Data lake-driven analytics identify nocturnal non-dipping of heart rate as predictor of unfavorable stroke outcome at discharge
Journal of neurology(2023)
摘要
Background Post-stroke heart rate (HR) and heart rate variability (HRV) changes have been proposed as outcome predictors after stroke. We used data lake-enabled continuous electrocardiograms to assess post-stroke HR and HRV, and to determine the utility of HR and HRV to improve machine learning-based predictions of stroke outcome. Methods In this observational cohort study, we included stroke patients admitted to two stroke units in Berlin, Germany, between October 2020 and December 2021 with final diagnosis of acute ischemic stroke or acute intracranial hemorrhage and collected continuous ECG data through data warehousing. We created circadian profiles of several continuously recorded ECG parameters including HR and HRV parameters. The pre-defined primary outcome was short-term unfavorable functional outcome after stroke indicated through modified Rankin Scale (mRS) score of > 2. Results We included 625 stroke patients, 287 stroke patients remained after matching for age and National Institute of Health Stroke Scale (NIHSS; mean age 74.5 years, 45.6% female, 88.9% ischemic, median NIHSS 5). Both higher HR and nocturnal non-dipping of HR were associated with unfavorable functional outcome ( p < 0.01). The examined HRV parameters were not associated with the outcome of interest. Nocturnal non-dipping of HR ranked highly in feature importance of various machine learning models. Conclusions Our data suggest that a lack of circadian HR modulation, specifically nocturnal non-dipping, is associated with short-term unfavorable functional outcome after stroke, and that including HR into machine learning-based prediction models may lead to improved stroke outcome prediction.
更多查看译文
关键词
Data warehouse,Heart rate,Heart rate variability,Machine learning,Nocturnal non-dipping,Stroke outcome prediction
AI 理解论文
溯源树
样例
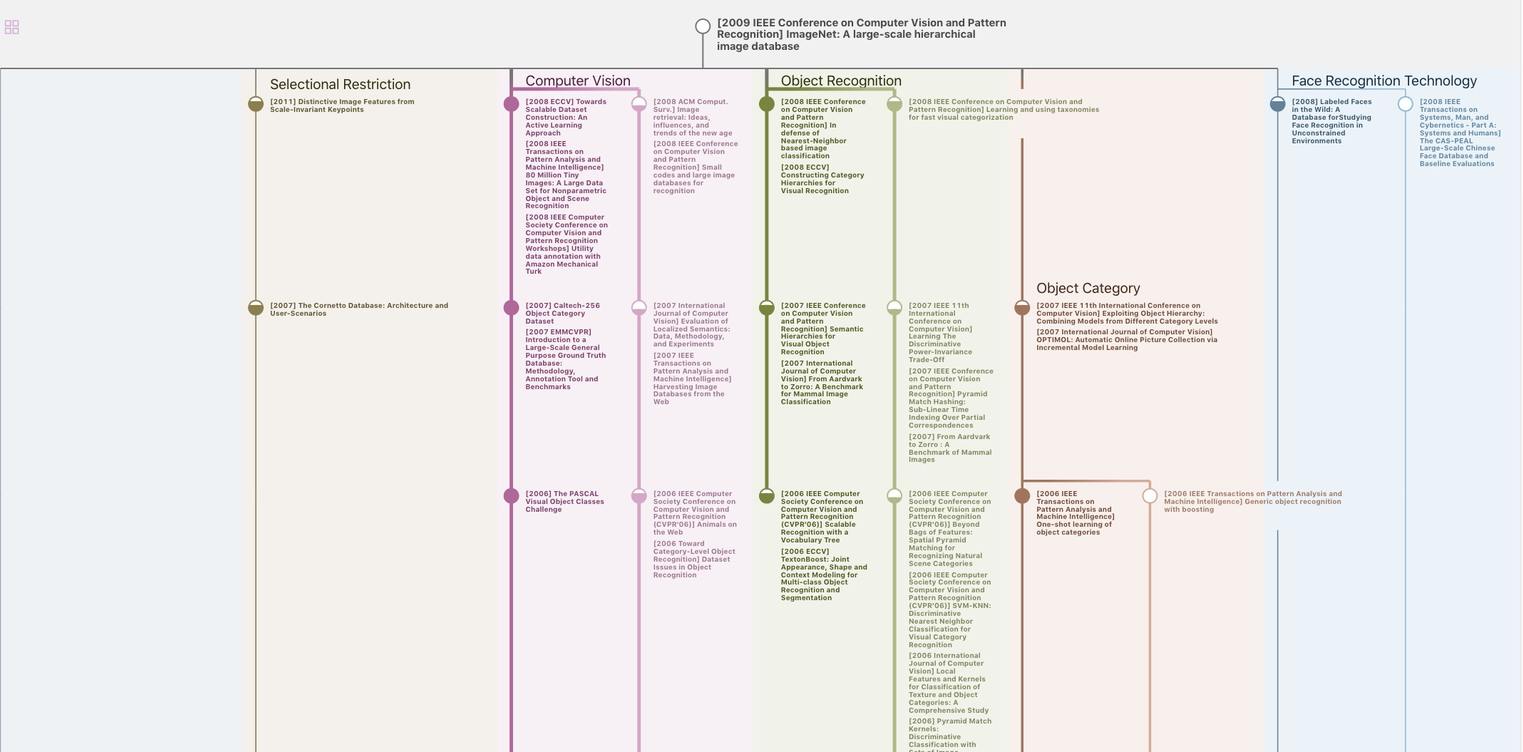
生成溯源树,研究论文发展脉络
Chat Paper
正在生成论文摘要