Optimal Weight Models for Ferroelectric Synapses Toward Neuromorphic Computing
IEEE Transactions on Electron Devices(2023)
摘要
Ferroelectric field effect transistors (FeFETs) as artificial synapses have attracted great interests in neuromorphic computing. To enable the applications, the electrical response of FeFETs is required to map into the neural network via a weight model. However, the mapping strategy is less studied. Herein, we put forth an alternative symmetric nonlinearity model (S-N model) to map the symmetric conductance response of FeFETs. Based on the finely tuned metal-ferroelectric-metal-insulator-semiconductor (MFMIS) structures, FeFETs achieve nearly 7-bit states with
${G}_{\text {max}}/{G}_{\text {min}}$
over
$10^{{3}}$
when applied with 40-ns-width incremental amplitude pulses. By invoking the proposed S-N model, global states of raw conductance are fully accessed without any loss. Comparing with the conventional asymmetric nonlinearity model (A-N model), the optimized mapping method helps deep neural network (DNN) to implement a higher recognition accuracy of 94.1% (with cycle-to-cycle variation of
$\sim $
1.79%). These results offer a modeling perspective for promoting the realization of FeFETs-based high-density and efficient neuromorphic systems.
更多查看译文
关键词
Ferroelectric synaptic transistor,metal-ferroelectric-metal-insulator-semiconductor (MFMIS),neuromorphic computing,symmetric nonlinearity model,weight model
AI 理解论文
溯源树
样例
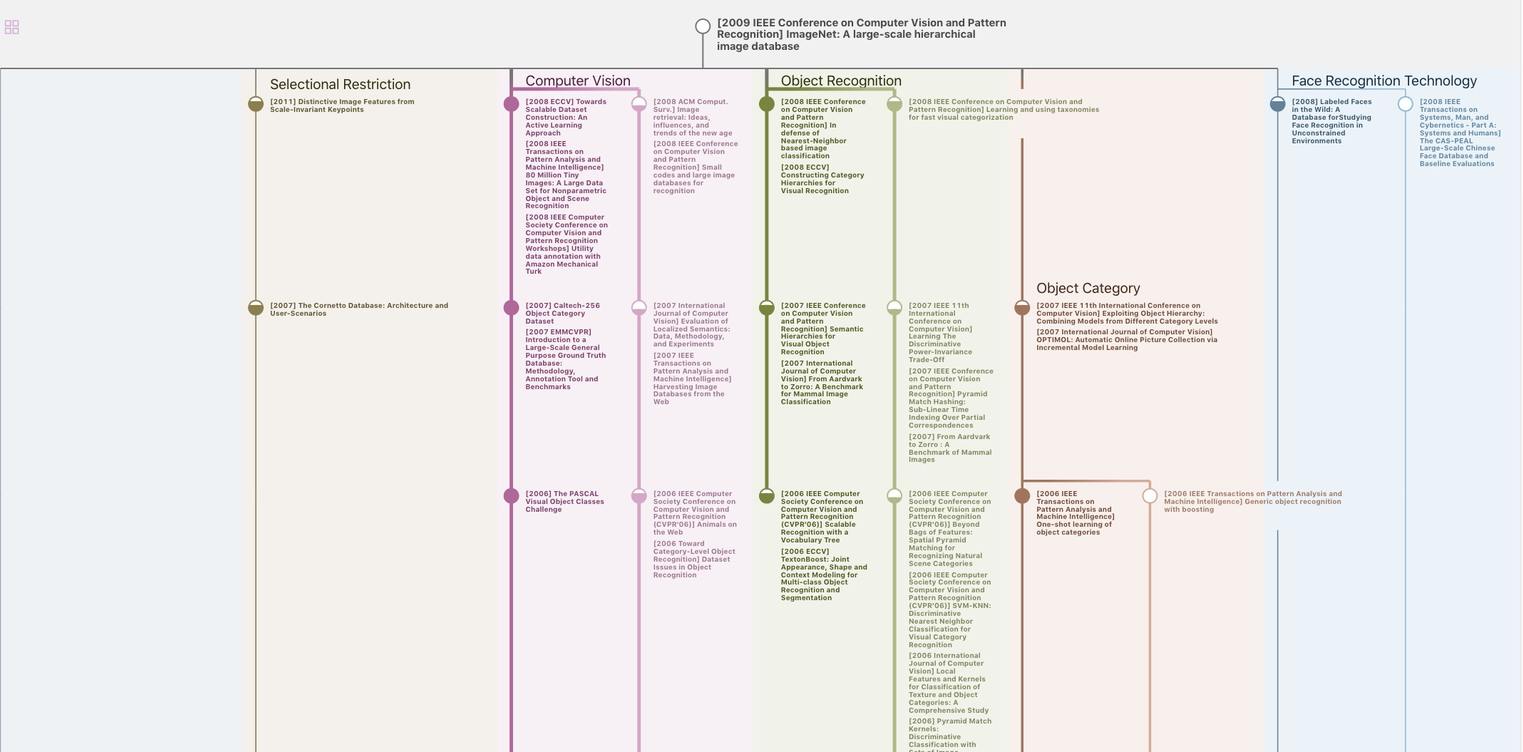
生成溯源树,研究论文发展脉络
Chat Paper
正在生成论文摘要