AC Power Flow Feasibility Restoration Via a State Estimation-Based Post-Processing Algorithm
ELECTRIC POWER SYSTEMS RESEARCH(2024)
摘要
This paper presents an algorithm for restoring AC power flow feasibility fromsolutions to simplified optimal power flow (OPF) problems, including convexrelaxations, power flow approximations, and machine learning (ML) models. Theproposed algorithm employs a state estimation-based post-processing techniquein which voltage phasors, power injections, and line flows from solutions torelaxed, approximated, or ML-based OPF problems are treated similarly to noisymeasurements in a state estimation algorithm. The algorithm leveragesinformation from various quantities to obtain feasible voltage phasors andpower injections that satisfy the AC power flow equations. Weight and biasparameters are computed offline using an adaptive stochastic gradient descentmethod. By automatically learning the trustworthiness of various outputs fromsimplified OPF problems, these parameters inform the online computations of thestate estimation-based algorithm to both recover feasible solutions andcharacterize the performance of power flow approximations, relaxations, and MLmodels. Furthermore, the proposed algorithm can simultaneously utilize combinedsolutions from different relaxations, approximations, and ML models to enhanceperformance. Case studies demonstrate the effectiveness and scalability of theproposed algorithm, with solutions that are both AC power flow feasible andmuch closer to the true AC OPF solutions than alternative methods, often byseveral orders of magnitude in the squared two-norm loss function.
更多查看译文
关键词
Optimal power flow (OPF),State estimation (SE),Operating point restoration,Machine learning (ML)
AI 理解论文
溯源树
样例
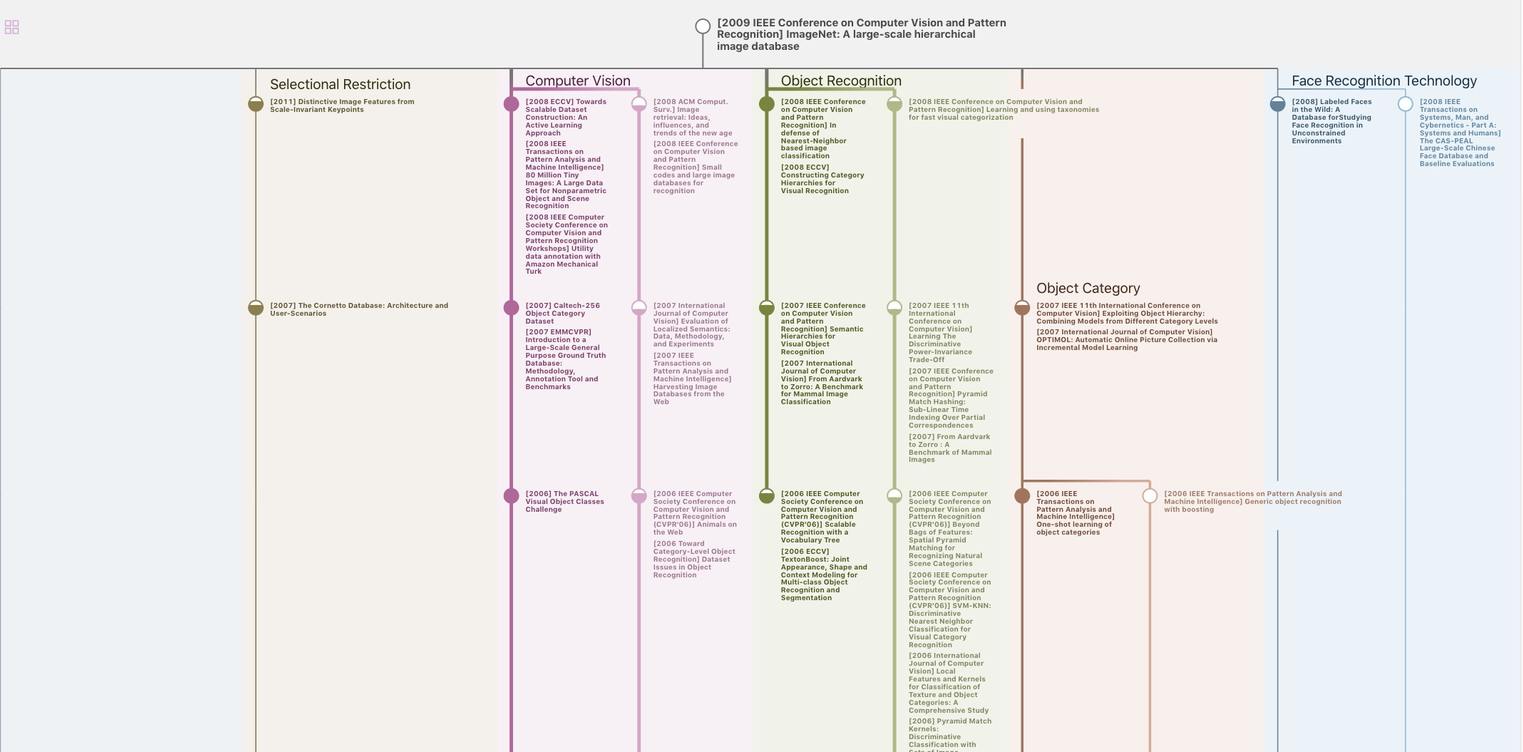
生成溯源树,研究论文发展脉络
Chat Paper
正在生成论文摘要