Diagnostic models predicting paediatric viral acute respiratory infections: a systematic review
BMJ open(2023)
摘要
Objectives To systematically review and evaluate diagnostic models used to predict viral acute respiratory infections (ARIs) in children. Design Systematic review. Data sources PubMed and Embase were searched from 1 January 1975 to 3 February 2022. Eligibility criteria We included diagnostic models predicting viral ARIs in children (<18 years) who sought medical attention from a healthcare setting and were written in English. Prediction model studies specific to SARS-CoV-2, COVID-19 or multisystem inflammatory syndrome in children were excluded. Data extraction and synthesis Study screening, data extraction and quality assessment were performed by two independent reviewers. Study characteristics, including population, methods and results, were extracted and evaluated for bias and applicability using the Checklist for Critical Appraisal and Data Extraction for Systematic Reviews of Prediction Modelling Studies and PROBAST (Prediction model Risk Of Bias Assessment Tool). Results Of 7049 unique studies screened, 196 underwent full text review and 18 were included. The most common outcome was viral-specific influenza (n=7; 58%). Internal validation was performed in 8 studies (44%), 10 studies (56%) reported discrimination measures, 4 studies (22%) reported calibration measures and none performed external validation. According to PROBAST, a high risk of bias was identified in the analytic aspects in all studies. However, the existing studies had minimal bias concerns related to the study populations, inclusion and modelling of predictors, and outcome ascertainment. Conclusions Diagnostic prediction can aid clinicians in aetiological diagnoses of viral ARIs. External validation should be performed on rigorously internally validated models with populations intended for model application.
更多查看译文
关键词
epidemiology,respiratory infections,virology
AI 理解论文
溯源树
样例
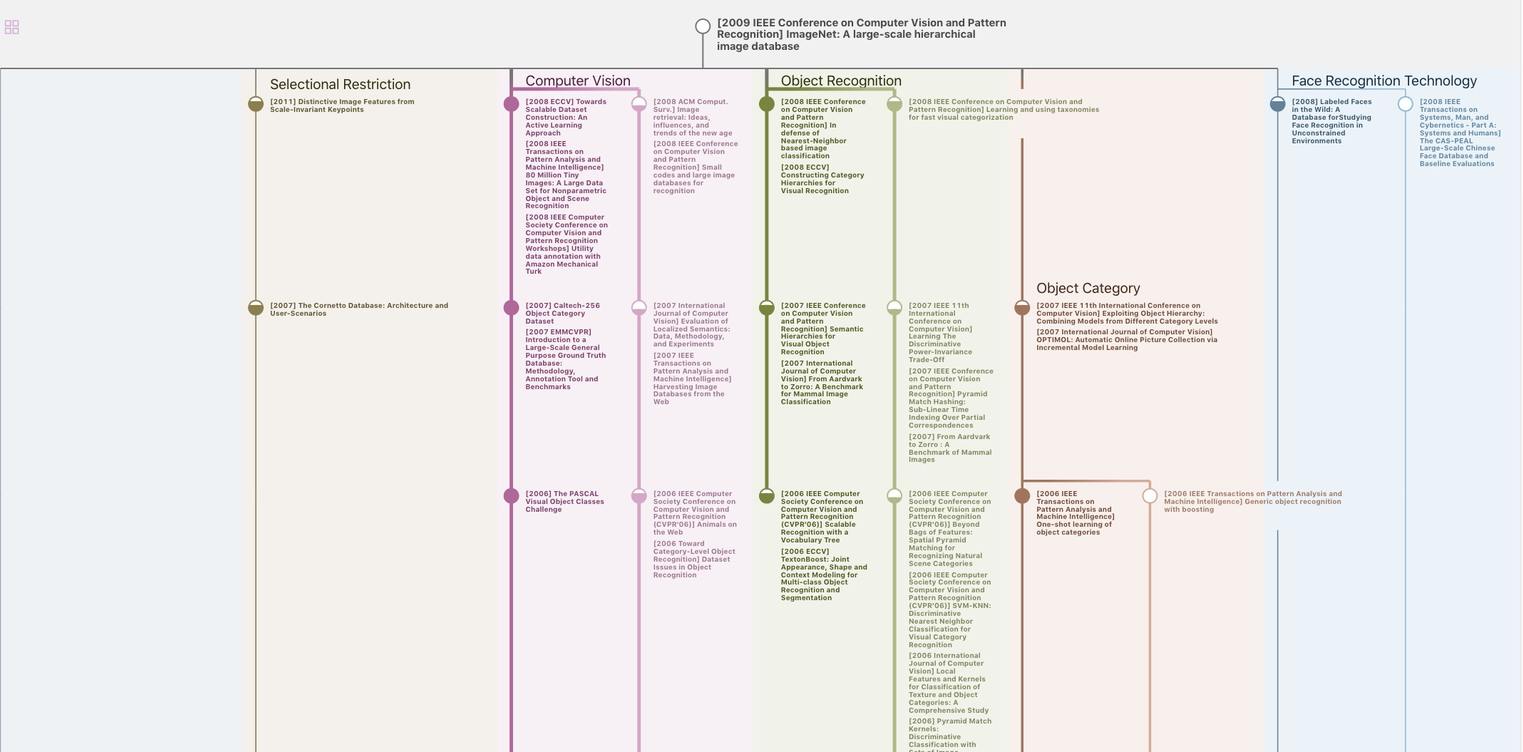
生成溯源树,研究论文发展脉络
Chat Paper
正在生成论文摘要