Predicting one-year left ventricular mass index regression following transcatheter aortic valve replacement in patients with severe aortic stenosis: A new era is coming.
Frontiers in cardiovascular medicine(2023)
摘要
Aortic stenosis (AS) is the most common valvular heart disease in the western world, particularly worrisome with an ever-aging population wherein postoperative outcome for aortic valve replacement is strongly related to the timing of surgery in the natural course of disease. Yet, guidelines for therapy planning overlook insightful, quantified measures from medical imaging to educate clinical decisions. Herein, we leverage statistical shape analysis (SSA) techniques combined with customized machine learning methods to extract latent information from segmented left ventricle (LV) shapes. This enabled us to predict left ventricular mass index (LVMI) regression a year after transcatheter aortic valve replacement (TAVR). LVMI regression is an expected phenomena in patients undergone aortic valve replacement reported to be tightly correlated with survival one and five year after the intervention. In brief, LV geometries were extracted from medical images of a cohort of AS patients using deep learning tools, and then analyzed to create a set of statistical shape models (SSMs). Then, the supervised shape features were extracted to feed a support vector regression (SVR) model to predict the LVMI regression. The average accuracy of the predictions was validated against clinical measurements calculating root mean square error and score which yielded the satisfactory values of 0.28 and 0.67, respectively, on test data. Our work reveals the promising capability of advanced mathematical and bioinformatics approaches such as SSA and machine learning to improve medical output prediction and treatment planning.
更多查看译文
关键词
Structural heart disease,TAVR surgery,left ventricle remodeling,machine learning,statistical shape analysis
AI 理解论文
溯源树
样例
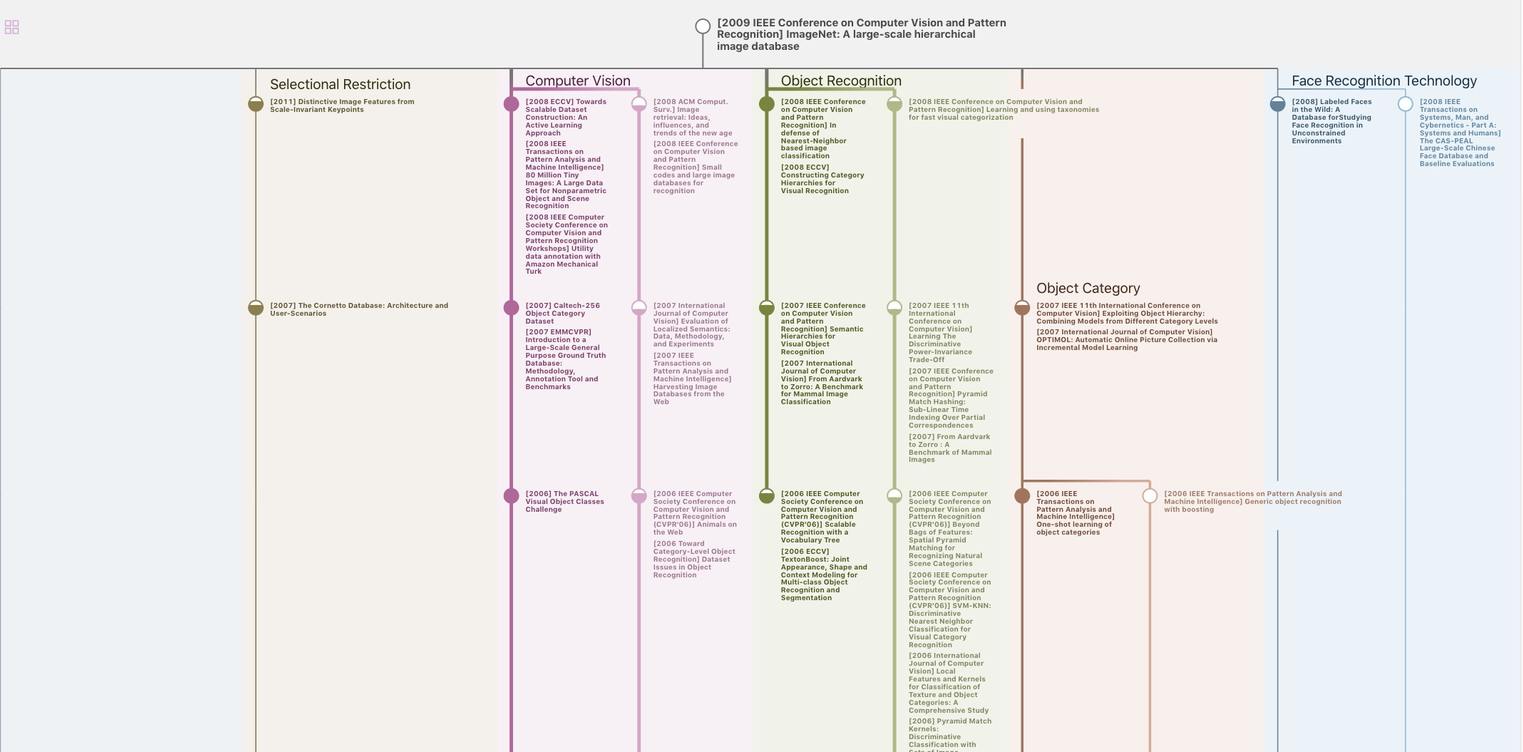
生成溯源树,研究论文发展脉络
Chat Paper
正在生成论文摘要