Spectral normalization and dual contrastive regularization for image-to-image translation
The Visual Computer(2024)
摘要
Existing image-to-image (I2I) translation methods achieve state-of-the-art performance by incorporating the patch-wise contrastive learning into generative adversarial networks. However, patch-wise contrastive learning only focuses on the local content similarity but neglects the global structure constraint, which affects the quality of the generated images. In this paper, we propose a new unpaired I2I translation framework based on dual contrastive regularization and spectral normalization, namely SN-DCR. To maintain consistency of the global structure and texture, we design the dual contrastive regularization using different deep feature spaces respectively. In order to improve the global structure information of the generated images, we formulate a semantic contrastive loss to make the global semantic structure of the generated images similar to the real images from the target domain in the semantic feature space. We use gram matrices to extract the style of texture from images. Similarly, we design a style contrastive loss to improve the global texture information of the generated images. Moreover, to enhance the stability of the model, we employ the spectral normalized convolutional network in the design of our generator. We conduct comprehensive experiments to evaluate the effectiveness of SN-DCR, and the results prove that our method achieves SOTA in multiple tasks. The code and pretrained models are available at https://github.com/zhihefang/SN-DCR .
更多查看译文
关键词
Image-to-image translation,Contrastive learning,Generative adversarial network
AI 理解论文
溯源树
样例
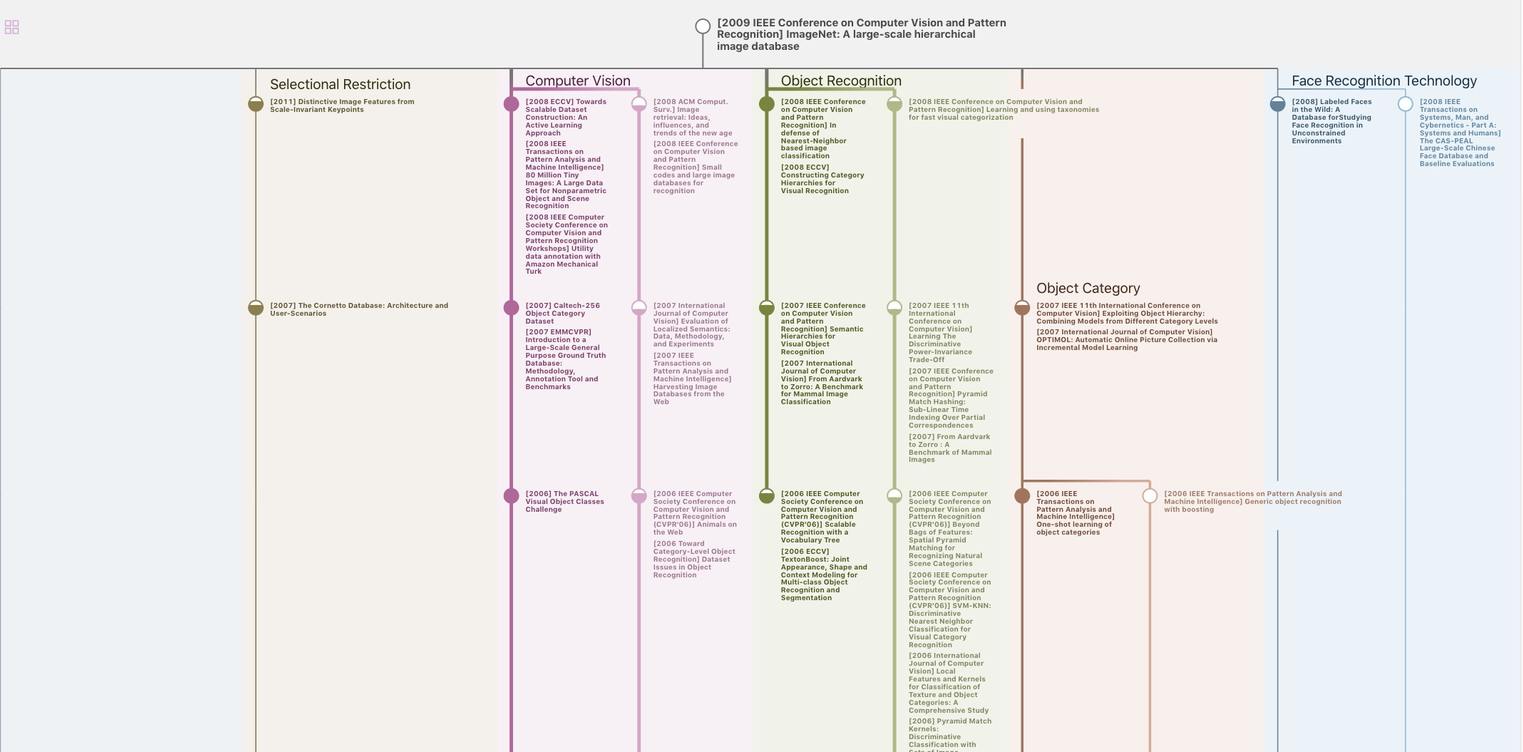
生成溯源树,研究论文发展脉络
Chat Paper
正在生成论文摘要