An accuracy study of the YouDiagnose disease predictive model based on a double-validated real-world dataset from the UK
medRxiv (Cold Spring Harbor Laboratory)(2023)
摘要
Through the utilisation of algorithms and data-driven analytics, predictive technology can be leveraged to provide clinicians with invaluable insight into their patients conditions, allowing for more accurate, informed, and timely decision-making in the fast-paced clinical environment. This study aims to provide a preliminary proof of concept with a double-validated real-world dataset from the UK. The YouDiagnose predictive model, developed using retrospective data from over 41,257 patient data, was assessed by testing it with a double-validated real-world dataset from the UK and the machine predictions have been compared here with the final diagnosis of the diseases as the gold standard. Out of the total of 433 cases, 60 cases had a mismatch in their prediction, all of them being cancer overdiagnosis, resulting in a lower specificity rate of 84.3%. The combined prediction accuracy at the first prediction level was 86% (n=373) while prediction 1-3 combined was successful in predicting diseases in 93% of the cases when evaluated against the gold standard. The model accurately predicted all 52 cases of cancer, indicating a 100% sensitivity rate. The study shows that the tool can be used in the frontline to accurately screen patients with a high level of confidence in the inclusion of cancer patients. The high sensitivity means that there is little chance of missing any cancer cases.
### Competing Interest Statement
The authors have declared no competing interest.
### Funding Statement
This study was funded by YouDiagnose Limited as it is part of its market research activities
### Author Declarations
I confirm all relevant ethical guidelines have been followed, and any necessary IRB and/or ethics committee approvals have been obtained.
Yes
The details of the IRB/oversight body that provided approval or exemption for the research described are given below:
: At the outset, the research methodology was approved by YouDiagnose Ethical Approval Committee. This research work was commenced after this approval and this study did not involve any non-anonymised human data, tissues, samples, or materials. Consent to participate was obtained from each participant at the beginning of the study. Using the NHS Health Research Authority and United Kingdom Medical Research Council decision-making tool, it was determined that this study would produce generalisable or transferable findings. Therefore, informed consent was obtained from each participant before the structured interview. It was also determined that the study would anonymize the participants to mitigate any risk. All the methodologies were in line with the core practices of the Committee on Publication Ethics (COPE) and the Declaration of Helsinki.
I confirm that all necessary patient/participant consent has been obtained and the appropriate institutional forms have been archived, and that any patient/participant/sample identifiers included were not known to anyone (e.g., hospital staff, patients or participants themselves) outside the research group so cannot be used to identify individuals.
Yes
I understand that all clinical trials and any other prospective interventional studies must be registered with an ICMJE-approved registry, such as ClinicalTrials.gov. I confirm that any such study reported in the manuscript has been registered and the trial registration ID is provided (note: if posting a prospective study registered retrospectively, please provide a statement in the trial ID field explaining why the study was not registered in advance).
Yes
I have followed all appropriate research reporting guidelines, such as any relevant EQUATOR Network research reporting checklist(s) and other pertinent material, if applicable.
Yes
https://predictive-pilot.eniston.com/about-the-study/can-i-see-the-database-which-was-used-to-build-the-model
更多查看译文
关键词
youdiagnose disease,predictive model,accuracy study,double-validated,real-world
AI 理解论文
溯源树
样例
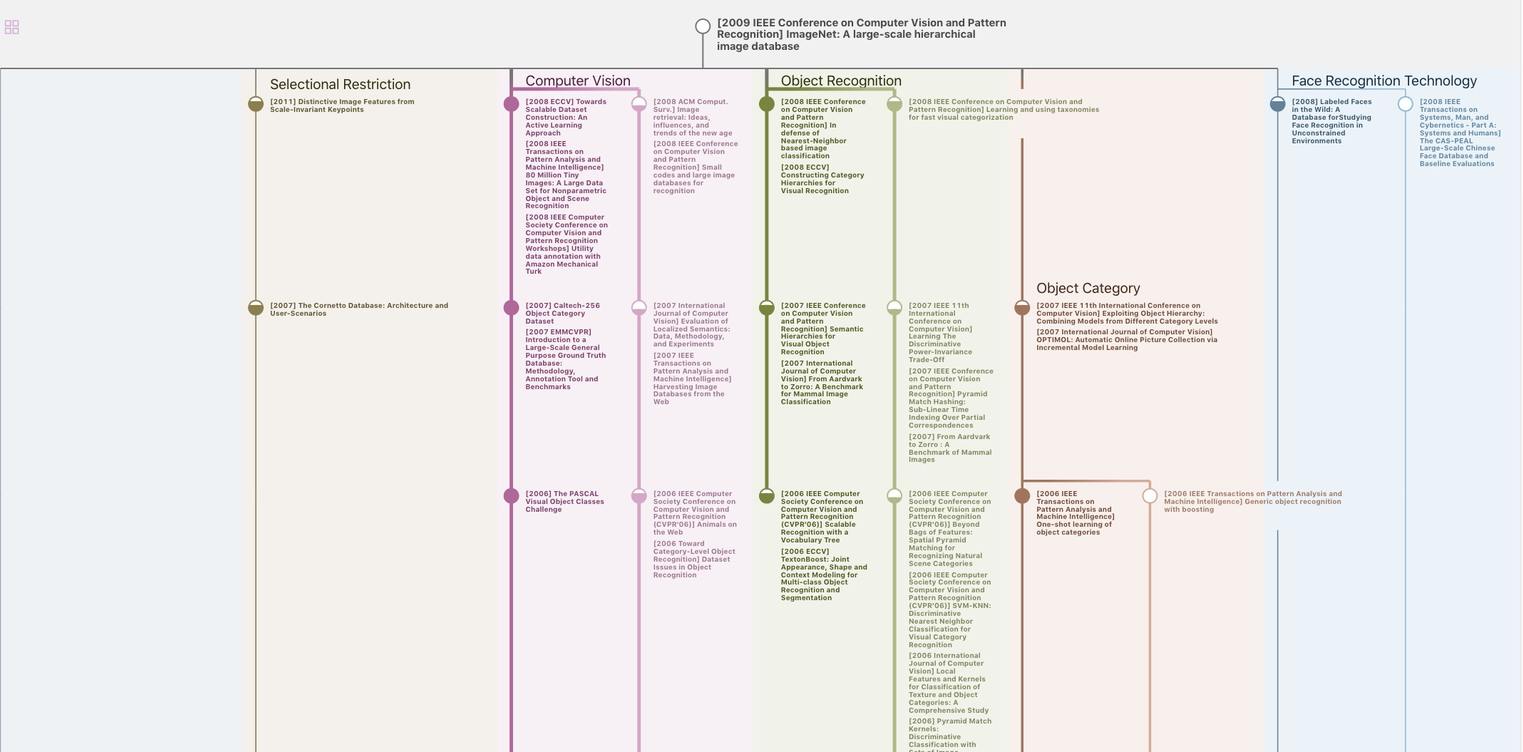
生成溯源树,研究论文发展脉络
Chat Paper
正在生成论文摘要