TransFlow: Transformer As Flow Learner.
CVPR 2023(2023)
摘要
Optical flow is an indispensable building block for various important computer vision tasks, including motion estimation, object tracking, and disparity measurement. In this work, we propose TransFlow, a pure transformer architecture for optical flow estimation. Compared to dominant CNN-based methods, TransFlow demonstrates three advantages. First, it provides more accurate correlation and trustworthy matching in flow estimation by utilizing spatial self-attention and crossattention mechanisms between adjacent frames to effectively capture global dependencies; Second, it recovers more compromised information (e.g., occlusion and motion blur) in flow estimation through long-range temporal association in dynamic scenes; Third, it enables a concise self-learning paradigm and effectively eliminate the complex and laborious multi-stage pre-training procedures. We achieve the state-of-the-art results on the Sintel, KITTI-15, as well as several downstream tasks, including video object detection, interpolation and stabilization. For its efficacy, we hope TransFlow could serve as a flexible baseline for optical flow estimation.
更多查看译文
关键词
Video: Low-level analysis,motion,tracking
AI 理解论文
溯源树
样例
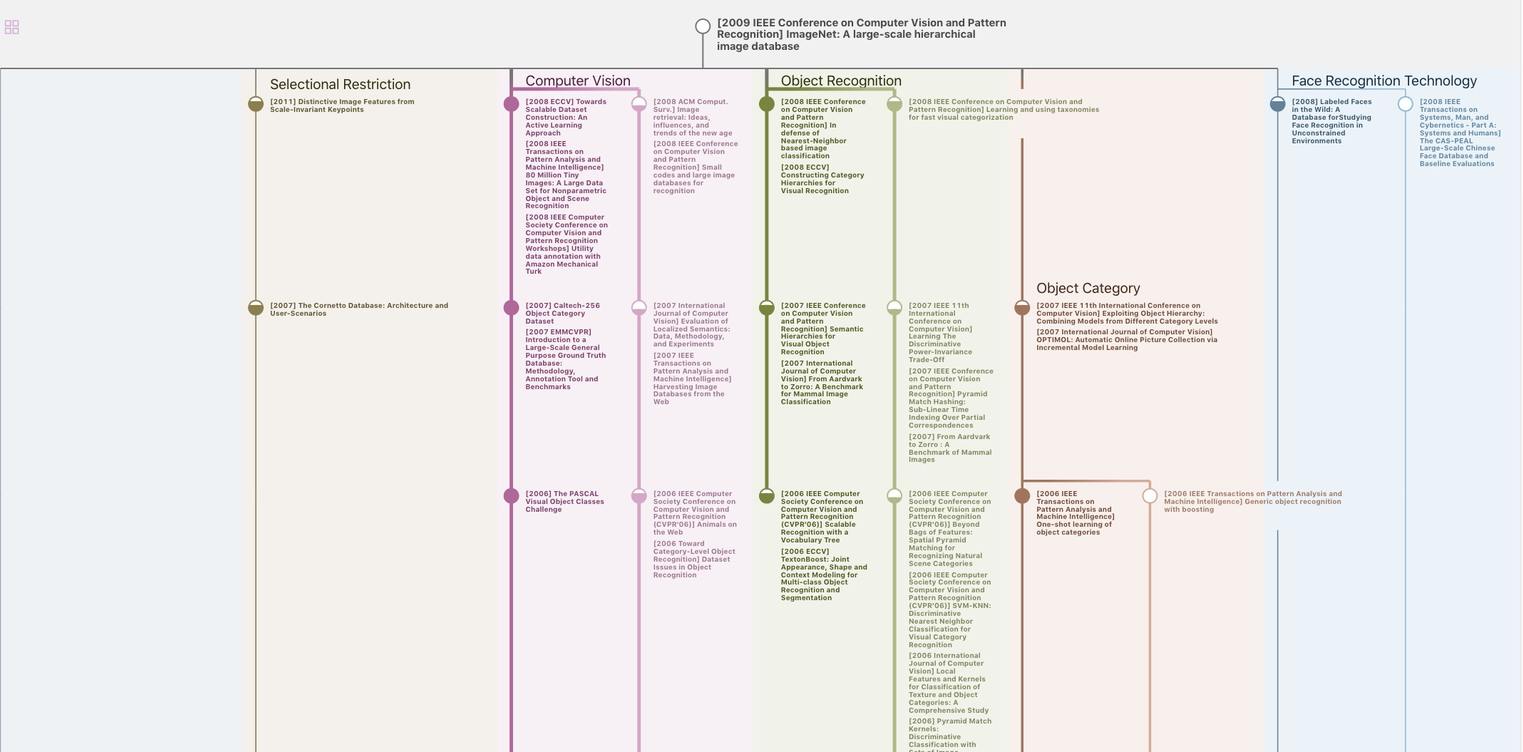
生成溯源树,研究论文发展脉络
Chat Paper
正在生成论文摘要