Competitive performance and superior noise robustness of a non-negative deep convolutional spiking network
bioRxiv (Cold Spring Harbor Laboratory)(2023)
摘要
Networks of spiking neurons promise to combine energy efficiency with high performance. However, spiking models that match the performance of current state-of-the-art networks while requiring moderate computational resources are still lacking. Here we present an alternative framework to deep convolutional networks (CNNs), the "Spike by Spike" network (SbS), together with an efficient backpropagation algorithm. SbS implements networks based on non-negative matrix factorisation (NNMF), but uses discrete events as signals instead of real values. On clean data, the performance of CNNs is matched by both NNMF-based networks and SbS. SbS are found to be most robust when the data is corrupted by noise, specially when this noise was not seen before.
更多查看译文
关键词
superior noise robustness,non-negative
AI 理解论文
溯源树
样例
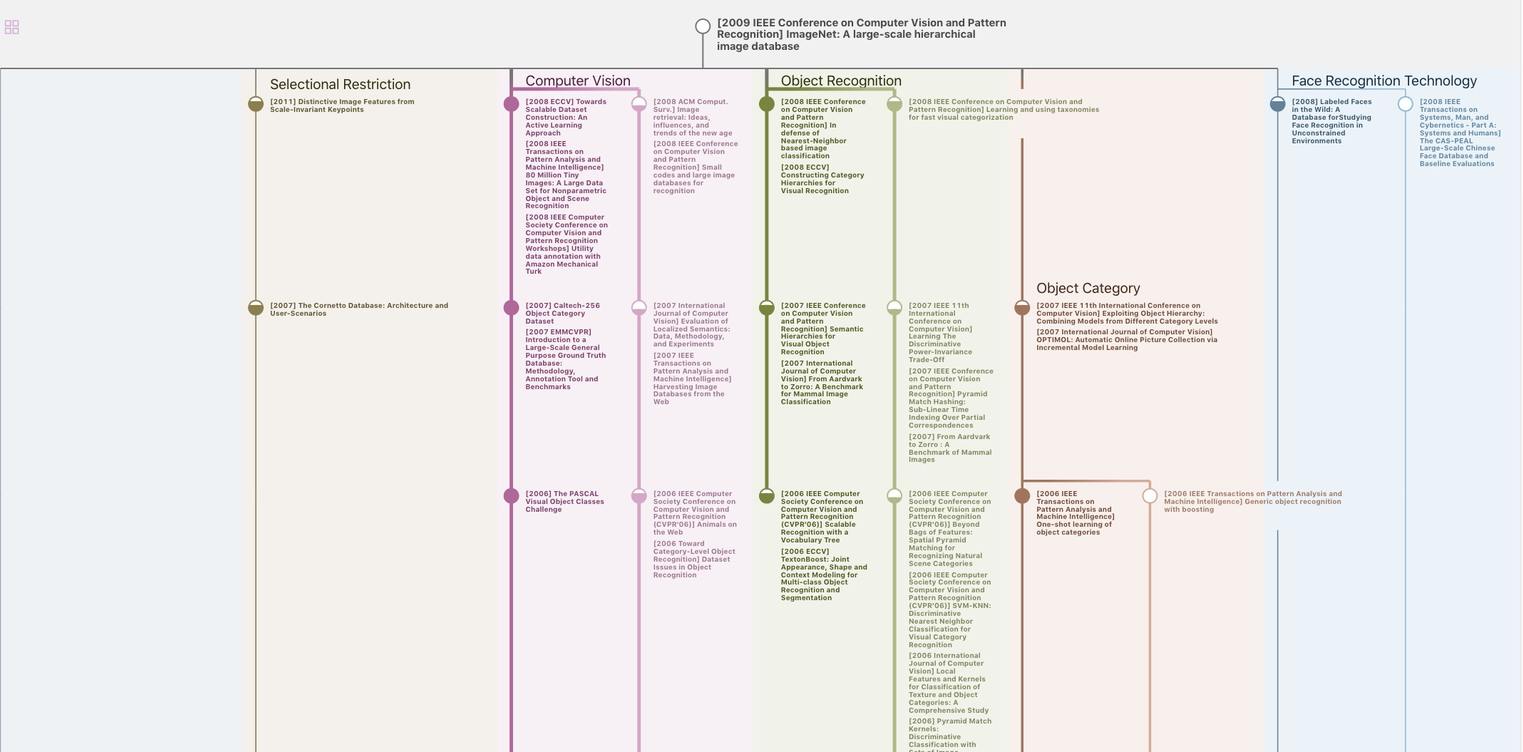
生成溯源树,研究论文发展脉络
Chat Paper
正在生成论文摘要