracy. Our approach, filter-aware MPC, penalises the loss of information by what we call "trackability", the expected error of the state estimator. We show that model-based simulation allows condensing trackability into a neural network, which allows fast planning. In experiments involving visual navigation, realistic every-day environments and a two-link robot arm, we show that filter-aware MPC vastly improves regular MPC.
更多查看译文
关键词
control,filter-aware,model-predictive
AI 理解论文
溯源树
样例
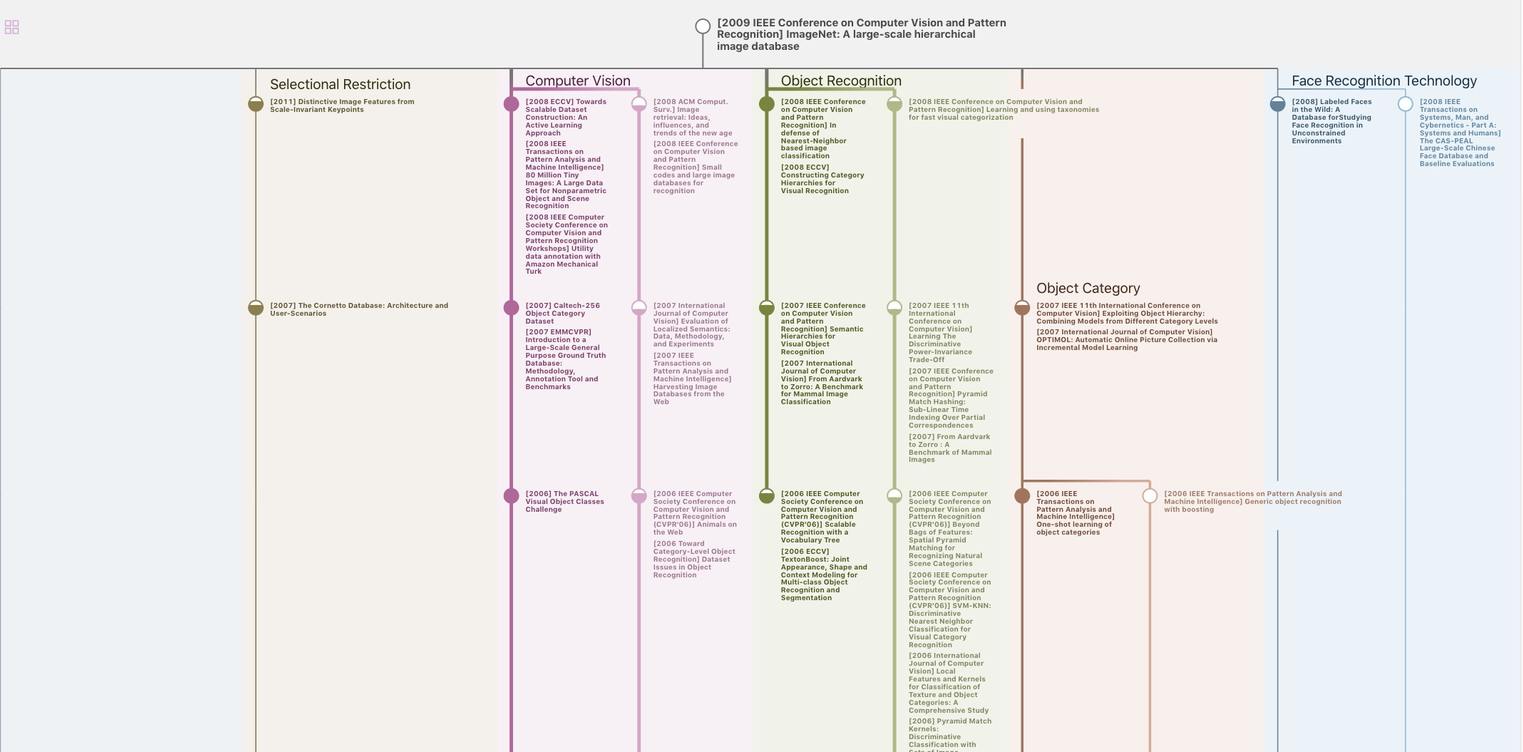
生成溯源树,研究论文发展脉络
Chat Paper
正在生成论文摘要