Search-Map-Search: A Frame Selection Paradigm for Action Recognition
CVPR 2023(2023)
摘要
Despite the success of deep learning in video understanding tasks, processing every frame in a video is computationally expensive and often unnecessary in real-time applications. Frame selection aims to extract the most informative and representative frames to help a model better understand video content. Existing frame selection methods either individually sample frames based on per-frame importance prediction, without considering interaction among frames, or adopt reinforcement learning agents to find representative frames in succession, which are costly to train and may lead to potential stability issues. To overcome the limitations of existing methods, we propose a Search-Map-Search learning paradigm which combines the advantages of heuristic search and supervised learning to select the best combination of frames from a video as one entity. By combining search with learning, the proposed method can better capture frame interactions while incurring a low inference overhead. Specifically, we first propose a hierarchical search method conducted on each training video to search for the optimal combination of frames with the lowest error on the downstream task. A feature mapping function is then learned to map the frames of a video to the representation of its target optimal frame combination. During inference, another search is performed on an unseen video to select a combination of frames whose feature representation is close to the projected feature representation. Extensive experiments based on several action recognition benchmarks demonstrate that our frame selection method effectively improves performance of action recognition models, and significantly outperforms a number of competitive baselines.
更多查看译文
AI 理解论文
溯源树
样例
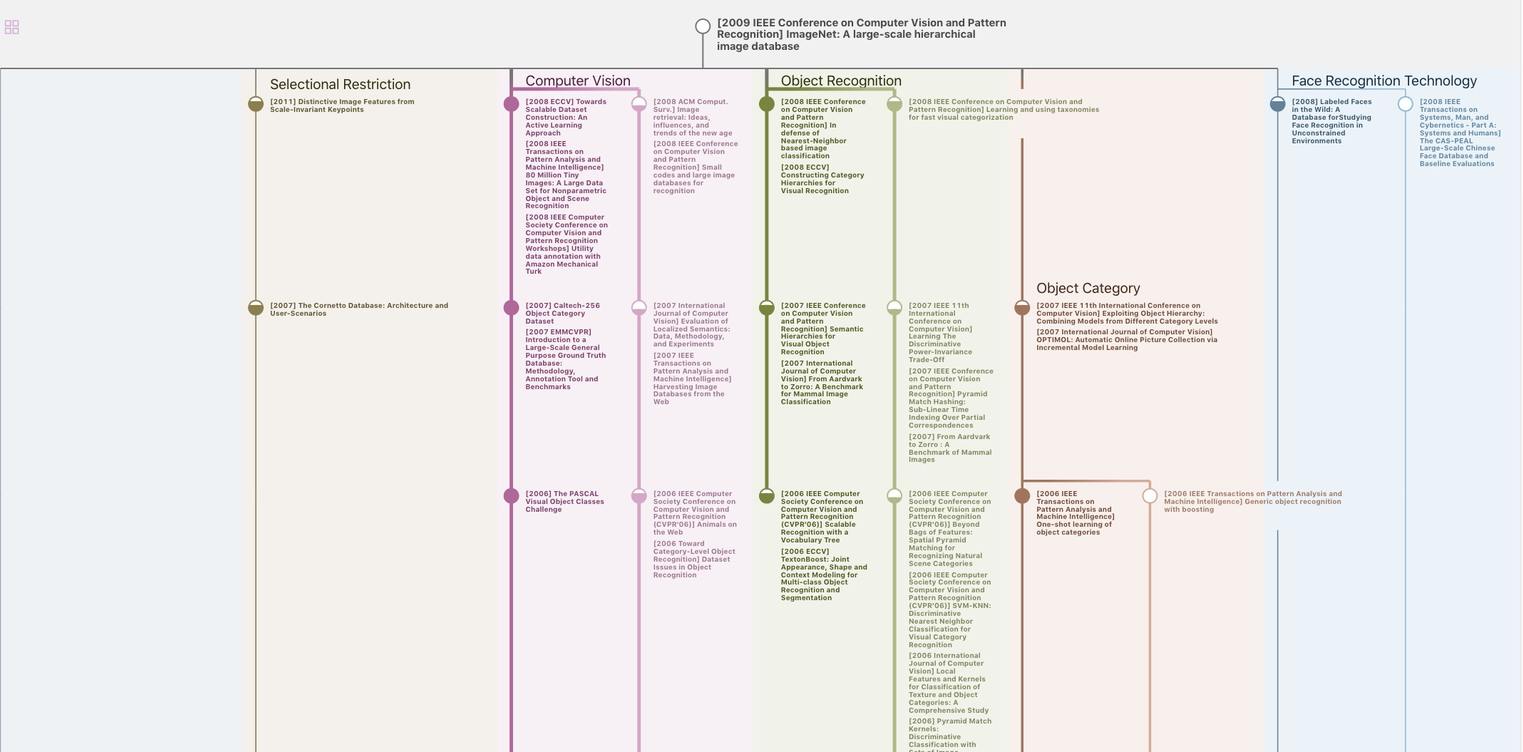
生成溯源树,研究论文发展脉络
Chat Paper
正在生成论文摘要