NaviNeRF: NeRF-based 3D Representation Disentanglement by Latent Semantic Navigation
Proceedings of the IEEE/CVF International Conference on Computer Vision (ICCV)(2023)
摘要
3D representation disentanglement aims to identify, decompose, and manipulate the underlying explanatory factors of 3D data, which helps AI fundamentally understand our 3D world. This task is currently under-explored and poses great challenges: (i) the 3D representations are complex and in general contains much more information than 2D image; (ii) many 3D representations are not well suited for gradient-based optimization, let alone disentanglement. To address these challenges, we use NeRF as a differentiable 3D representation, and introduce a self-supervised Navigation to identify interpretable semantic directions in the latent space. To our best knowledge, this novel method, dubbed NaviNeRF, is the first work to achieve fine-grained 3D disentanglement without any priors or supervision. Specifically, NaviNeRF is built upon the generative NeRF pipeline, and equipped with an Outer Navigation Branch and an Inner Refinement Branch. They are complementary ---- the outer navigation is to identify global-view semantic directions, and the inner refinement dedicates to fine-grained attributes. A synergistic loss is further devised to coordinate two branches. Extensive experiments demonstrate that NaviNeRF has a superior fine-grained 3D disentanglement ability than the previous 3D-aware models. Its performance is also comparable to editing-oriented models relying on semantic or geometry priors.
更多查看译文
AI 理解论文
溯源树
样例
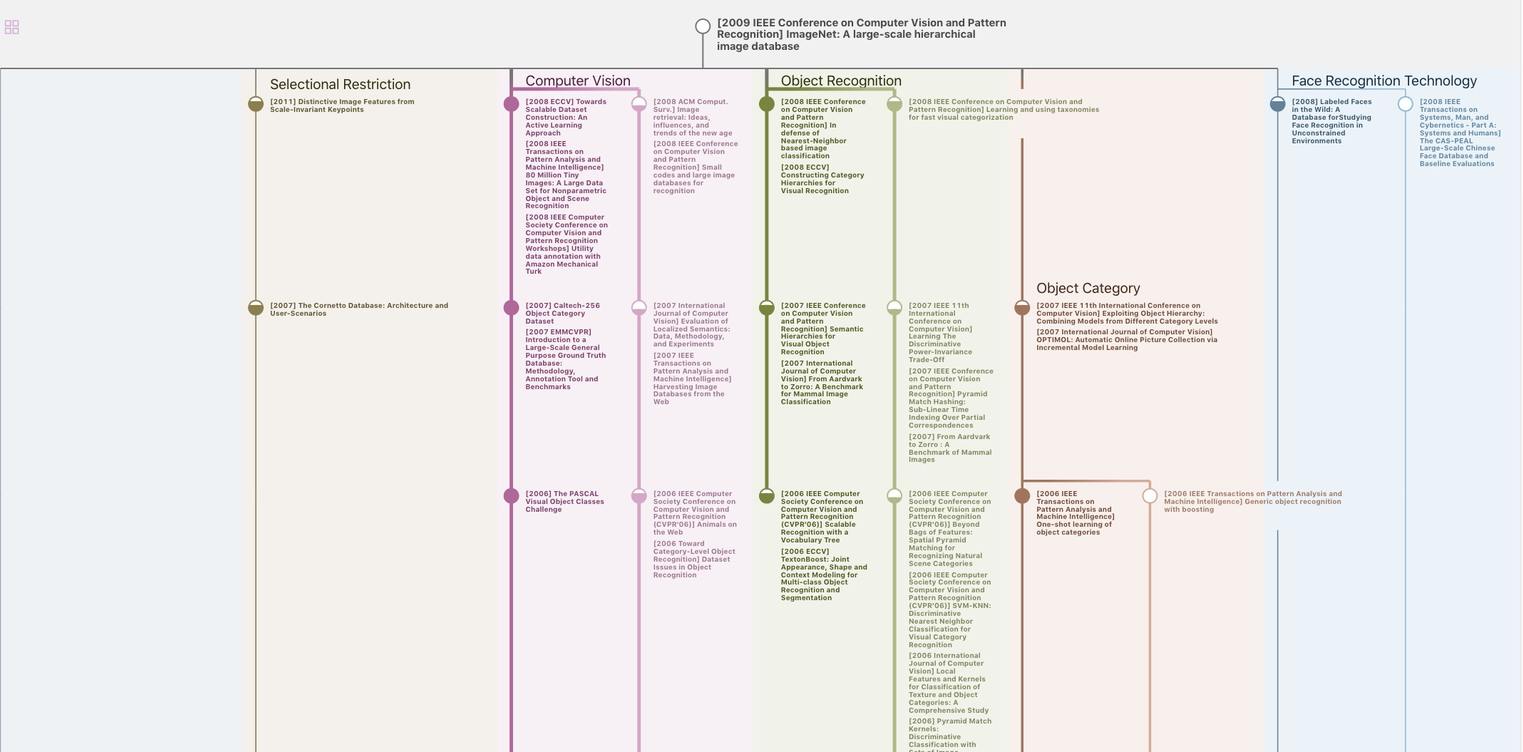
生成溯源树,研究论文发展脉络
Chat Paper
正在生成论文摘要